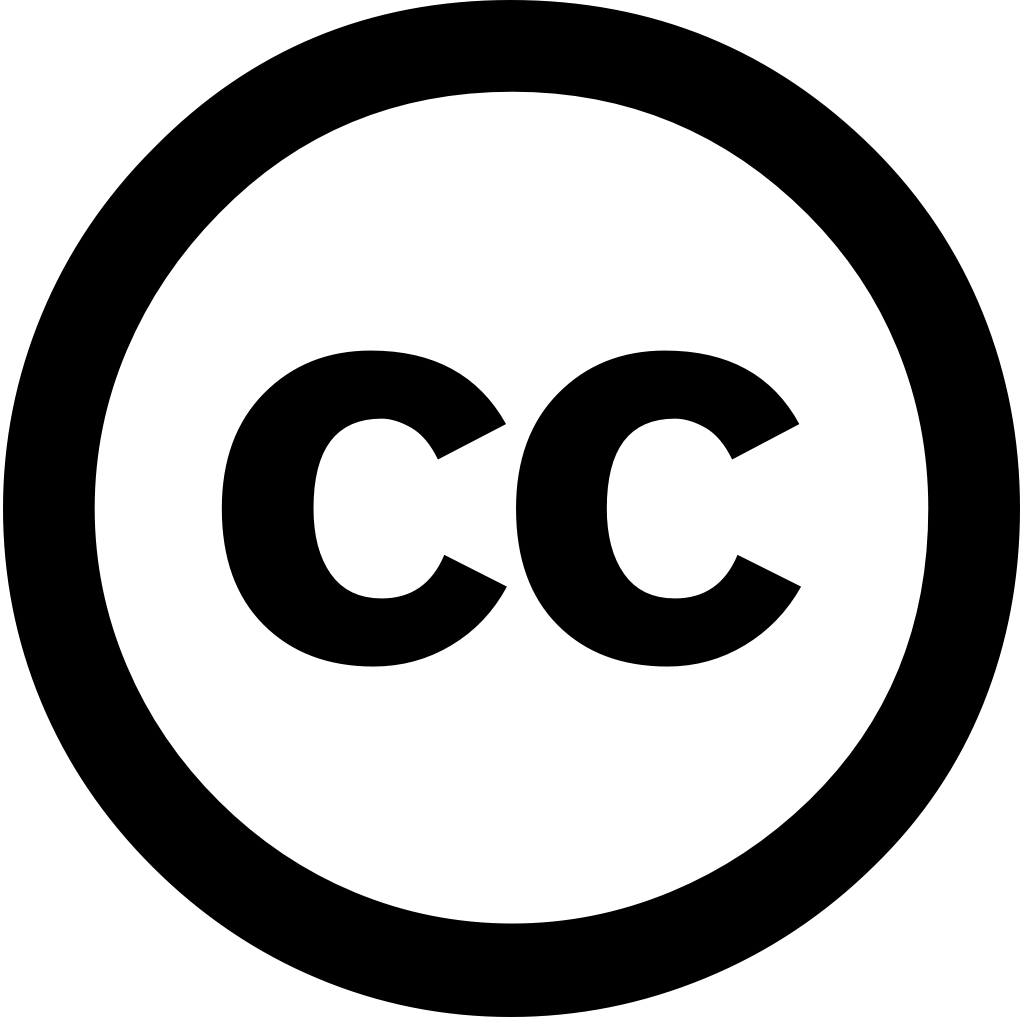
Applied Sciences, Journal Year: 2025, Volume and Issue: 15(6), P. 3320 - 3320
Published: March 18, 2025
As a result of the increase in number smart buildings and advances technology, energy consumption has become increasingly important. The estimation is critical for efficiency. Accurate photovoltaic (PV) solar power plant efficiency crucial optimizing performance renewable applications. In this study, advanced machine learning regression models such as XGBoost, CatBoost, LightGBM, AdaBoost Histogram-Based Gradient Boosting are used to predict PV based on ten internal features (Open Circuit Voltage (Voc), Short Current (Isc), Maximum Power (Pmpp), Solar Irradiation Spread (SIS), (Vmpp), (Impp), Fill Factor (FF), Parallel Resistance (Rp), Series (Rs), Module Temperature (Tm)) module measurements from Utrecht University Photovoltaic Outdoor Test Facility. result, CatBoost outperformed others, achieving lowest prediction error MSE 0.002 highest R2 value 0.90. To interpret model’s predictions, we applied Explainable Artificial Intelligence techniques, particular SHAP LIME, which identify key affecting model transparency. integration these methods provides valuable insights design optimization.
Language: Английский