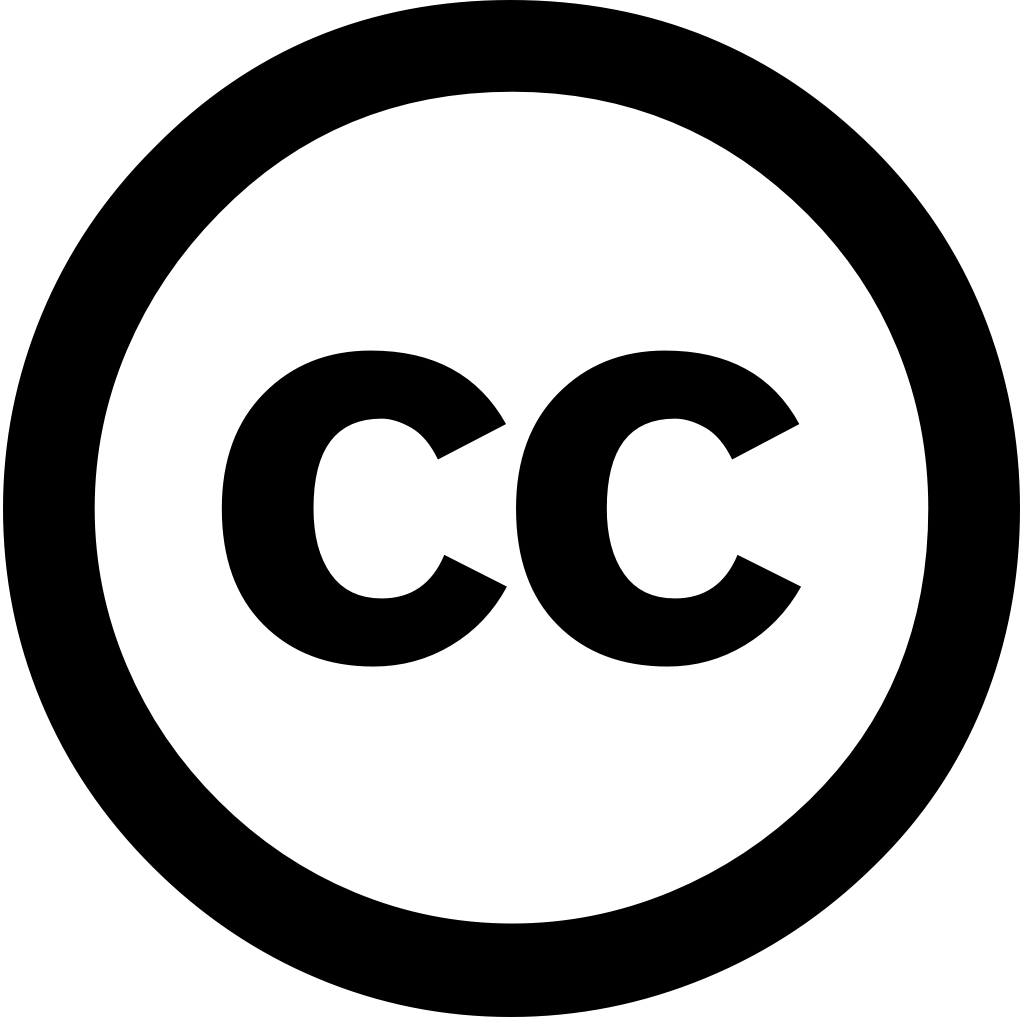
AI, Journal Year: 2025, Volume and Issue: 6(4), P. 76 - 76
Published: April 11, 2025
Breast cancer detection is a critical task in healthcare, requiring fast, accurate, and efficient diagnostic tools. However, the high computational demands latency of deep learning models medical imaging present significant challenges, especially resource-constrained environments. This paper addresses these challenges by presenting an FPGA hardware accelerator tailored for breast classification, leveraging Zynq XC7Z020 SoC. The system integrates FPGA-accelerated layers with ARM Cortex-A9 processor to optimize both performance resource efficiency. We developed modular IP cores, including Conv2D, Average Pooling, ReLU, using Vivado HLS maximize utilization. By adopting 8-bit fixed-point arithmetic, design achieves 15.8% reduction execution time compared traditional CPU-based implementations while maintaining classification accuracy. Additionally, our optimized approach significantly enhances energy efficiency, reducing power consumption from 3.8 W 1.4 63.15% reduction. improvement makes highly suitable real-time, power-sensitive applications, particularly embedded edge computing Furthermore, it underscores scalability efficiency FPGA-based AI solutions healthcare diagnostics, enabling faster more energy-efficient inference on devices.
Language: Английский