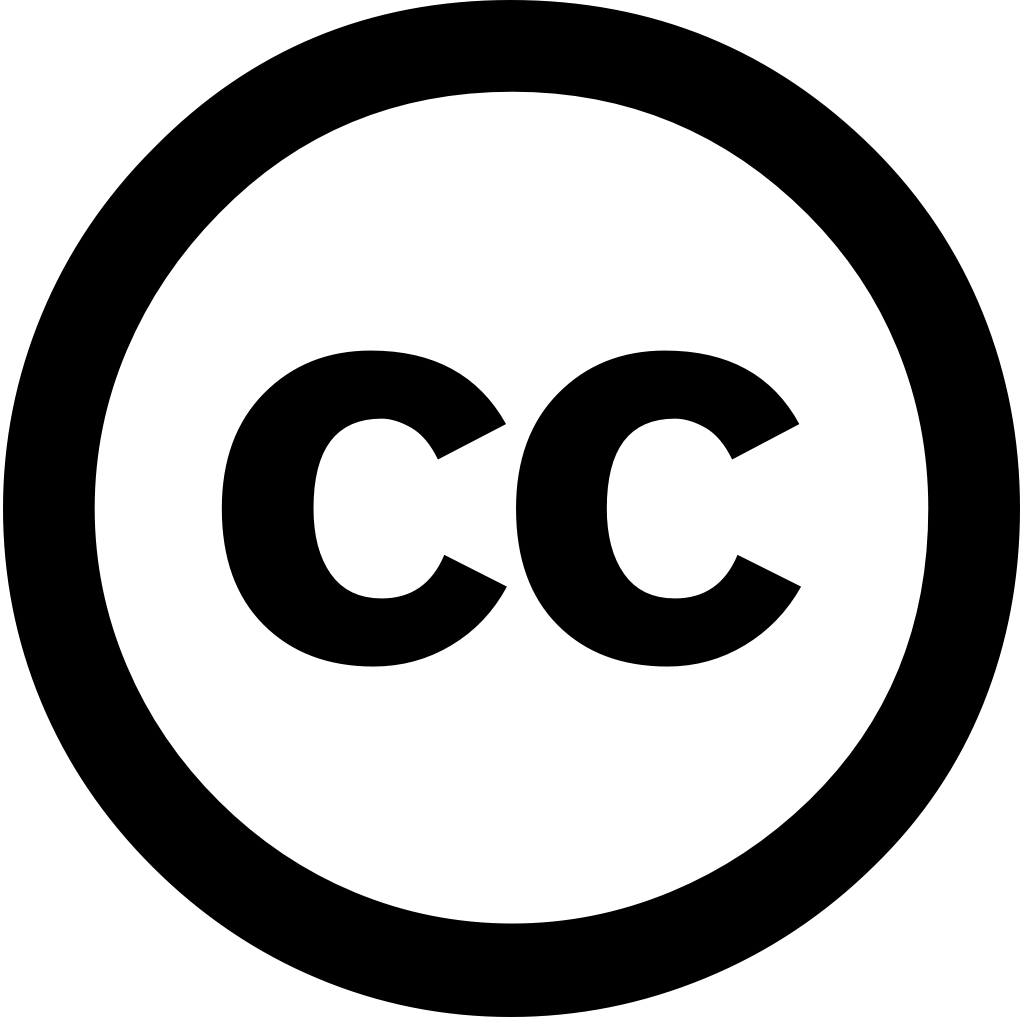
Applied Sciences, Journal Year: 2024, Volume and Issue: 14(23), P. 11342 - 11342
Published: Dec. 5, 2024
Emotion recognition is crucial for enhancing human–machine interactions by establishing a foundation AI systems that integrate cognitive and emotional understanding, bridging the gap between machine functions human emotions. Even though deep learning algorithms are actively used in this field, study of sequence modeling accounts shifts emotions over time has not been thoroughly explored. In research, we present comprehensive speech emotion-recognition framework amalgamates ZCR, RMS, MFCC feature sets. Our approach employs both CNN LSTM networks, complemented an attention model, enhanced emotion prediction. Specifically, model addresses challenges long-term dependencies, enabling system to factor historical experiences alongside current ones. We also incorporate psychological “peak–end rule”, suggesting preceding states significantly influence emotion. The plays pivotal role restructuring input dimensions, facilitating nuanced processing. rigorously evaluated proposed utilizing two distinct datasets, namely TESS RAVDESS. empirical outcomes highlighted model’s superior performance, with accuracy rates reaching 99.8% 95.7% These results notable advancement, showcasing our system’s precision innovative contributions recognition.
Language: Английский