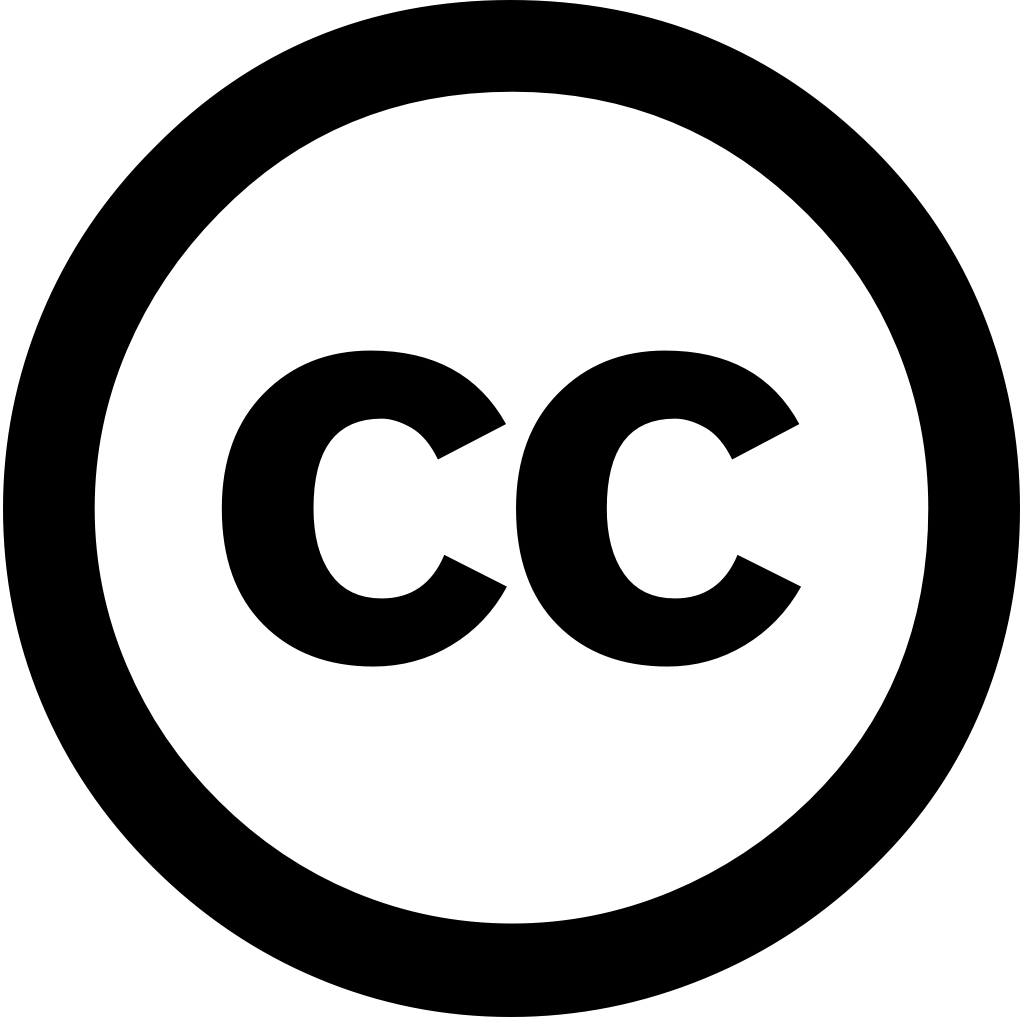
Journal of Marine Science and Engineering, Journal Year: 2025, Volume and Issue: 13(5), P. 902 - 902
Published: April 30, 2025
Marine voyage optimization determines the optimal route and speed to ensure timely arrival. The problem becomes particularly complex when incorporating a dynamic environment, such as future expected weather conditions along unexpected disruptions. This study explores two model-free Deep Reinforcement Learning (DRL) algorithms: (i) Double Q Network (DDQN) (ii) Deterministic Policy Gradient (DDPG). These algorithms are computationally costly, so we split into an offline phase (costly pre-training for route) online where fine-tuned updated data become available. Fine tuning is quick enough en-route adjustments updating planning different dates might be very different. models compared classical heuristic methods: DDPG achieved 4% lower fuel consumption than DDQN was only outperformed by Tabu Search 1%. Both DRL demonstrate high adaptability updates, achieving up 12% improvement in distance-based baseline model. Additionally, they non-graph-based self-learning, making them more straightforward extend integrate digital twin-driven autonomous solutions, traditional approaches.
Language: Английский