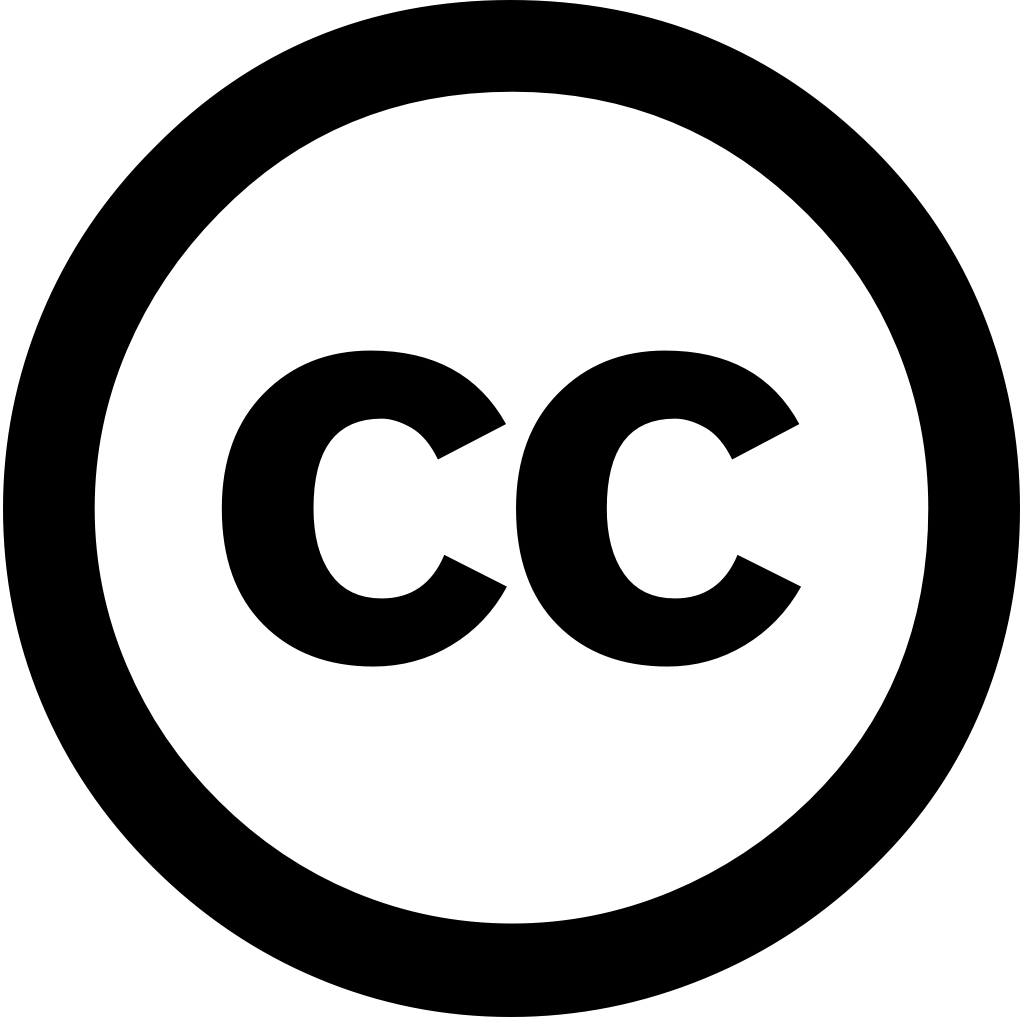
Research Square (Research Square), Journal Year: 2023, Volume and Issue: unknown
Published: Dec. 13, 2023
Language: Английский
Research Square (Research Square), Journal Year: 2023, Volume and Issue: unknown
Published: Dec. 13, 2023
Language: Английский
Computers and Electronics in Agriculture, Journal Year: 2024, Volume and Issue: 221, P. 109017 - 109017
Published: May 7, 2024
Language: Английский
Citations
11Ecological Indicators, Journal Year: 2023, Volume and Issue: 149, P. 110168 - 110168
Published: March 27, 2023
Climate change has caused inland wetlands shrinkage and exacerbated problems, such as sustainable development ecological security, for years. These issues are mainly pronounced in the arid area. The environment's deterioration is especially severe drylands of interior. However, dryland wetland changes their response to climate poorly understood. This study uses K-means algorithm Google Earth Engine (GEE) classify two typical (Ebinur Bosten Lakes) rapidly accurately detecting changes. Moreover, it explores long-term spatial–temporal variation distribution. In addition, investigates various lakes northern southern Xinjiang using wavelet analysis. study's results showed that clustering GEE platform a high classification accuracy (Kappa > 0.8) classification, making feasible approach. terminal lake types, represented by Ebinur Lake, changed significantly between 2001 2021. contrast, inflow-outflow perform more consistently. Significant observed at with gradually shrinking transforming into marsh, where largest marsh proportion degrades non-wetland during year. Lake experienced frequent conversions throughout Furthermore, responses consistent, low precedes precipitation follows evapotranspiration. sensitivity varies, being most affected change. Mastering dynamic achieves goals drylands, including carbon neutrality peak dioxide emissions.
