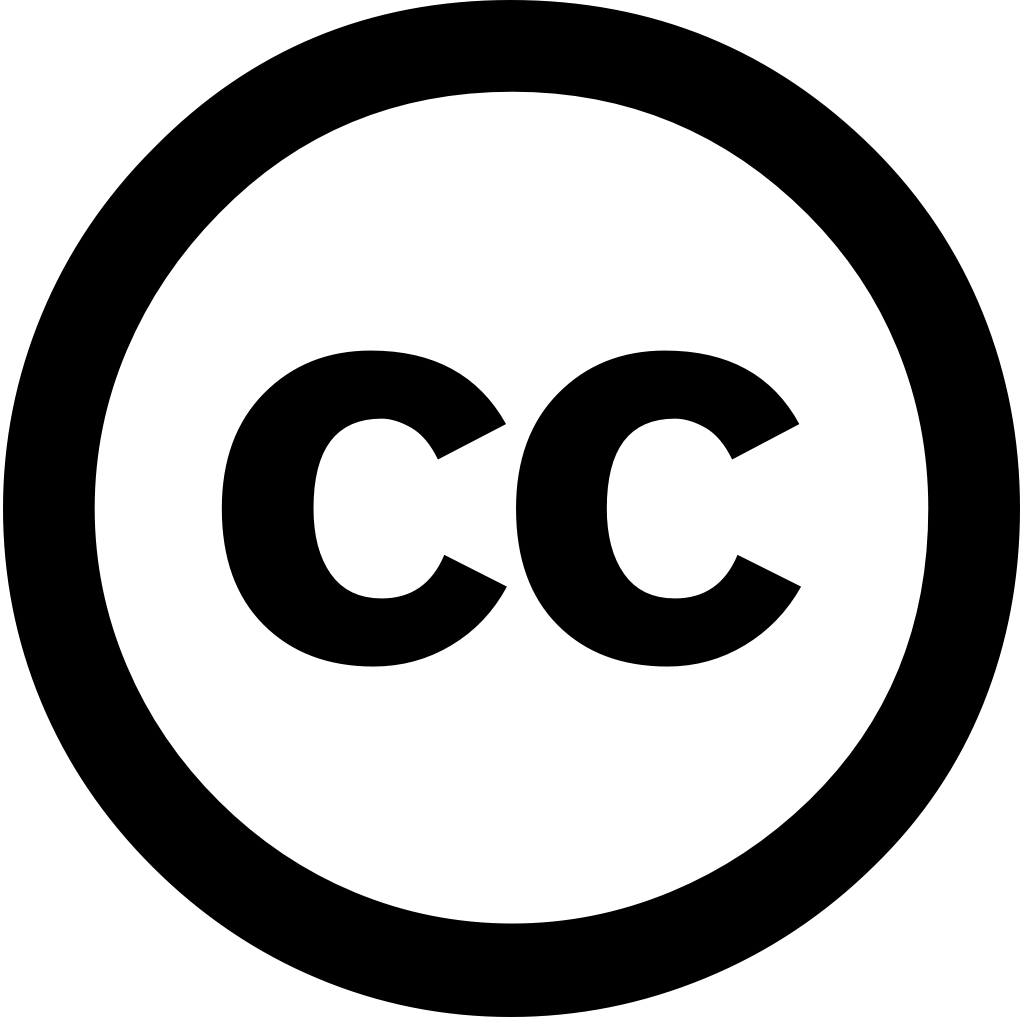
Heliyon, Journal Year: 2024, Volume and Issue: 10(19), P. e37876 - e37876
Published: Sept. 18, 2024
Language: Английский
Heliyon, Journal Year: 2024, Volume and Issue: 10(19), P. e37876 - e37876
Published: Sept. 18, 2024
Language: Английский
Scientific Reports, Journal Year: 2023, Volume and Issue: 13(1)
Published: Oct. 30, 2023
The investigation compares the conventional, advanced machine, deep, and hybrid learning models to introduce an optimum computational model assess ground vibrations during blasting in mining projects. long short-term memory (LSTM), artificial neural network (ANN), least square support vector machine (LSSVM), ensemble tree (ET), decision (DT), Gaussian process regression (GPR), (SVM), multilinear (MLR) are employed using 162 data points. For first time, blackhole-optimized LSTM has been used predict blasting. Fifteen performance metrics have implemented measure prediction capabilities of models. study concludes that blackhole optimized-LSTM PPV11 is highly capable predicting vibration. Model assessed with RMSE = 0.0181 mm/s, MAE 0.0067 R 0.9951, a20 96.88, IOA 0.9719, IOS 0.0356 testing. Furthermore, this reveals accuracy less affected by multicollinearity because optimization algorithm. external cross-validation literature validation confirm PPV11. ANOVA Z tests reject null hypothesis for actual vibration, Anderson-Darling test rejects predicted This also GPR LSSVM overfit moderate problematic assessing vibration
Language: Английский
Citations
62Buildings, Journal Year: 2024, Volume and Issue: 14(3), P. 591 - 591
Published: Feb. 22, 2024
Landscape geopolymer concrete (GePoCo) with environmentally friendly production methods not only has a stable structure but can also effectively reduce environmental damage. Nevertheless, GePoCo poses challenges its intricate cementitious matrix and vague mix design, where the components their relative amounts influence compressive strength. In response to these challenges, application of accurate applicable soft computing techniques becomes imperative for predicting strength such composite matrix. This research aimed predict using waste resources through novel ensemble ML algorithm. The dataset comprised 156 statistical samples, 15 variables were selected prediction. model employed combination RF, GWO algorithm, XGBoost. A stacking strategy was implemented by developing multiple RF models different hyperparameters, combining outcome predictions into new dataset, subsequently XGBoost model, termed RF–XGBoost model. To enhance accuracy errors, algorithm optimized hyperparameters resulting in RF–GWO–XGBoost proposed compared stand-alone models, hybrid GWO–XGBoost system. results demonstrated significant performance improvement strategies, particularly assistance exhibited better effectiveness, an RMSE 1.712 3.485, R2 0.983 0.981. contrast, (RF XGBoost) lower performance.
Language: Английский
Citations
26Buildings, Journal Year: 2024, Volume and Issue: 14(2), P. 396 - 396
Published: Feb. 1, 2024
