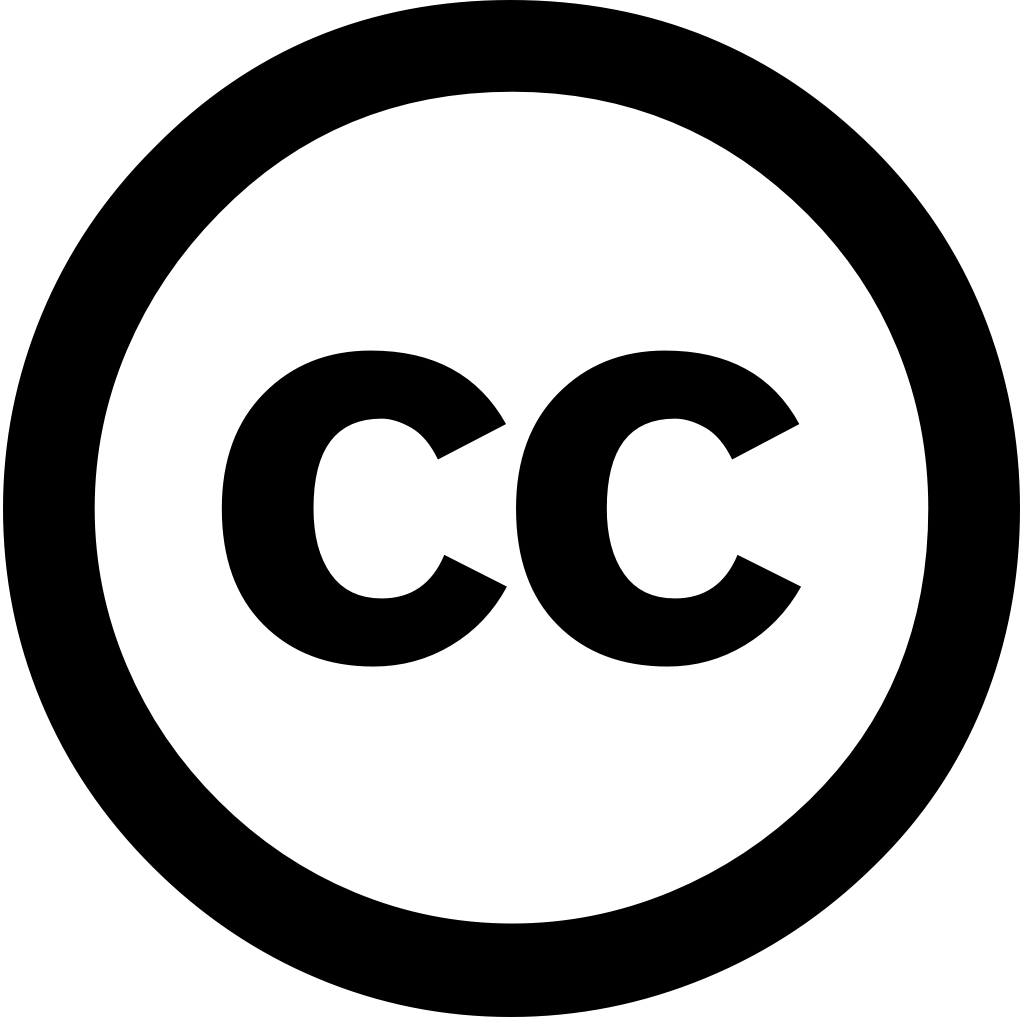
Journal of Engineering and Applied Science, Journal Year: 2025, Volume and Issue: 72(1)
Published: Jan. 17, 2025
Abstract Skin cancer presents in various forms, including squamous cell carcinoma (SCC), basal (BCC), and melanoma. Established risk factors include ultraviolet (UV) radiation exposure from solar or artificial sources, lighter skin pigmentation, a history of sunburns, family the disease. Early detection prompt intervention are crucial for achieving favorable prognosis. Traditionally, treatment modalities surgery, therapy, chemotherapy. Recent advancements immunotherapy have revolutionized diagnosis, but manual identification remains time-consuming. Artificial intelligence (AI) has shown potential classification, leading to automated screening methods. To support dermatologists, we improved model classifying images. This is able recognize seven different kinds lesions. On ISIC dataset, an analysis been done. study offers novel approach early diagnosis based on image processing. Our leverages high accuracy specific convolutional neural network architecture, utilizing transfer learning with pre-trained data further enhance performance. findings demonstrate that employed ResNet-50 achieves remarkable 97%, while ResNet50 without augmentation gives 81.57% F1-score 75.75%.
Language: Английский