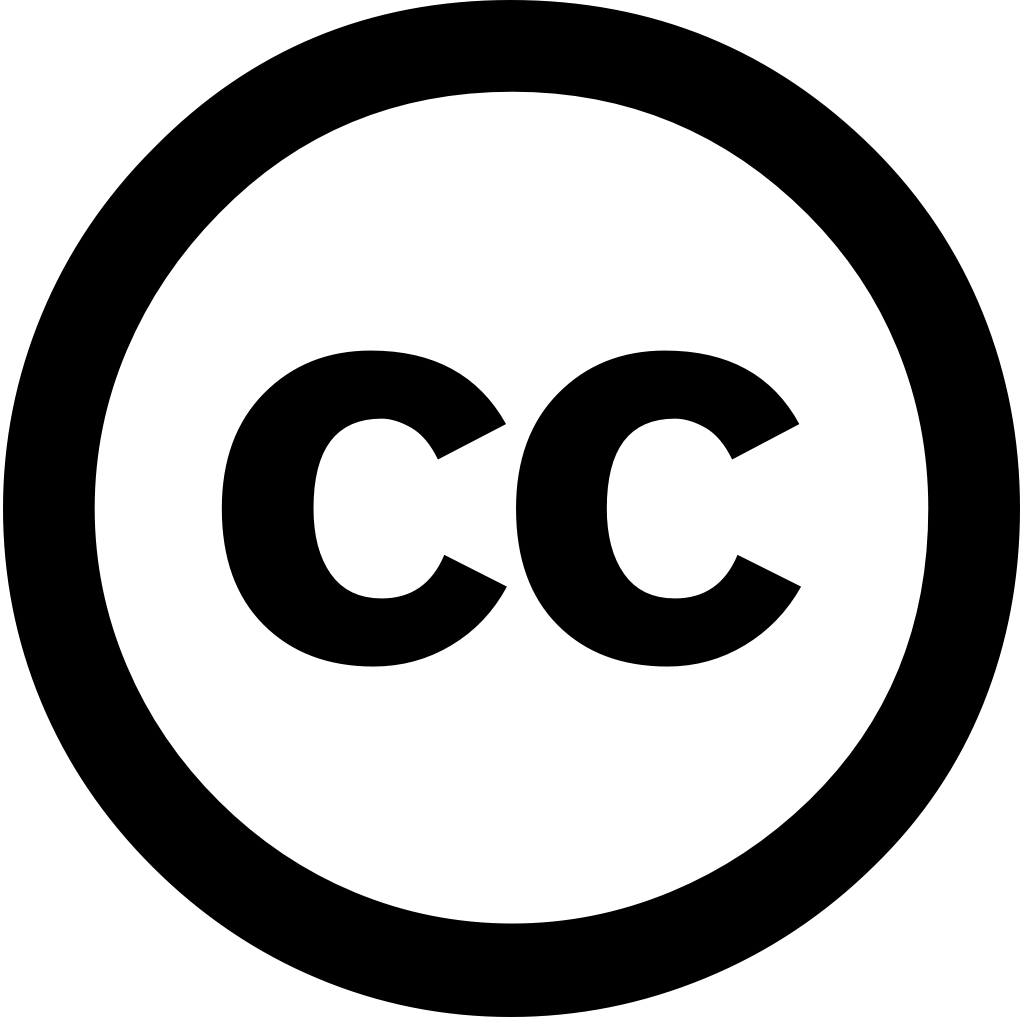
Information, Journal Year: 2025, Volume and Issue: 16(2), P. 71 - 71
Published: Jan. 21, 2025
In this study, anomalies in a fan system were classified using real measurement setup to simulate mechanical such as blade detachment or debris accumulation. Data collected under normal operating conditions and with an added unbalancing mass. Additionally, sensor introduced by manipulating accelerometer readings examining three types: spike, stuck, dropout. To classify the anomalies, four neural network models—variations Long Short-Term Memory (LSTM) Convolutional Neural Network (CNN) tested. These models incorporated Continuous Wavelet Transform (CWT) layer. A novel approach for implementing CWT layer both LSTM CNN architectures was proposed, along dual-branch input structure featuring two layers different mother wavelets. The configuration wavelets yielded better accuracy simpler network. Accuracy comparisons conducted 10 best-performing based on validation set predictions, revealing improved classification performance. study concluded summary of prediction test sets data, calculation average accuracy, demonstrating effectiveness proposed classifying systems.
Language: Английский