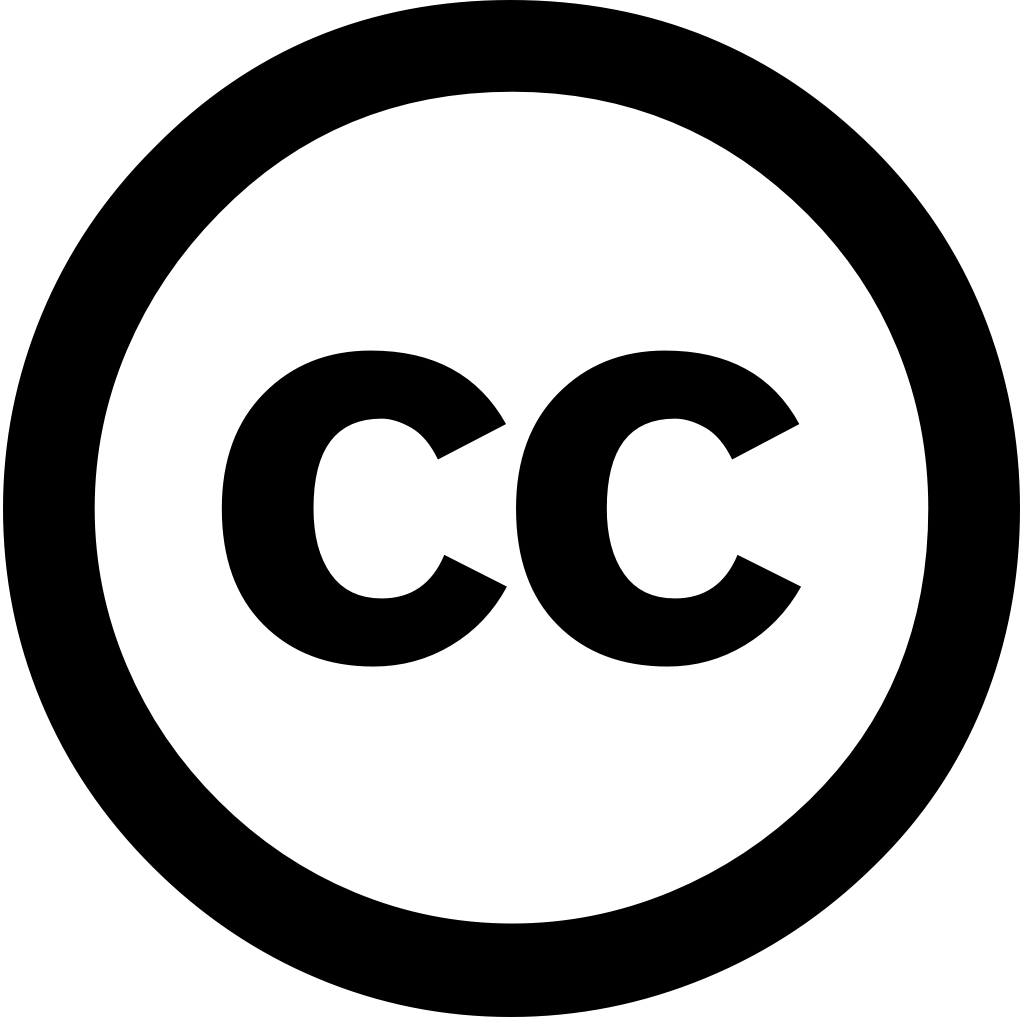
Advances in Space Research, Journal Year: 2021, Volume and Issue: 68(4), P. 1761 - 1788
Published: April 24, 2021
Language: Английский
Advances in Space Research, Journal Year: 2021, Volume and Issue: 68(4), P. 1761 - 1788
Published: April 24, 2021
Language: Английский
Remote Sensing Applications Society and Environment, Journal Year: 2022, Volume and Issue: 29, P. 100907 - 100907
Published: Dec. 18, 2022
Google Earth Engine (GEE) is a geospatial processing platform based on geo-information applications in the 'cloud'. This provides free access to huge volumes of satellite data for computing, and offers support tools monitor analyse environmental features large scale. Such facilities have been widely used numerous studies about land management planning. Considering current lack relevant overviews, it may be useful evaluate utilization paths GEE its impact scientific community. For this purpose, systematic review has conducted using PRISMA methodology 343 articles published from 2020 2022 high-impact journals, selected Scopus Scholar databases. After an overview publishing context, analysis frequency features, methods, are carried out, special attention given COVID-19 studies. Finally, geographical distribution reviewed evaluated, citation metrics analysed. On bibliometric approach, 90 journals reference period (January April 2022), number reveals multidisciplinary application as well interest publishers towards topic relevance international The results meta-analysis following showed that: (i) Landsat 8 was most widely-used (25%); non-parametric classification mainly Random Forest, were recurrent algorithms (31%); (iii) water resources assessment prediction common methodological (22%). A low COVID-19, spite planetary importance pandemic effects. geographically distributed among 86 countries, China, United States, India accounting number. 'Remote Sensing' Sensing Environment' leading metrics, while Forest method agriculture-related being mostly cited. It expected that these might change over mid long term, due fast progress spatial information technologies, although currently our findings worthwhile assessing global deployment platform.
