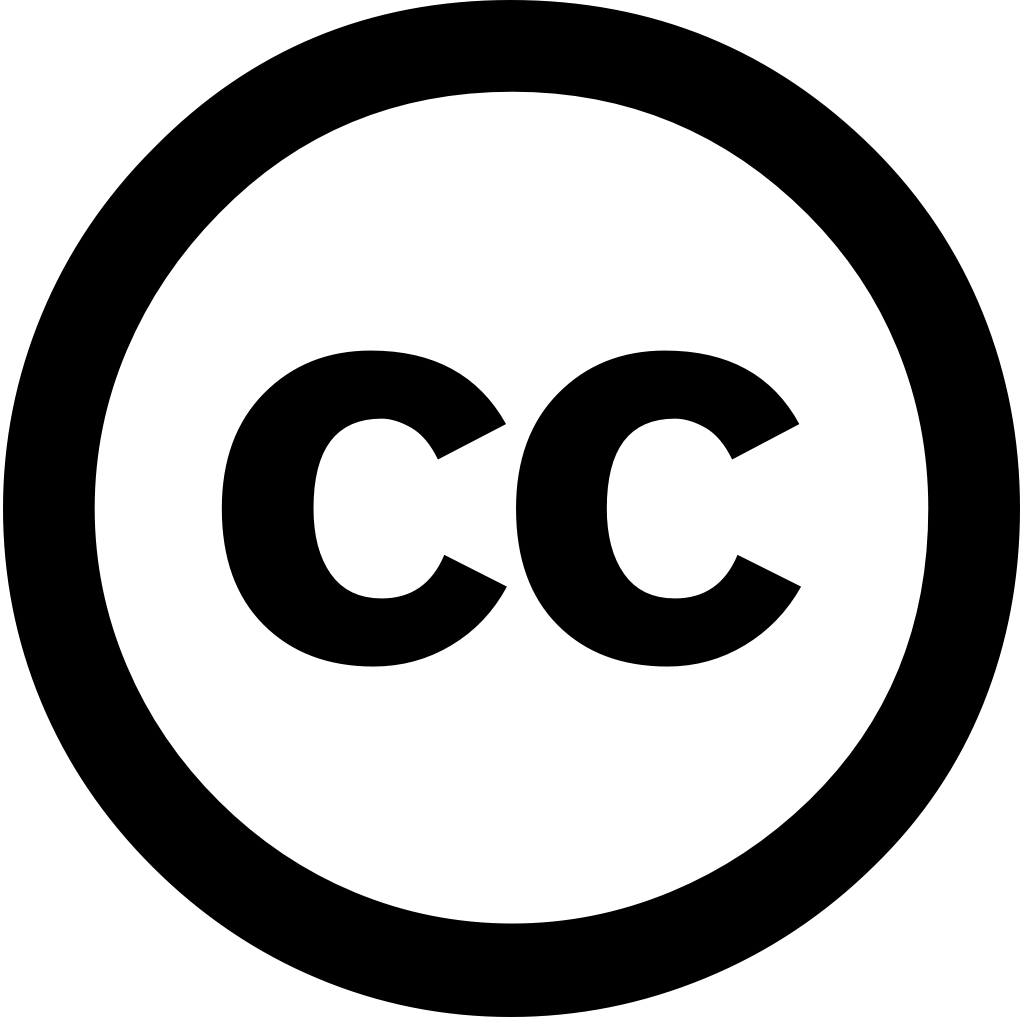
Emergency Management Science and Technology, Journal Year: 2020, Volume and Issue: 0(0), P. 1 - 12
Published: Jan. 1, 2020
Language: Английский
Emergency Management Science and Technology, Journal Year: 2020, Volume and Issue: 0(0), P. 1 - 12
Published: Jan. 1, 2020
Language: Английский
Forest Ecology and Management, Journal Year: 2024, Volume and Issue: 555, P. 121729 - 121729
Published: Jan. 31, 2024
Accurately assessing forest fire susceptibility (FFS) in the Similipal Tiger Reserve (STR) is essential for biodiversity conservation, climate change mitigation, and community safety. Most existing studies have primarily focused on climatic topographical factors, while this research expands scope by employing a synergistic approach that integrates geographical information systems (GIS), remote sensing (RS), machine learning (ML) methodologies identifying fire-prone areas STR their vulnerability to change. To achieve this, study employed comprehensive dataset of forty-four influencing including topographic, climate-hydrologic, health, vegetation indices, radar features, anthropogenic interference, into ten ML models: neural net (nnet), AdaBag, Extreme Gradient Boosting (XGBTree), Machine (GBM), Random Forest (RF), its hybrid variants with differential evolution algorithm (RF-DEA), Gravitational Based Search (RF-GBS), Grey Wolf Optimization (RF-GWO), Particle Swarm (RF-PSO), genetic (RF-GA). The revealed high FFS both northern southern portions area, nnet RF-PSO models demonstrating percentages 12.44% 12.89%, respectively. Conversely, very low zones consistently displayed scores approximately 23.41% 18.57% models. robust mapping methodology was validated impressive AUROC (>0.88) kappa coefficient (>0.62) across all validation metrics. Future (ssp245 ssp585, 2022–2100) indicated along edges STR, central zone categorized from susceptibility. Boruta analysis identified actual evapotranspiration (AET) relative humidity as key factors ignition. SHAP evaluation reinforced influence these FFS, also highlighting significant role distance road, settlement, dNBR, slope, prediction accuracy. These results emphasize critical importance proposed provide invaluable insights firefighting teams, management, planning, qualification strategies address future sustainability.
