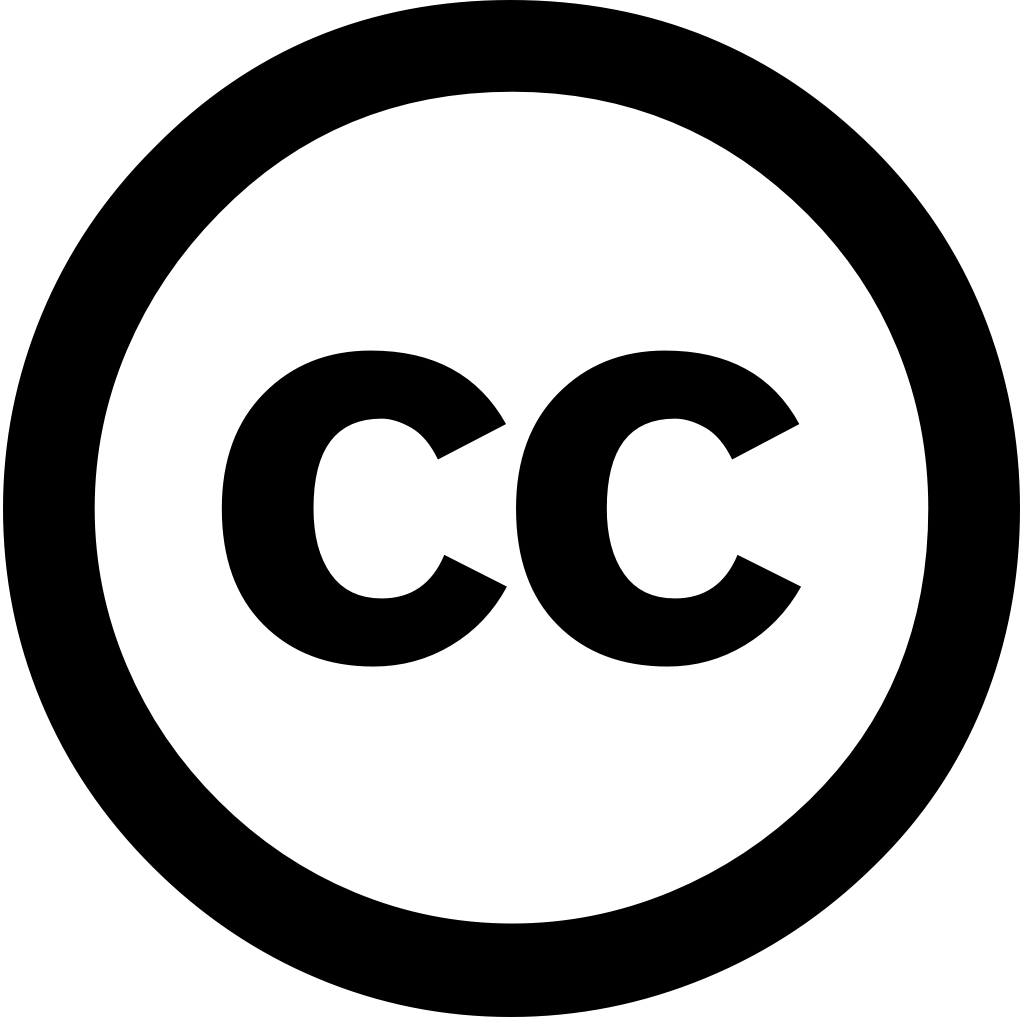
Emergency Management Science and Technology, Journal Year: 2020, Volume and Issue: 0(0), P. 1 - 12
Published: Jan. 1, 2020
Language: Английский
Emergency Management Science and Technology, Journal Year: 2020, Volume and Issue: 0(0), P. 1 - 12
Published: Jan. 1, 2020
Language: Английский
Forests, Journal Year: 2025, Volume and Issue: 16(4), P. 704 - 704
Published: April 19, 2025
In recent years, the increasingly significant impacts of climate change and human activities on environment have led to more frequent occurrences extreme events such as forest fires. The recurrent wildfires pose severe threats ecological environments life safety. Consequently, fire prediction has become a current research hotspot, where accurate forecasting technologies are crucial for reducing economic losses, improving management efficiency, ensuring personnel safety property security. To enhance comprehensive understanding wildfire research, this paper systematically reviews studies since 2015, focusing two key aspects: datasets with related tools algorithms. We categorized literature into three categories: statistical analysis physical models, traditional machine learning methods, deep approaches. Additionally, review summarizes data types open-source used in selected literature. further outlines challenges future directions, including exploring risk multimodal learning, investigating self-supervised model interpretability developing explainable integrating physics-informed models constructing digital twin technology real-time simulation scenario analysis. This study aims provide valuable support natural resource enhanced environmental protection through application remote sensing artificial intelligence
Language: Английский
Citations
0Research Square (Research Square), Journal Year: 2025, Volume and Issue: unknown
Published: April 24, 2025
Language: Английский
Citations
0Future Internet, Journal Year: 2024, Volume and Issue: 16(11), P. 396 - 396
Published: Oct. 28, 2024
For thousands of years forest fires played the role a regulator in ecosystem. Forest contributed to ecological balance by destroying old and diseased plant material; but modern era are major problem that tests endurance not only government agencies around world, also have an effect on climate change. become more intense, destructive, deadly; these known as megafires. They can cause economic problems, especially summer months (dry season). However, humanity has developed tool predict fire events, detect them time, their duration. This is artificial intelligence, specifically, machine learning, which one part AI. Consequently, this paper briefly mentions several methods learning used predicting early detection, submitting overall review current models. Our main objective venture into new field: duration ongoing fires. contribution offers way manage fires, using accessible open data, available from Hellenic Fire Service. In particular, we imported over 72,000 data 10-year period (2014–2023) techniques. The experimental validation results than encouraging, with Random achieving lowest value for error range (8–13%), meaning it was 87–92% accurate prediction Finally, some future directions extend research presented.
Language: Английский
Citations
0Emergency Management Science and Technology, Journal Year: 2020, Volume and Issue: 0(0), P. 1 - 12
Published: Jan. 1, 2020
Language: Английский
Citations
1