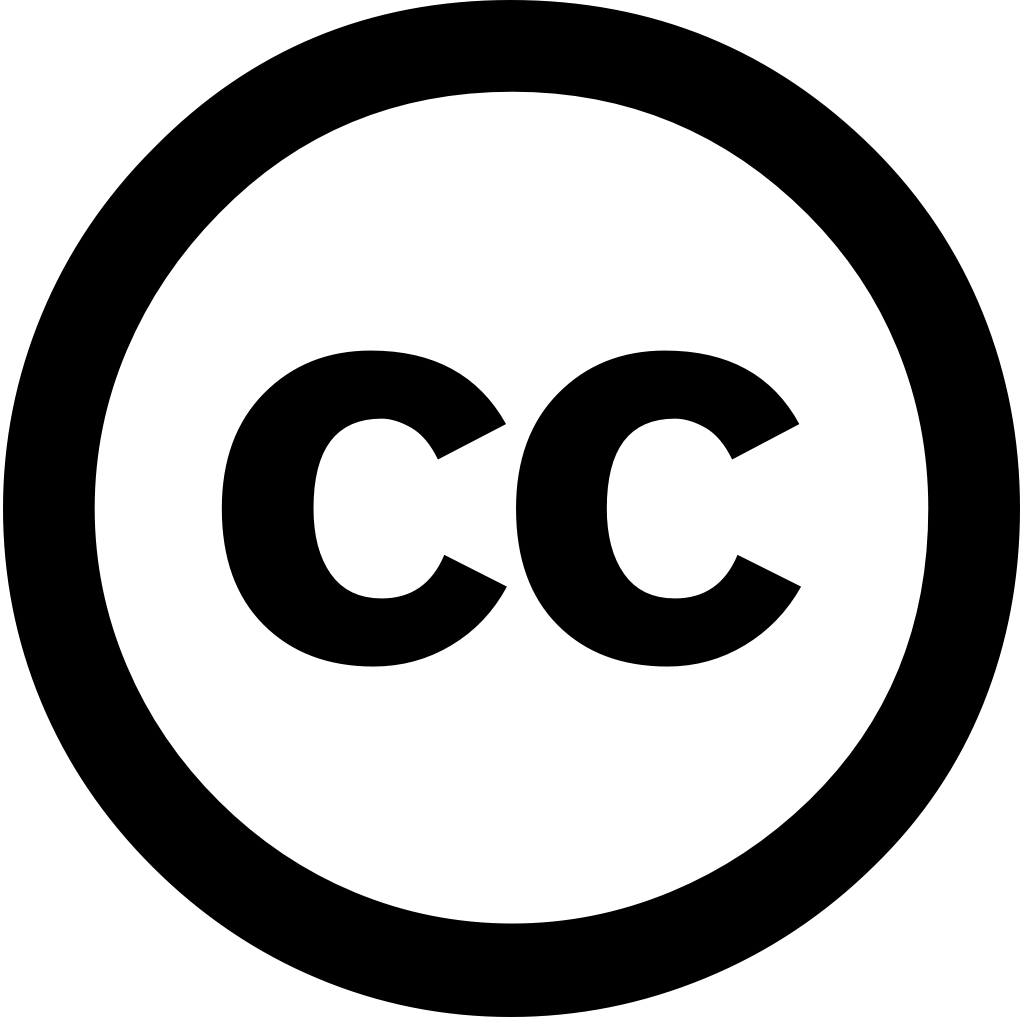
Virtual and Physical Prototyping, Journal Year: 2025, Volume and Issue: 20(1)
Published: March 31, 2025
Additive manufacturing of ceramics, specifically through vat-photopolymerization, offers significant potential due to its high precision and ability produce complex geometries. This study addresses the primary challenge in vat-photopolymerization: developing binder formulations that optimise both viscosity mechanical properties while accommodating ceramic loadings. introduces supervised machine learning (ML) algorithms as a novel approach predict tensile strength formulations. A comprehensive dataset was generated using full factorial experimental design with three factors at levels. Various ML were evaluated for their efficacy regression applications. The leave-one-out cross-validation (LOOCV) method employed assess performance these small size. ANN model 5 hidden nodes delivered exceptional results, achieving mean absolute errors 2.504 mPa·s 1.163 MPa strength, outperforming other models. models particularly adept capturing non-linear relationships. inclusion Okoruwa Maximum Saturation Potential (OMSP) area peak position input features significantly enhanced predictive accuracy properties. research demonstrates remarkable revolutionise formulation process VPP resins.
Language: Английский