Fiber-reinforced nano-silica concrete (FrRNSC) was applied to a sculpture address the issue of brittle fracture, and primary objective this study explore potential hybridizing Grey Wolf Optimizer (GWO) with four robust intelligent ensemble learning techniques, namely XGBoost, LightGBM, AdaBoost, CatBoost, anticipate compressive strength fiber-reinforced for sculptural elements. The optimization hyperparameters these techniques performed using GWO metaheuristic algorithm, enhancing accuracy through creation hybrid models: GWO-XGBoost, GWO-LightGBM, GWO-AdaBoost, GWO-CatBoost. A comparative analysis conducted between results obtained from models their conventional counterparts. evaluation is based on five key indices: R2, RMSE, VAF, MAE, bias, addressing an assessment predictive models’ performance capabilities. outcomes reveal that exhibiting R2 values (0.971 0.978) train test stages, respectively, emerges as best model estimating compared other models. Consequently, proposed GWO-XGBoost algorithm proves be efficient tool anticipating CSFrRNSC.
Language: Английский
Citations
19Artificial Intelligence Review, Journal Year: 2025, Volume and Issue: 58(4)
Published: Jan. 30, 2025
Language: Английский
Citations
2Mathematical Biosciences & Engineering, Journal Year: 2023, Volume and Issue: 21(1), P. 1413 - 1444
Published: Jan. 1, 2023
<abstract> <p>The green concretes industry benefits from utilizing gel to replace parts of the cement in concretes. However, measuring compressive strength geo-polymer (CSGPoC) needs a significant amount work and expenditure. Therefore, best idea is predicting CSGPoC with high level accuracy. To do this, base learner super machine learning models were proposed this study anticipate CSGPoC. The decision tree (DT) applied as learner, random forest extreme gradient boosting (XGBoost) techniques are used system. In regard, database was provided involving 259 data samples, which four-fifths considered for training model one-fifth selected testing models. values fly ash, ground-granulated blast-furnace slag (GGBS), Na2SiO3, NaOH, fine aggregate, gravel 4/10 mm, 10/20 water/solids ratio, NaOH molarity input estimate evaluate reliability performance (DT), XGBoost, (RF) models, 12 evaluation metrics determined. Based on obtained results, highest degree accuracy achieved by XGBoost mean absolute error (MAE) 2.073, percentage (MAPE) 5.547, Nash–Sutcliffe (NS) 0.981, correlation coefficient (R) 0.991, R<sup>2</sup> 0.982, root square (RMSE) 2.458, Willmott's index (WI) 0.795, weighted (WMAPE) 0.046, Bias (SI) 0.054, p 0.027, relative (MRE) -0.014, a<sup>20</sup> 0.983 MAE 2.06, MAPE 6.553, NS 0.985, R 0.993, 0.986, RMSE 2.307, WI 0.818, WMAPE 0.05, SI 0.056, 0.028, MRE -0.015, 0.949 model. By importing set into trained 0.8969, 0.9857, 0.9424 DT, RF, respectively, show superiority estimation. conclusion, capable more accurately than DT RF models.</p> </abstract>
Language: Английский
Citations
29Resources Policy, Journal Year: 2023, Volume and Issue: 85, P. 103903 - 103903
Published: July 11, 2023
Language: Английский
Citations
28Gels, Journal Year: 2024, Volume and Issue: 10(2), P. 148 - 148
Published: Feb. 16, 2024
As an environmentally responsible alternative to conventional concrete, geopolymer concrete recycles previously used resources prepare the cementitious component of product. The challenging issue with employing in building business is absence a standard mix design. According chemical composition its components, this work proposes thorough system or framework for estimating compressive strength fly ash-based (FAGC). It could be possible construct predicting FAGC using soft computing methods, thereby avoiding requirement time-consuming and expensive experimental tests. A complete database 162 datasets was gathered from research papers that were published between years 2000 2020 prepared develop proposed models. To address relationships inputs output variables, long short-term memory networks deployed. Notably, model examined several methods. modeling process incorporated 17 variables affect CSFAG, such as percentage SiO
