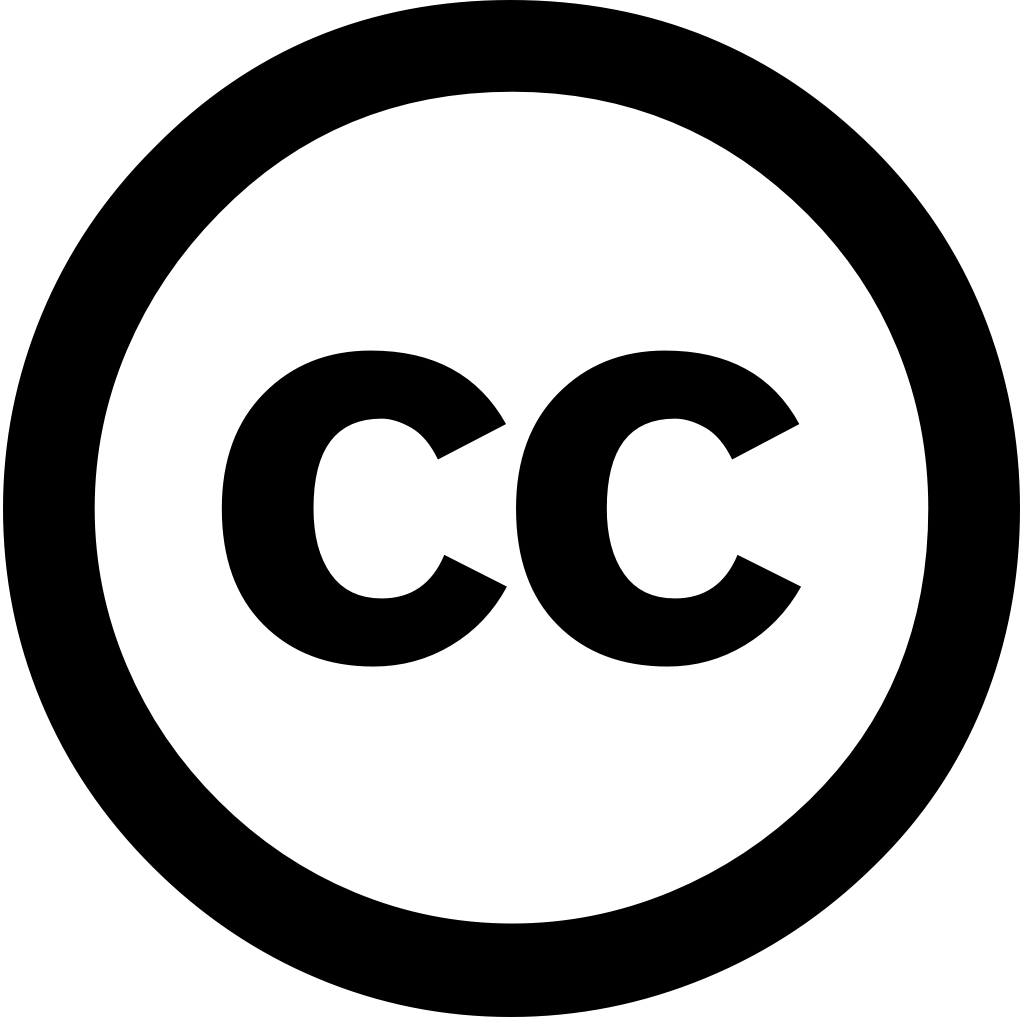
Mathematics, Journal Year: 2024, Volume and Issue: 12(10), P. 1431 - 1431
Published: May 7, 2024
Feature selection in classification is a complex optimization problem that cannot be solved polynomial time. Bi-objective feature selection, aiming to minimize both selected features and errors, challenging due the conflict between objectives, while one of most effective ways tackle this use multi-objective evolutionary algorithms. However, very few these have ever reflected an multi-tasking framework, despite implicit parallelism offered by population-based search characteristic. In paper, dynamic multi-tasking-based algorithm (termed DTEA) proposed for handling bi-objective classification, which not only suitable datasets with relatively lower dimensionality features, but also higher features. The role influence on were studied, tasking mechanism self-adaptively assign multiple tasks intermittently analyzing population behaviors. efficacy DTEA tested 20 compared seven state-of-the-art A component contribution analysis was conducted comparing its three variants. empirical results show dynamic-tasking works efficiently enables outperform other algorithms terms classification.
Language: Английский