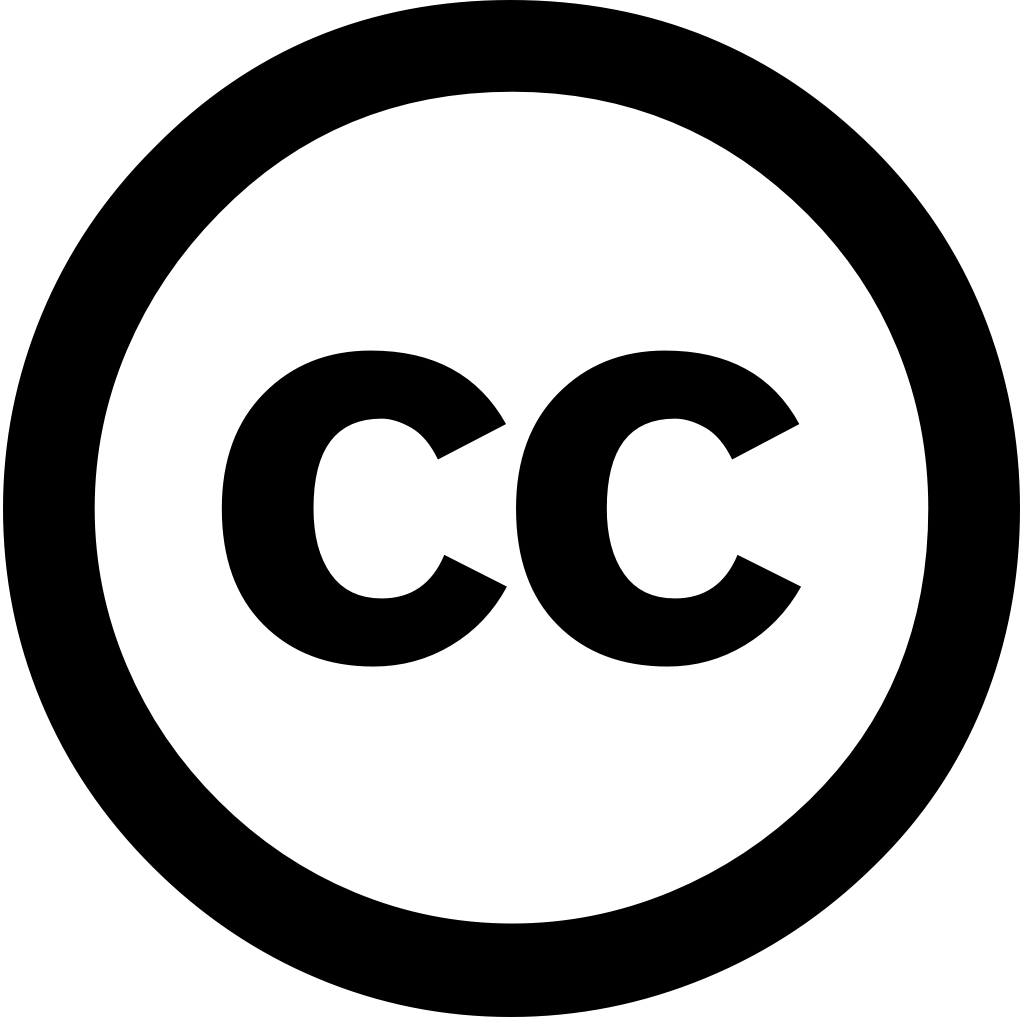
IET Image Processing, Journal Year: 2025, Volume and Issue: 19(1)
Published: Jan. 1, 2025
ABSTRACT VGG‐16 and Inception are widely used CNN architectures for image classification, but they face challenges in target categorization. This study introduces B4‐GraftingNet, a novel deep learning model that integrates VGG‐16's hierarchical feature extraction with Inception's diversified receptive field strategy. The is trained on the OCT‐CXR dataset evaluated NIH‐CXR to ensure robust generalization. Unlike conventional approaches, B4‐GraftingNet incorporates binary particle swarm optimization (BPSO) selection grad‐CAM interpretability. Additionally, performed, multiple machine classifiers (SVM, KNN, random forest, naïve Bayes) determine optimal representation. achieves 94.01% accuracy, 94.22% sensitivity, 93.36% specificity, 95.18% F1‐score maintains 87.34% accuracy despite not being it. These results highlight model's superior classification performance, adaptability, potential real‐world deployment both medical general tasks.
Language: Английский