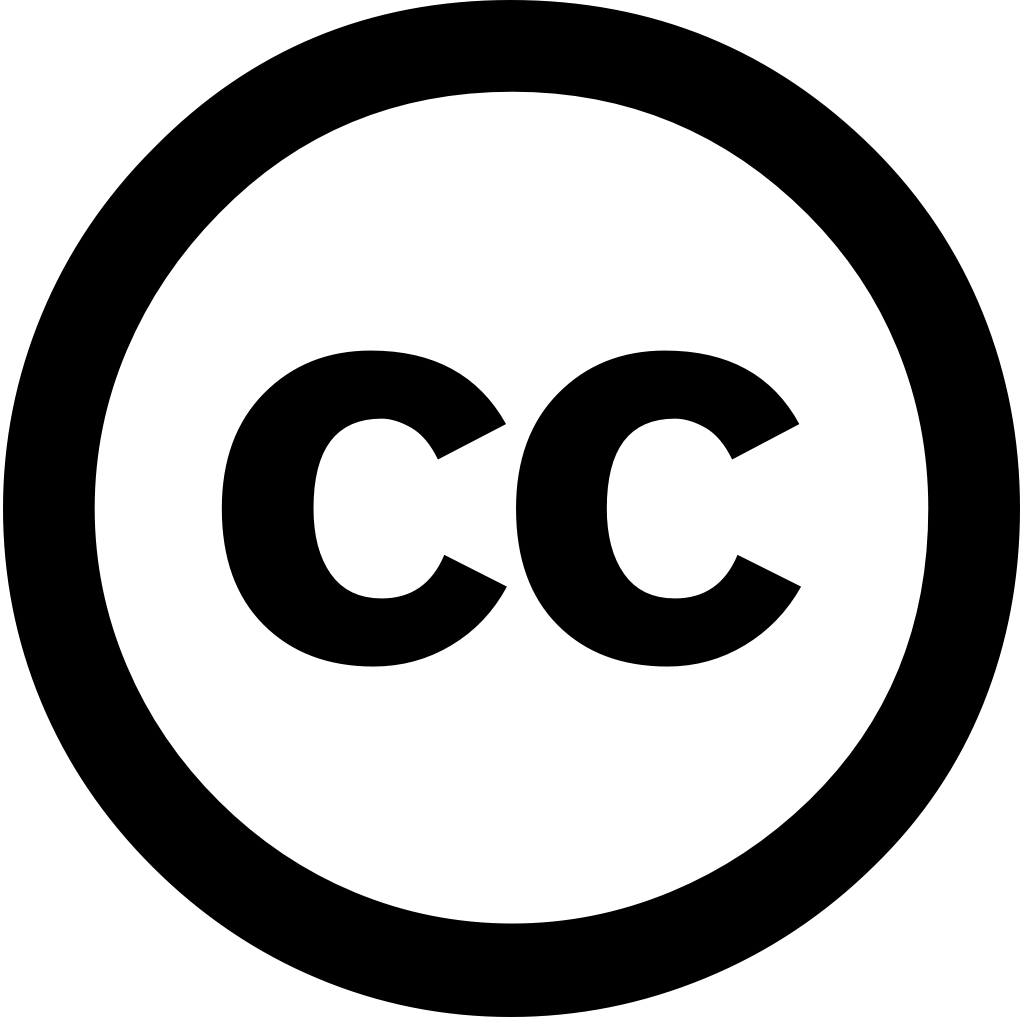
Journal of Marine Science and Engineering, Journal Year: 2025, Volume and Issue: 13(4), P. 783 - 783
Published: April 15, 2025
The condition monitoring of mooring equipment is an important engineering reliability issue during the operation a floating production storage and offloading unit (FPSO). chain jack (CJ) key for powering in spread system. Under complex dynamic marine operating conditions, different severity faults CJ hydraulic system display distinct time-scale characteristics. Hence, this paper proposes real-time fault diagnosis method based on multi-scale feature fusion. Firstly, model incorporates convolutional neural network (CNN) layer to extract localized spatial features from multivariate time-series data, effectively identifying patterns over associated short intervals. Subsequently, bidirectional long short-term memory (BiLSTM) introduced construct temporal comprehensively capture evolution severity. Finally, global attention mechanism (GAM) emphasizes persistent behaviors across time scales, dynamically prioritizing relevant improve diagnostic accuracy interpretability. study results indicate that proposed model’s improves by 7.36% CNN-GAM 11 failure modes, up 99.34%. This contributes safe FPSO guiding operations under load conditions.
Language: Английский