Language: Английский
Citations
64International Journal of Applied Earth Observation and Geoinformation, Journal Year: 2023, Volume and Issue: 118, P. 103288 - 103288
Published: April 1, 2023
Large-scale and dynamic surface water mapping is crucial for understanding the impact of global climate change human activities on distribution resources. Remote sensing imagery has become primary data source due to its high spatiotemporal resolution wide coverage. However, reliability current products during flood seasons limited influence clouds optical remote images. Moreover, annual seasonal cannot capture intra-month variations bodies. To address these challenges, we proposed a framework Google Earth Engine that combines multi-source data. Our can generate 10 m spatial maps at 15-day time step. We classified bodies using Sentinel-2 images classification tree algorithm, then used Sentinel-1 compensate cloudy missing areas in images, resulting seamless cloud-unaffected maps. evaluated effectiveness our six floodplains around world, experimental results demonstrate generated by outperform existing public datasets great potential hydrological applications. details dynamics with higher temporal free from cloud influence, which necessary resources management, monitoring, disaster response.
Language: Английский
Citations
28Ecological Indicators, Journal Year: 2021, Volume and Issue: 129, P. 107863 - 107863
Published: June 4, 2021
Côte d'Ivoire and Ghana are the largest producers of cocoa in world. In recent decades cultivation this crop has led to loss vast tracts forest areas both countries. Efficient accurate methods for remotely identifying plantations essential implementation sustainable practices periodic effective monitoring forests. study, a method plantation identification was developed based on multi-temporal stack Sentinel-1 Sentinel-2 images multi-feature Random Forest (RF) algorithm. The Normalized Difference Vegetation Index (NDVI) second-order texture features were assessed their importance an RF classification, optimal combination used as input variables model identify model-based map achieved 82.89% producer's 62.22% user's accuracy, detecting 3.69 million hectares (Mha) 2.15 Mha Ghana, respectively. results demonstrate that classification can distinguish from other land cover/use, effectively reducing feature dimensions improving efficiency. also highlight farms largely encroach into protected (PAs), 20% detected area is located PAs almost 70% study house plantations.
Language: Английский
Citations
45Water, Journal Year: 2022, Volume and Issue: 14(12), P. 1902 - 1902
Published: June 13, 2022
Mapping water bodies with a high accuracy is necessary for resource assessment, and mapping them rapidly flood monitoring. Poyang Lake the largest freshwater lake in China, its wetland one of most important world. affected by floods from Yangtze River basin every year, fluctuation area level directly or indirectly affects ecological environment Lake. Synthetic Aperture Radar (SAR) particularly suitable large-scale body mapping, as SAR allows data acquisition regardless illumination weather conditions. The two-satellite Sentinel-1 constellation, providing C-Band data, passes over about five times month. With temporal-spatial resolution, can be used to accurately monitor body. After acquiring all (1A 1B) ensure consistency processing, we propose use Python SeNtinel Application Platform (SNAP)-based engine (SARProcMod) process construct dataset 10 m resolution. To extract information an automatic classification based on modified U-Net convolutional neural network (WaterUNet), which classifies using artificial sample datasets validation accuracy. results show that maximum minimum areas our study were 2714.08 km2 20 July 2020, 634.44 4 January 2020. Compared gauging station, was highly correlated level, correlation coefficient being up 0.92 R2 quadratic polynomial fitting 0.88; thus, resulting relationship estimate According results, conclude WaterUNet are very monitoring well emergency mapping.