Language: Английский
Citations
27Environmental Technology & Innovation, Journal Year: 2024, Volume and Issue: 35, P. 103655 - 103655
Published: May 5, 2024
Forest fires pose a significant threat to ecosystems and socio-economic activities, necessitating the development of accurate predictive models for effective management mitigation. In this study, we present novel machine learning approach combined with Explainable Artificial Intelligence (XAI) techniques predict forest fire susceptibility in Nainital district. Our innovative methodology integrates several robust — AdaBoost, Gradient Boosting Machine (GBM), XGBoost Random Deep Neural Network (DNN) as meta-model stacking framework. This not only utilises individual strengths these models, but also improves overall prediction performance reliability. By using XAI techniques, particular SHAP (SHapley Additive exPlanations) LIME (Local Interpretable Model-agnostic Explanations), improve interpretability provide insights into decision-making processes. results show effectiveness ensemble model categorising different zones: very low, moderate, high high. particular, identified extensive areas susceptibility, precision, recall F1 values underpinning their effectiveness. These achieved ROC AUC above 0.90, performing exceptionally well an 0.94. The are remarkably inclusion confidence intervals most important metrics all emphasises robustness reliability supports practical use management. Through summary plots, analyze global variable importance, revealing annual rainfall Evapotranspiration (ET) key factors influencing susceptibility. Local analysis consistently highlights importance rainfall, ET, distance from roads across models. study fills research gap by providing comprehensive interpretable modelling that our ability effectively manage risk is consistent environmental protection sustainable goals.
Language: Английский
Citations
20Environmental Science and Pollution Research, Journal Year: 2025, Volume and Issue: unknown
Published: Jan. 30, 2025
Language: Английский
Citations
2Remote Sensing, Journal Year: 2024, Volume and Issue: 16(15), P. 2842 - 2842
Published: Aug. 2, 2024
Wildfire susceptibility maps play a crucial role in preemptively identifying regions at risk of future fires and informing decisions related to wildfire management, thereby aiding mitigating the risks potential damage posed by wildfires. This study employs eXplainable Artificial Intelligence (XAI) techniques, particularly SHapley Additive exPlanations (SHAP), map Izmir Province, Türkiye. Incorporating fifteen conditioning factors spanning topography, climate, anthropogenic influences, vegetation characteristics, machine learning (ML) models (Random Forest, XGBoost, LightGBM) were used predict wildfire-prone areas using freely available active fire pixel data (MODIS Active Fire Collection 6 MCD14ML product). The evaluation trained ML showed that Random Forest (RF) model outperformed XGBoost LightGBM, achieving highest test accuracy (95.6%). All classifiers demonstrated strong predictive performance, but RF excelled sensitivity, specificity, precision, F-1 score, making it preferred for generating conducting SHAP analysis. Unlike prevailing approaches focusing solely on global feature importance, this fills critical gap employing summary dependence plots comprehensively assess each factor’s contribution, enhancing explainability reliability results. analysis reveals clear associations between such as wind speed, temperature, NDVI, slope, distance villages with increased susceptibility, while rainfall streams exhibit nuanced effects. spatial distribution classes highlights areas, flat coastal near settlements agricultural lands, emphasizing need enhanced awareness preventive measures. These insights inform targeted management strategies, highlighting importance tailored interventions like firebreaks management. However, challenges remain, including ensuring selected factors’ adequacy across diverse regions, addressing biases from resampling spatially varied data, refining broader applicability.
