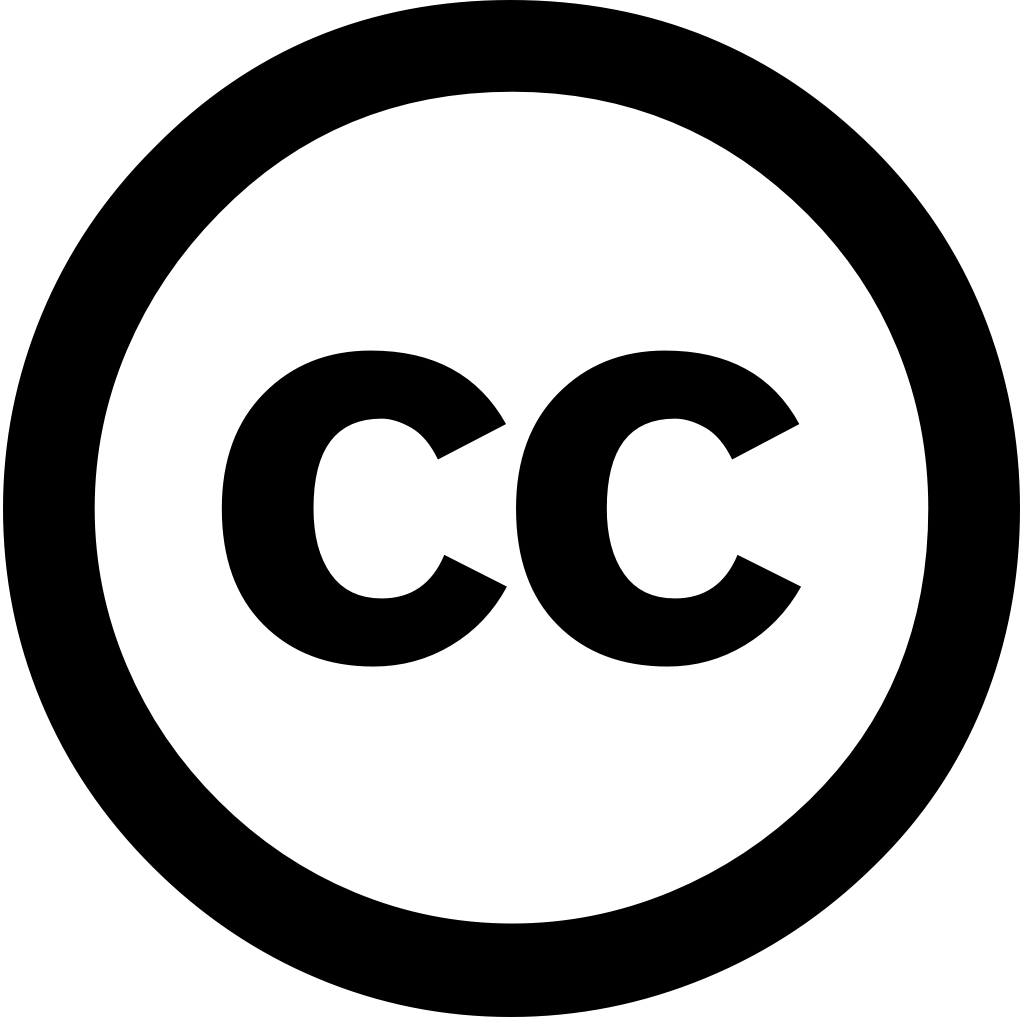
Biomolecules, Journal Year: 2025, Volume and Issue: 15(4), P. 524 - 524
Published: April 3, 2025
Molecular modelling is a vital tool in the discovery and characterisation of bioactive peptides, providing insights into their structural properties interactions with biological targets. Many models predicting peptide function or structure rely on intrinsic properties, including influence amino acid composition, sequence, chain length, which impact stability, folding, aggregation, target interaction. Homology predicts structures based known templates. Peptide-protein can be explored using molecular docking techniques, but there are challenges related to inherent flexibility addressed by more computationally intensive approaches that consider movement over time, called dynamics (MD). Virtual screening many usually against single target, enables rapid identification potential peptides from large libraries, typically approaches. The integration artificial intelligence (AI) has transformed leveraging amounts data. AlphaFold general protein prediction deep learning greatly improved predictions conformations interactions, addition estimates model accuracy at each residue guide interpretation. Peptide being further enhanced Protein Language Models (PLMs), deep-learning-derived statistical learn computer representations useful identify fundamental patterns proteins. Recent methodological developments discussed context canonical as well those modifications cyclisations. In designing therapeutics, main outstanding challenge for these methods incorporation diverse non-canonical acids
Language: Английский