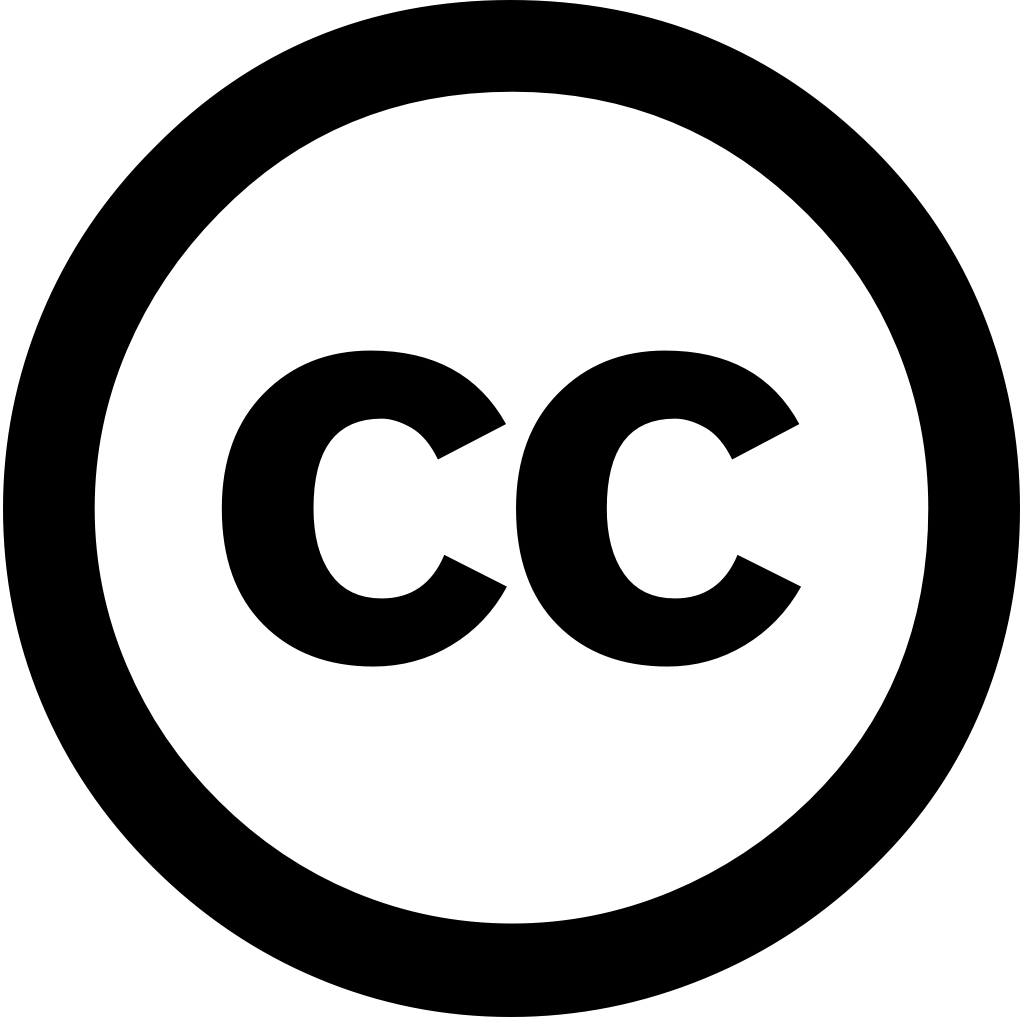
Bioengineering, Journal Year: 2024, Volume and Issue: 11(10), P. 1032 - 1032
Published: Oct. 16, 2024
This paper presents a deep-learning architecture for segmenting retinal fluids in patients with Diabetic Macular Oedema (DME) and Age-related Degeneration (AMD). Accurate segmentation of multiple fluid types is critical diagnosis treatment planning, but existing techniques often struggle precision. We propose an encoder–decoder network inspired by U-Net, processing enhanced OCT images their edge maps. The encoder incorporates Residual Inception modules autoencoder-based multiscale attention mechanism to extract detailed features. Our method shows superior performance across several datasets. On the RETOUCH dataset, achieved F1 Scores 0.82 intraretinal (IRF), 0.93 subretinal (SRF), 0.94 pigment epithelial detachment (PED). model also performed well on OPTIMA DUKE datasets, demonstrating high precision, recall, Scores. significantly enhances accuracy offering valuable tool diagnosing managing diseases. Its integration dual-input processing, attention, advanced highlights its potential improve clinical outcomes advance disease treatment.
Language: Английский