Language: Английский
Citations
29Journal of Hydrology, Journal Year: 2023, Volume and Issue: 620, P. 129455 - 129455
Published: March 29, 2023
Language: Английский
Citations
19Remote Sensing, Journal Year: 2023, Volume and Issue: 15(6), P. 1517 - 1517
Published: March 9, 2023
Rice is a globally significant staple food crop. Therefore, it crucial to have adequate tools for monitoring changes in the extent of rice paddy cultivation. Such system would require sustainable and operational workflow that employs open-source medium high spatial temporal resolution satellite imagery efficient classification techniques. This study used similar phenological data from Sentinel-2 (S2) optical Sentinel-1 (S1) Synthetic Aperture Radar (SAR) identify distribution with deep learning (DL) Using Google Earth Engine (GEE) U-Net Convolutional Neural Networks (CNN) segmentation, accurately delineates smallholder fields using multi-temporal S1 SAR S2 was investigated. The study′s accuracy assessment results showed optimal dataset mapping fusion multispectral bands (visible near infra-red (VNIR), red edge (RE) short-wave infrared (SWIR)), S1-SAR dual polarization (VH VV) captured within crop growing season (i.e., vegetative, reproductive, ripening). Compared random forest (RF) classification, DL model ResU-Net) had an overall 94% (three percent higher than RF prediction). ResU-Net prediction F1-Score 0.92 compared 0.84 generated 500 trees model. classified maps dates analyzed 2016–2020), change detection analysis over two epochs (2016 2018 2020) provided better understanding spatial–temporal dynamics agriculture area. indicated 377,895 8551 hectares were converted other land-use first (2016–2018) second (2018–2020) epochs. These statistics valuable insight into field across selected districts analyzed. proposed framework has potential be upscaled transferred regions. approach could locally, improve decision making, support security region.
Language: Английский
Citations
18Remote Sensing, Journal Year: 2022, Volume and Issue: 14(23), P. 6104 - 6104
Published: Dec. 1, 2022
Despite their importance to ecosystem services, wetlands are threatened by pollution and development. Over the last few decades, a growing number of wetland studies employed remote sensing (RS) scientifically monitor status support sustainability. Considering rapid evolution significant progress that has been made in field, this paper constitutes an overview utilizing RS methods monitoring. It investigates publications from 1990 up middle 2022, providing systematic survey on data type, machine learning (ML) tools, publication details (e.g., authors, affiliations, citations, date), case studies, accuracy metrics, other parameters interest for RS-based covering 344 papers. The ML combination is deemed helpful monitoring multi-proxy it may open new perspectives research studies. In rapidly changing landscape, integrating multiple types algorithms opportunity advance science management decisions. This provides insight into selection suitable detailed wetland-associated systems. synthesized findings essential determining best practices environmental management, restoration, conservation wetlands. meta-analysis establishes avenues future outlines baseline framework facilitate further scientific using latest state-of-art tools processing data. Overall, present work recommends sustainability requires special land-use policy relevant protocols, regulation, and/or legislation.
Language: Английский
Citations
26Applied Geography, Journal Year: 2022, Volume and Issue: 151, P. 102868 - 102868
Published: Dec. 30, 2022
Language: Английский
Citations
23Water, Journal Year: 2023, Volume and Issue: 15(5), P. 854 - 854
Published: Feb. 22, 2023
Wetlands are highly productive ecosystems with the capability of carbon sequestration, providing an effective solution for climate change. Recent advancements in remote sensing have improved accuracy mapping wetland types, but there remain challenges accurate and automatic mapping, additional requirements complex input data a number types natural habitats. Here, we propose approach using Google Earth Engine (GEE) to automate extraction water bodies growing lotus, type high economic cultural values central Vietnam. Sentinel-1 was used K-Means clustering, whilst Sentinel-2 combined machine learning smile Random Forest (sRF) Gradient Tree Boosting (sGTB) models map areas lotus. The derived from S-1 images confidence (F1 = 0.97 Kappa coefficient 0.94). sGTB outperformed sRF model deliver growth (overall 0.95, 0.92, Precision 0.93, F1 0.93). total lotus area estimated at 145 ha distributed low land study site. Our proposed framework is simple reliable technique, has scalable potential GEE, capable extension other large-scale worldwide.
Language: Английский
Citations
16Discover Geoscience, Journal Year: 2024, Volume and Issue: 2(1)
Published: Oct. 2, 2024
Abstract Effective River system management is essential for conserving water resources, improving agricultural productivity, and sustaining ecological health. Remote sensing crucial evaluating tracking several elements of river systems. The study explores the incorporation remote into Geographic Information Systems (GIS) Artificial Intelligence (AI) to acquire a thorough comprehension dynamics accurately record minor fluctuations in conditions. demonstrates utilization satellite series such as Landsat, Sentinel enhance monitoring methods through analysis high-resolution imagery data. AI helps by automating data processing, finding patterns, making predictions about conditions trends. Machine learning techniques analytical capabilities GIS classifying land cover, predicting flood events, quality. research highlights novel approaches utilizing tackle issues related accessibility, analysis, verification. also acknowledges specific constraints difficulties, concerns over accessibility data, intricacies processes involved validation. statement underscores importance ongoing research, technical progress, collaboration among stakeholders overcome these limitations fully exploit sensing, artificial intelligence, geographic information An integrated approach development successful policies strategies that improve resilience sustainable This eventually promotes resource practices preservation.
Language: Английский
Citations
5