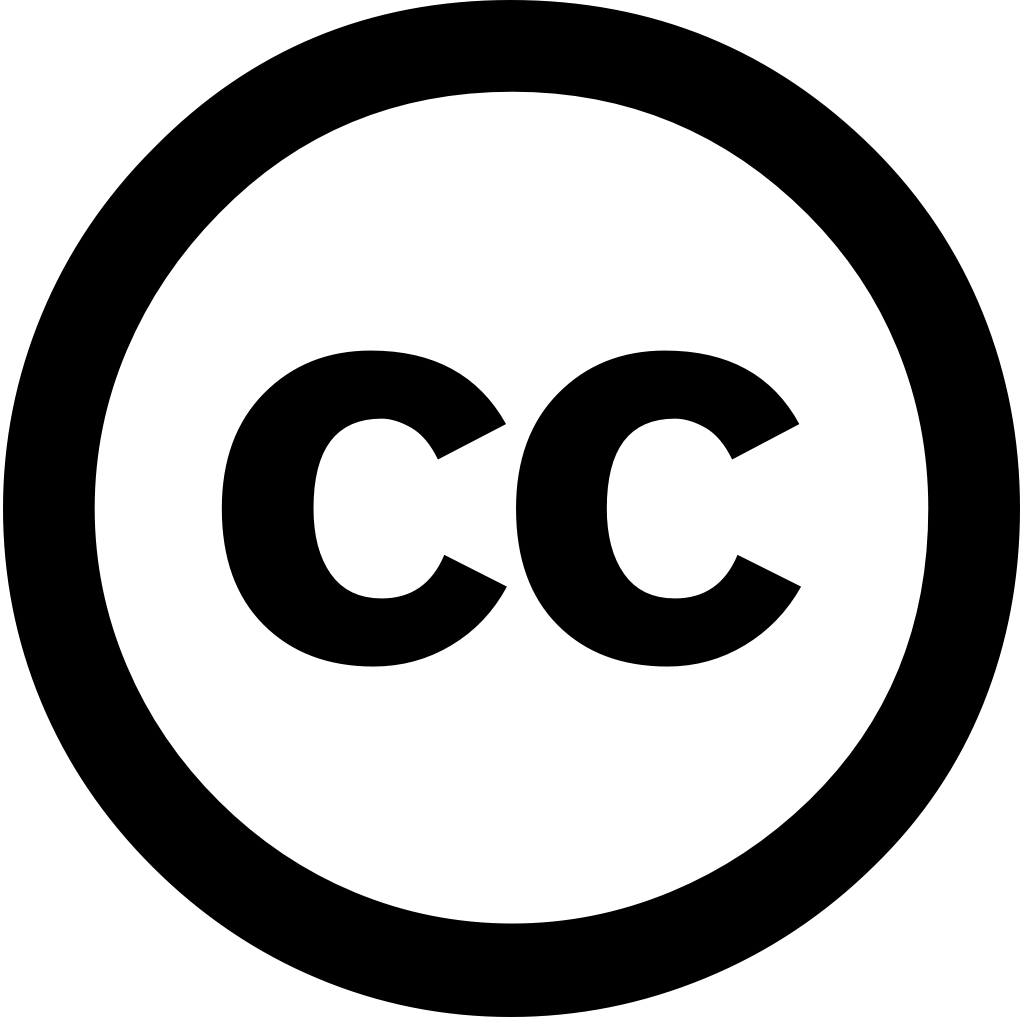
Research Square (Research Square), Journal Year: 2023, Volume and Issue: unknown
Published: Dec. 14, 2023
Language: Английский
Research Square (Research Square), Journal Year: 2023, Volume and Issue: unknown
Published: Dec. 14, 2023
Language: Английский
Scientific Reports, Journal Year: 2023, Volume and Issue: 13(1)
Published: May 8, 2023
Abstract Due to the wide availability of easy-to-access content on social media, along with advanced tools and inexpensive computing infrastructure, has made it very easy for people produce deep fakes that can cause spread disinformation hoaxes. This rapid advancement panic chaos as anyone easily create propaganda using these technologies. Hence, a robust system differentiate between real fake become crucial in this age media. paper proposes an automated method classify images by employing Deep Learning Machine based methodologies. Traditional (ML) systems handcrafted feature extraction fail capture more complex patterns are poorly understood or represented simple features. These cannot generalize well unseen data. Moreover, sensitive noise variations data, which reduce their performance. problems limit usefulness real-world applications where data constantly evolves. The proposed framework initially performs Error Level Analysis image determine if been modified. is then supplied Convolutional Neural Networks extraction. resultant vectors classified via Support Vector Machines K-Nearest Neighbors performing hyper-parameter optimization. achieved highest accuracy 89.5% Residual Network Neighbor. results prove efficiency robustness technique; hence, be used detect potential threat slander propaganda.