Language: Английский
Citations
19Remote Sensing, Journal Year: 2023, Volume and Issue: 15(21), P. 5254 - 5254
Published: Nov. 6, 2023
Soil salinization seriously affects the sustainable development of agricultural production; thus, timely, efficient, and accurate estimation soil salt content (SSC) has important research significance. In this study, feasibility retrieval using machine learning models was explored based on a UAV (unmanned aerial vehicle) multi-spectral remote sensing platform. First, two variable screening methods (Pearson correlation analysis Grey relational analysis) are used to screen characteristic importance 20 commonly spectral indices. Then, sensitive variables were divided into vegetation index group, combination which represent model. To estimate SSC information for depths 0–20 cm 20–40 cm, three regression constructed: Support Vector Machine (SVM), Random Forest (RF), Backpropagation Neural Network (BPNN). Finally, distribution map depth drawn best The results experiments show that GRA is better than PCA in improving accuracy model, group containing moisture performs best. have achieved good prediction effects some extent. stability model considered comprehensively, effect higher validation set coefficient determination (R2), Root-Mean-Square-Error (RMSE), Mean Absolute Error (MAE) inversion 0.775, 0.055, 0.038, spatial optimal can reflect study area. Therefore, shows platform combined with monitor farmland content.
Language: Английский
Citations
11Environmental Monitoring and Assessment, Journal Year: 2025, Volume and Issue: 197(2)
Published: Jan. 10, 2025
This study investigated the distribution of salinity and sodicity in irrigated areas Abaya Chamo. Representative water soil samples were collected from different depths (0–30 cm 30–60 cm). Sodium absorption ratio (SAR), electrical conductivity (Ec), pH, exchange sodium, magnesium, calcium, potassium cations, sodium percentage (ESP) sampled sites analyzed for classification severity analysis. The spatial analysis revealed predominant strongly saline (72%) sodic (71.1%) conditions surface layer cm), intensifying very (78.7%) (71.9%) at depth. Combined saline-sodic dominated both layers, increasing 91.2% to 97.1% deeper layer, indicating severe agricultural limitations. results show that catchments Lakes Chamo suffer content classes. Therefore, appropriate remedial measures based on should be developed achieve better reclamation, production, land sustainability.
Language: Английский
Citations
0Frontiers in Environmental Science, Journal Year: 2025, Volume and Issue: 13
Published: March 24, 2025
Rapid and accurate soil salinity (SS) analysis is essential for effective management of salinized agricultural lands. However, the potential utilizing periodic remote sensing satellite data to improve accuracy regional SS inversion requires further exploration. This study proposes a novel approach that combines multi-temporal images captured near field sampling period (September 5–10, 2020). Focusing on Wudi County, China, we analyzed three time-series Sentinel-2 obtained determine time window. Images within window were synthesized into four combined-temporal through arithmetic operation strategies one band combination strategy. SS-related spectral variables derived from both single selected using Random Forest (RF), ReliefF, Support Vector Machine Recursive Feature Elimination algorithms (SVM-RFE). Subsequently, models developed compared an Extreme Learning Machine. The optimal model was then applied map distribution. results demonstrate that: (1) consistently outperformed single-temporal models, particularly those employing strategy, showing 0.25–0.53 higher mean Relative Percentage Deviation (RPD); (2) RF variable selection exhibited superior stability efficiency, with RPD 0.02 0.04 than other algorithms; (3) ELM image achieved highest validation precision (Coefficient Determination = 0.72, Root Mean Square Error 0.87 dS/m, 1.93); (4) final revealed spatial gradient increasing in farmland southwestern area toward northeastern coastal region, 46.7% exhibiting yield-affecting levels. These findings provide empirical insights development techniques supporting agricultural-environmental strategies.