Language: Английский
Citations
10Forests, Journal Year: 2025, Volume and Issue: 16(2), P. 273 - 273
Published: Feb. 5, 2025
Forest fires are the result of poor land management and climate change. Depending on type affected eco-system, they can cause significant biodiversity losses. This study was conducted in Amazonas department Peru. Binary data obtained from MODIS satellite occurrence between 2010 2022 were used to build risk models. To avoid multicollinearity, 12 variables that trigger selected (Pearson ≤ 0.90) grouped into four factors: (i) topographic, (ii) social, (iii) climatic, (iv) biological. The program Rstudio three types machine learning applied: MaxENT, Support Vector Machine (SVM), Random (RF). results show RF model has highest accuracy (AUC = 0.91), followed by MaxENT 0.87) SVM 0.84). In fire map elaborated with model, 38.8% region possesses a very low occurrence, 21.8% represents high-risk level zones. research will allow decision-makers improve forest Amazon prioritize prospective strategies such as installation water reservoirs areas zone. addition, it support awareness-raising actions among inhabitants at greatest so be prepared mitigate control generate solutions event occurring under different scenarios.
Language: Английский
Citations
1Geoenergy Science and Engineering, Journal Year: 2023, Volume and Issue: 233, P. 212518 - 212518
Published: Nov. 25, 2023
Language: Английский
Citations
17IEEE Access, Journal Year: 2024, Volume and Issue: 12, P. 55389 - 55403
Published: Jan. 1, 2024
Forest fires constitute a widespread and impactful natural disaster, annually ravaging millions of hectares forests posing severe threat to human life property. Accurate quantitative prediction forest fire spread is essential for devising swift risk management strategies implementing effective firefighting approaches. In response this imperative, study introduces Fire Spread Behavior Prediction (FFSBP) model, encompassing two integral components: the Process (FFSPP) model Results (FFSRP) model. The FFSPP involves direction speed spread, achieved through fusion Cellular Automata Wang Zhengfei On other hand, FFSRP focuses on forecasting extent burned area, leveraging machine learning methods. To validate efficacy proposed models, real case undertaken using "3.29 Fire" incident in China. validated against case, while assessed dataset obtained from Montesinho National Park Portugal. validation process reveal that during development period Fire," predicts area 286.81 hm 2 , with an associated relative error 28.94%. This notably smaller than those observed Farsite Prometheus behavior simulation models. Additionally, demonstrates commendable predictive performance, particularly context small medium-sized scenarios. These findings underscore potential FFSBP as valuable tool enhancing accuracy facilitating more robust mitigation strategies.
Language: Английский
Citations
6Fire, Journal Year: 2024, Volume and Issue: 7(2), P. 58 - 58
Published: Feb. 17, 2024
Vegetation classification, biomass assessment, and wildfire dynamics are interconnected wildfire-ecosystem components. The Chongli District, located in Zhangjiakou City, was the venue for skiing at 2022 Winter Olympics. Its high mountains dense forests create a unique environment. establishment of alpine ski resorts highlighted importance comprehensive forest surveys. Understanding vegetation types their is critical to assessing distribution local resources predicting likelihood fires. This study used satellite multispectral data from Sentinel-2B classify surface District through K-means clustering. By combining this classification with inversion model, total survey area can be calculated. equation established based on remote sensing terrain information have strong correlation (shrub R2 = 0.811, broadleaf 0.356, coniferous 0.223). These coefficients key indicators our understanding relationship between actual biomass, reflecting performance model. Taking shrubland as an example, coefficient 0.811 shows model’s ability accurately predict type vegetation. In addition, multiple linear regression, optimal shrub, broadleaf, models were obtained, overall accuracy reaching 93.58%, 89.56%, 97.53%, respectively, meeting strict requirements accuracy. successfully conducted using data. research results important implications prevention control
Language: Английский
Citations
4Expert Systems with Applications, Journal Year: 2024, Volume and Issue: 258, P. 124963 - 124963
Published: Aug. 5, 2024
Language: Английский
Citations
4Sustainability, Journal Year: 2025, Volume and Issue: 17(5), P. 1971 - 1971
Published: Feb. 25, 2025
Forest fires pose significant environmental and economic risks, particularly in fire-prone regions like the Mediterranean coast of Türkiye. This study presents a comprehensive Fire Danger Assessment System (FoFiDAS), by integrating Geographic Information Systems (GIS), literature-based model, Analytical Hierarchy Process (AHP), machine learning (ML) to improve forest fire danger classification. Both models integrate 13 key parameters identified through literature. A comparison these revealed 53% overlap classifications. While AHP based on expert-weighted assessment, provided more structured localized classification, model relied broader scientific data but lacked adaptability. Pearson correlation analysis demonstrated strong between classifications historical occurrences, with scores 0.927 (AHP) 0.939 (literature-based). Further ROC confirmed predictive performance both models, yielding AUC values 0.91 0.9121 for respectively. Five ML algorithms were used validate classification performances, Artificial Neural Network (ANN) achieving highest accuracy (86.5%). The ANN algorithm exceeded 0.93 each class, F1-Score was above 0.85. FoFiDAS offers reliable tool supporting early intervention decision making.
Language: Английский
Citations
0