Language: Английский
Citations
56Expert Systems with Applications, Journal Year: 2025, Volume and Issue: unknown, P. 126817 - 126817
Published: Feb. 1, 2025
Language: Английский
Citations
1Knowledge-Based Systems, Journal Year: 2023, Volume and Issue: 280, P. 111023 - 111023
Published: Oct. 5, 2023
Language: Английский
Citations
12PeerJ Computer Science, Journal Year: 2025, Volume and Issue: 11, P. e2693 - e2693
Published: March 11, 2025
The proliferation of fake news has become a significant threat, influencing individuals, institutions, and societies at large. This issue been exacerbated by the pervasive integration social media into daily life, directly shaping opinions, trends, even economies nations. Social platforms have struggled to mitigate effects news, relying primarily on traditional methods based human expertise knowledge. Consequently, machine learning (ML) deep (DL) techniques now play critical role in distinguishing necessitating their extensive deployment counter rapid spread misinformation across all languages, particularly Arabic. Detecting Arabic presents unique challenges, including complex grammar, diverse dialects, scarcity annotated datasets, along with lack research field detection compared English. study provides comprehensive review examining its types, domains, characteristics, life cycle, approaches. It further explores recent advancements leveraging ML, DL, transformer-based for detection, special attention delves Arabic-specific pre-processing techniques, methodologies tailored language, datasets employed these studies. Additionally, it outlines future directions aimed developing more effective robust strategies address challenge content.
Language: Английский
Citations
0Lecture notes in networks and systems, Journal Year: 2025, Volume and Issue: unknown, P. 34 - 43
Published: Jan. 1, 2025
Language: Английский
Citations
0Neurocomputing, Journal Year: 2024, Volume and Issue: 598, P. 128078 - 128078
Published: Sept. 1, 2024
Language: Английский
Citations
3Cluster Computing, Journal Year: 2024, Volume and Issue: 27(6), P. 7721 - 7740
Published: March 30, 2024
Language: Английский
Citations
2e-Prime - Advances in Electrical Engineering Electronics and Energy, Journal Year: 2023, Volume and Issue: 6, P. 100370 - 100370
Published: Nov. 22, 2023
The main contribution in this article is proposing a modern meta-heuristic algorithm for solving nonlinear, nonconvex, and complex OPF problems effectively distribution networks, considering RES. Fire Hawk Optimizer (FHO) proposed work. considered objective function (OF) the combined minimization of generating cost, active power loss (Plosses), load bus voltage deviation (VD). strategy validated using standard IEEE 69-bus benchmark. obtained results are compared with various recent techniques such as Capuchin Search Algorithm (CSA), Bear Smell (BSSA), Black Widow Optimization (BWO) Algorithm, Walrus (WaOA), Prairie Dog (PDO) Algorithm. One-way ANOVA Tukey test, two common statistical tests, used to compare algorithms effectively. FHO shows best superior performance minimum mean cost values 51.2711 5.1131 respectively. This demonstrates that more consistent less diverse. lower computational time (25.3241 s) other strategies. confirm approach's reliability, stability, consistency. method provided reliable, high-quality faster computing times. Additionally, it has significant convergence makes networks variable RES NRES.
Language: Английский
Citations
5Jurnal Teknik Industri, Journal Year: 2023, Volume and Issue: 24(1), P. 65 - 80
Published: March 28, 2023
The current energy crisis is a pressing global challenge, with the industrial sector accounting for half of consumption. Scheduling considered one potential methods to reduce This article introduces Fire Hawk Optimizer (FHO) algorithm solve no-idle flow shop scheduling problem minimize overall FHO organizes job sequence in research investigates use different machine speed levels, namely slow, fast, and normal, based on case data manufacturing industries Indonesia. results this study compare performance Adaptive Integrated Greedy (AIG) heuristic method it Grey Wolf (GWO) algorithm. experimental showed that total consumption tends be high when processed at speed. Conversely, low-speed lower but requires longer processing time. comparison show more efficient reducing than AIG method. Meanwhile, performs comparably GWO completes enumeration. These findings confirm proposed procedure can an alternative optimization process.
Language: Английский
Citations
4International Journal of Knowledge-based and Intelligent Engineering Systems, Journal Year: 2024, Volume and Issue: unknown, P. 1 - 13
Published: Jan. 11, 2024
Multitask learning (MTL) is a machine paradigm where single model trained to perform several tasks simultaneously. Despite the considerable amount of research on MTL, majority it has been centered around English language, while other language such as Arabic have not received much attention. Most existing NLP techniques concentrate or multitask learning, sharing just limited number tasks, between two three tasks. To address this gap, we present ArMT-TNN, an Multi-Task Learning using Transformer Neural Network, designed for natural understanding (ANLU) Our approach involves learned information eight ANLU allowing solve all them. We achieve by fine-tuning simultaneously and multiple pre-trained Bidirectional models, like BERT, that are specifically processing. Additionally, explore effectiveness various models (LMs) different types text, Modern Standard (MSA) dialects. demonstrated outstanding performance compared current four test sets within ALUE benchmark, namely MQ2Q, OOLD, SVREG, SEC, margins 3.9%, 3.8%, 10.1%, 3.7%, respectively. Nonetheless, our did well remaining due negative transfer knowledge. This finding highlights importance carefully selecting when constructing benchmark. experiments also show LMs which were pretrained text differ from type used finetuned can still well.
Language: Английский
Citations
1