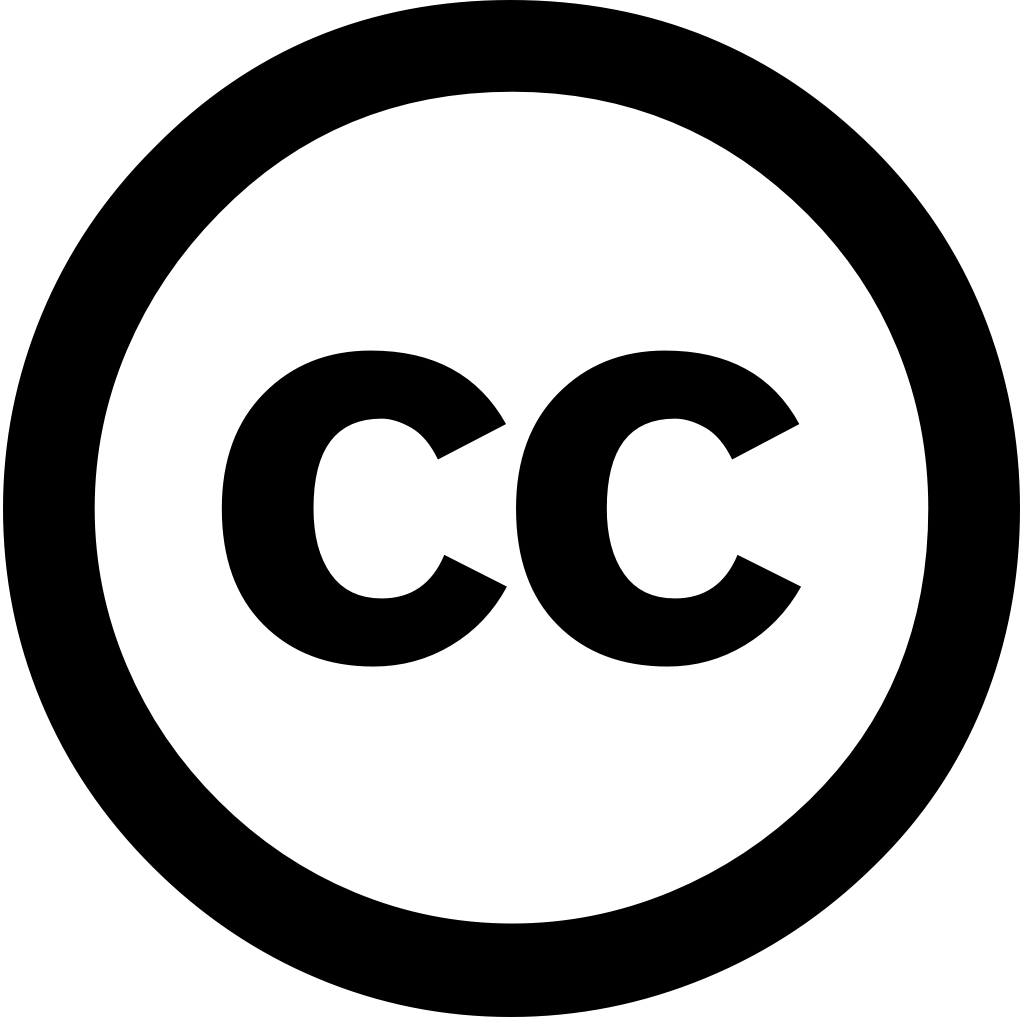
Sensors, Journal Year: 2025, Volume and Issue: 25(5), P. 1392 - 1392
Published: Feb. 25, 2025
In the field of sustainable mobility, this study highlights importance using machine learning for predictive modeling based on real traffic data collected from instrumented bicycles. The advent advanced technologies like mobility apps, sensors, and analysis methods led to ability collect various sources, which enabled researchers estimate battery state charge (SOC) accurately. Most current research uses them in lab experiments collection. work, we use real-time sensors construct data-driven models lithium-ion SOC estimation. This integrates both electric bicycle battery, environmental route variables achieve following goals: (1) Collect a multimodal set including operational, topography, vehicle, external variables, (2) Preprocess obtained installed (3) Create (4) Assess compare their performance with high accuracy. To that, conducted predict Remaining Useful Life (RUL), as measure charge, battery. was carried out 15 km cycle Medellín, Colombia, 28 days. RUL, used four different algorithms: Long Short-Term Memory (LSTM), Support Vector Regression (SVR), AdaBoost, Gradient Boost. Notably, preprocessing techniques played pivotal role, particular focus smoothing sensor Convolutional Neural Networks (CNN). results showed significant improvement prediction accuracy when preprocessing, confirming its improving model performance. Furthermore, comparison network facilitated selection most effective test data. underscores value real-world develop validate pursuit solutions, critical role methodologies addressing today’s urban transportation challenges.
Language: Английский