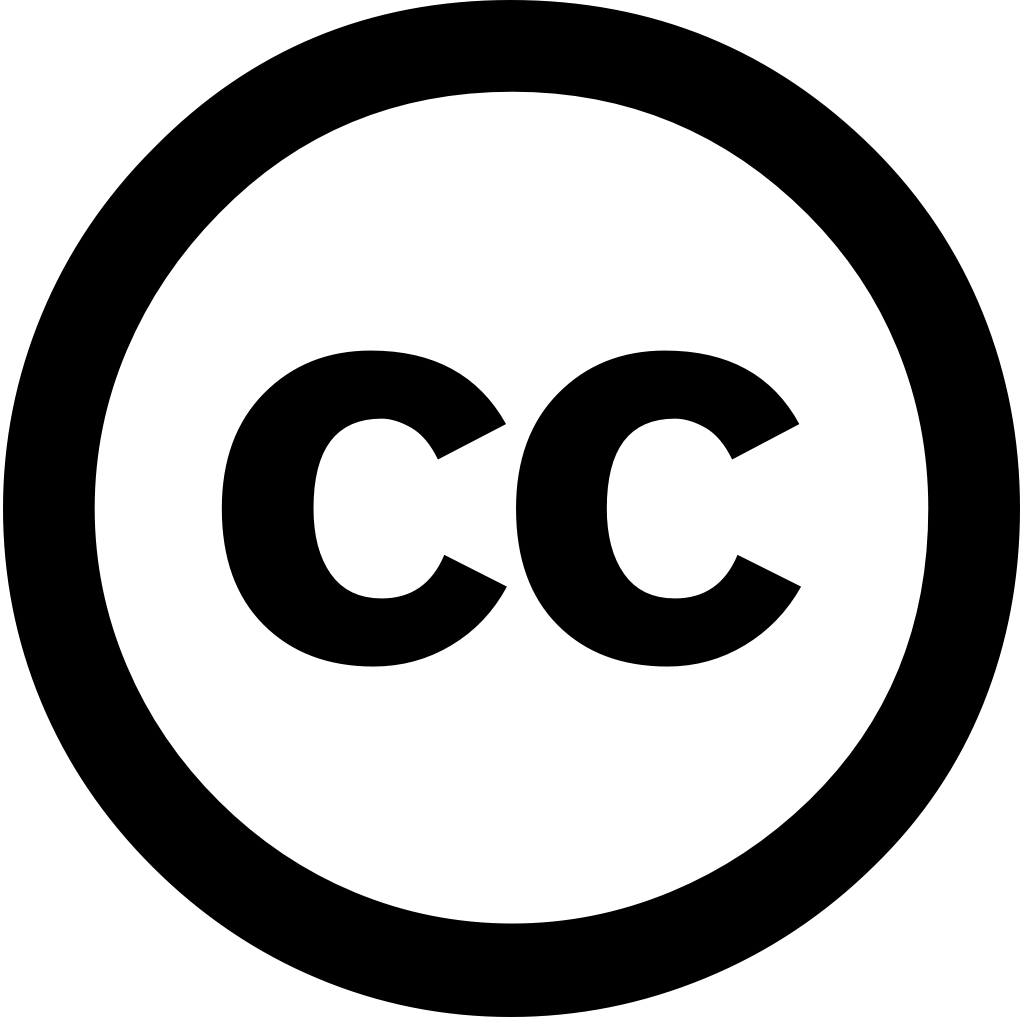
Surgeries, Journal Year: 2025, Volume and Issue: 6(1), P. 8 - 8
Published: Jan. 31, 2025
Background: Lung cancer surgery often involves complex decision-making, where accurate and interpretable predictive models are crucial for assessing postoperative risks optimizing outcomes. This study presents XplainLungSHAP, a novel framework combining SHAP (SHapley Additive exPlanations) attention mechanisms to enhance both accuracy transparency. The aim is support clinicians in preoperative evaluations by identifying prioritizing key clinical features. Methods: was developed using data from 470 patients undergoing lung surgery. Key features were identified through SHAP, ensuring alignment with medical expertise. These dynamically weighted an mechanism neural network, enhancing their impact on survival predictions. model’s performance evaluated accuracy, confusion matrices, ROC analysis, demonstrating its reliability interpretability. Results: XplainLungSHAP model achieved of 91.49%, outperforming traditional machine learning models. analysis critical predictors, including pulmonary function, comorbidities, age, while the prioritized these dynamically. combined approach ensured high offered actionable insights into Conclusions: addresses limitations black-box integrating explainability state-of-the-art techniques. provides transparent clinically relevant tool guiding surgical decisions, supporting personalized care, advancing AI applications thoracic oncology.
Language: Английский