Language: Английский
Citations
17Neural Computing and Applications, Journal Year: 2024, Volume and Issue: 36(29), P. 18387 - 18412
Published: July 26, 2024
Abstract This study proposes a novel system for accurately predicting grout’s uniaxial compressive strength (UCS) in fully grouted rock bolting systems. To achieve this, database comprising 73 UCS values with varying water-to-grout (W/G) ratios ranging from 22 to 42%, curing times 1 28 days, the admixture of fly ash contents 0 30%, and two Australian commercial grouts, Stratabinder HS, BU-100, was built after conducting comprehensive series experimental tests. After building dataset, metaheuristic technique, jellyfish search (JS) algorithm employed determine weight base models ensemble system. combined various data modelling techniques enhance accuracy predictions. What sets this technique apart is innovative use JS create weighted averaging model, going beyond traditional methods grout strength. The proposed model called (WAE-JS), which obtained results several soft computing such as multi-layer perceptron (MLP), Bayesian regularized (BR) neural networks, generalized feed-forward (GFF) classification regression tree (CART), random forest (RF) were based on new then generated. Eventually, result WAE-JS compared other models, including MLP, BR, GFF, CART, RF, some statistical parameters, R-squared coefficients, RMSE, VAF indices evaluating performance capability model. suggested superiority over models. In addition, effectively improved achieved RF. Furthermore, sensitivity analysis revealed that W/G had most significant impact values.
Language: Английский
Citations
10Natural Resources Research, Journal Year: 2023, Volume and Issue: 32(6), P. 2995 - 3023
Published: Sept. 4, 2023
Language: Английский
Citations
19Buildings, Journal Year: 2023, Volume and Issue: 13(9), P. 2278 - 2278
Published: Sept. 7, 2023
The main goal of the present study is to investigate capability hybridizing imperialist competitive algorithm (ICA) with an intelligent, robust, and data-driven technique named light gradient boosting machine (LightGBM) estimate compressive strength geo-polymer concrete (CSGCo). hyper-parameters LightGBM have been optimized based on ICA its accuracy improved. obtained results from proposed hybrid ICA-LightGBM are compared traditional model as well four different topologies artificial neural networks (ANN) comprising a multi-layer perceptron network (MLP), radial basis function (RBF), generalized feed-forward (GFFNN), Bayesian regularized (BRNN). these models were three evaluation indices R2, RMSE, VAF for providing objective performance predictive models. Concerning outcomes, R2 (0.9871 0.9805), RMSE (0.4703 1.3137), (98.5773 98.0397) training testing phases, respectively, was superior predictor CSGCo (0.9488 0.9478), (0.9532 2.1631), (94.3613 94.5173); MLP (0.9067 0.8959), (1.3093 3.3648), (88.9888 84.9125); RBF (0.8694 0.8055), (1.4703 5.0309), (86.3122 66.1888); BRNN (0.9212 0.9107), (1.1510 2.6569), (91.4168 90.5854); GFFNN (0.9144 0.8925), (1.1525 2.9415), (91.4092 88.9088). Hence, can be efficiently used in anticipating CSGCo.
Language: Английский
Citations
17