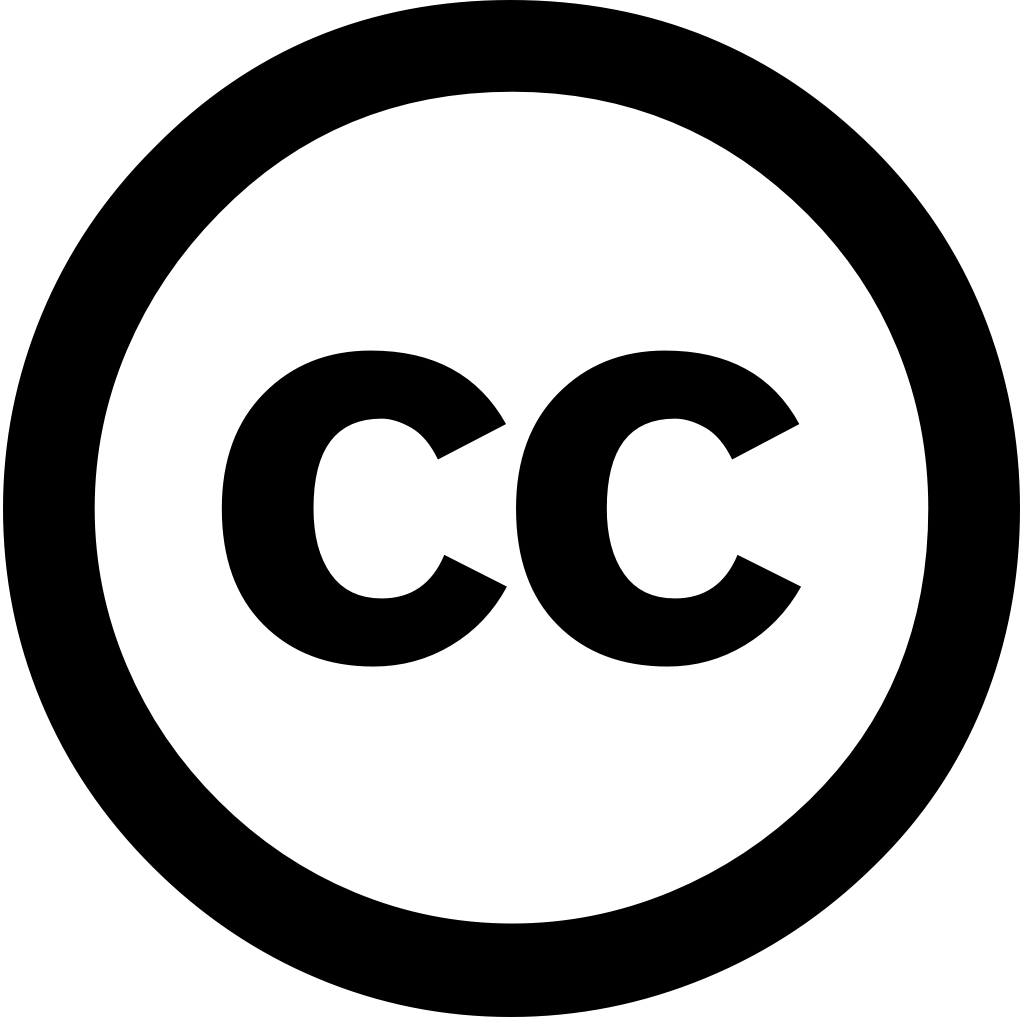
Ocean Engineering, Journal Year: 2024, Volume and Issue: 316, P. 119907 - 119907
Published: Dec. 2, 2024
Language: Английский
Ocean Engineering, Journal Year: 2024, Volume and Issue: 316, P. 119907 - 119907
Published: Dec. 2, 2024
Language: Английский
Journal of Marine Science and Engineering, Journal Year: 2025, Volume and Issue: 13(2), P. 251 - 251
Published: Jan. 29, 2025
Maritime behavior detection is vital for maritime surveillance and management, ensuring safe ship navigation, normal port operations, marine environmental protection, the prevention of illegal activities on water. Current methods detecting anomalous vessel behaviors primarily rely single time series data or feature point analysis, which struggle to capture relationships between behaviors, limiting anomaly identification accuracy. To address this challenge, we proposed a novel framework, called BPEF-TSD framework. It integrates pattern recognition algorithm, Smith–Waterman, text similarity measurement methods. Specifically, first introduced BPEF mining framework extract events from AIS data, then generated complete sequence chains through temporal combinations. Simultaneously, employed Smith–Waterman algorithm achieve local alignment test known sequences. Finally, evaluated overall based measure strategy, with vessels exceeding predefined threshold being flagged as anomalous. The results demonstrate that achieves over 90% accuracy in abnormal trajectories waters Xiamen Port, outperforming alternative such LSTM, iForest, HDBSCAN. This study contributes valuable insights enhancing safety advancing intelligent supervision while introducing research perspective big mining.
Language: Английский
Citations
0Ocean Engineering, Journal Year: 2024, Volume and Issue: 316, P. 119907 - 119907
Published: Dec. 2, 2024
Language: Английский
Citations
1