Language: Английский
Citations
0Sustainability, Journal Year: 2025, Volume and Issue: 17(9), P. 3779 - 3779
Published: April 22, 2025
The snow water equivalent (SWE) in high-altitude regions is crucial for resource management and disaster risk reduction, yet accurate predictions remain challenging due to complex snowmelt processes, nonlinear meteorological factors, time-lag effects. This study used remote sensing products from the Advanced Microwave Scanning Radiometer (AMSR) as predictand evaluating SWE predictions. It applied nine machine learning models—linear regression (LR), decision trees (DT), support vector (SVR), random forest (RF), artificial neural networks (ANNs), AdaBoost, XGBoost, gradient boosting (GBDT), CatBoost. For each model, submodels were constructed predict next 1 30 days. of model formed prediction over Through an accuracy evaluation ensemble forecasting, days Yalong River above Ganzi Basin was finally achieved. results showed that all models, average Nash–Sutcliffe Efficiency (NSE) rate greater than 0.8, root mean square error (RMSE) under 8 mm, relative (RE) below 7% across three lead time periods (1–10, 11–20, 21–30 days). combining ANNs, GBDT, CatBoost, demonstrated superior accuracy, with NSE values exceeding 0.85 RMSE 6 mm. A sensitivity analysis using Shapley Additive Explanations (SHAP) revealed temperature variables (average, minimum, maximum temperatures) most influential while humidity (Rhu) significantly affected by reducing evaporation. These findings provide insights improving regions.
Language: Английский
Citations
0GEOMATICA, Journal Year: 2024, Volume and Issue: 76(2), P. 100023 - 100023
Published: Sept. 3, 2024
Earth-observing satellites have become essential in comprehending human impacts on the landscape. Satellite-based imagery is indispensable for mapping Earth's features, managing resources, and studying environmental changes. Readily available remote sensing data with improved radiometric, spectral, spatial, temporal resolution presents opportunities advanced analysis. Precise accurate land use cover (LULC) information surveillance of conditions effective management natural resources. This research assesses performance PlanetScope product SuperDove sensor (PSB.SD), having two different band combinations, including 4-band (Red, Blue, Green Near-Infrared (NIR)) 8-band (Blue, II, Red, NIR, Coastal Green, Yellow, Red-Edge) ArcGIS Pro month July 2021. Four supervised classifiers, support vector machine (SVM), k-nearest neighbours (KNN), random forest (RF), maximum likelihood (ML) classifiers. study was carried out three major areas, i.e., City Summerside, Charlottetown, Town Three Rivers Prince Edward Island (PEI), Canada LULC classification scheme consists six classes, which include Agriculture, Forest, Vegetation, Bare Land, Urban Water bodies. For accuracy assessment, overall as well kappa coefficient were estimated to identify most combination classifier from PlanetScope. Results show that highest 0.94 0.93 Summerside Charlottetown observed using SVM classifier. The lowest 0.78 Rivers, 0.83 0.82 ML Further, performs PlanetScope, showcasing its potential compared previous imagery.
Language: Английский
Citations
3Land, Journal Year: 2023, Volume and Issue: 12(9), P. 1680 - 1680
Published: Aug. 28, 2023
A comprehensive understanding of soil salinity distribution in arid regions is essential for making informed decisions regarding agricultural suitability, water resource management, and land use planning. methodology was developed to identify Sudan by utilizing optical radar-based satellite data as well variables obtained from digital elevation models that are known indicate variations salinity. The includes the transfer areas where similar conditions prevail. geographically coordinated database established, incorporating a variety environmental based on Google Earth Engine (GEE) Electrical Conductivity (EC) measurements saturation extract samples collected at three different depths (0–30, 30–60, 60–90 cm). Thereafter, Multinomial Logistic Regression (MNLR) Gradient Boosting Algorithm (GBM), were utilized spatially classify levels region. To determine applicability model trained reference site target area, Multivariate Environmental Similarity Surface (MESS) analysis conducted. producer’s accuracy, user’s Tau index parameters used evaluate model’s spatial confusion indices computed assess uncertainty. At depths, values area ranged 0.38 0.77, whereas 0.66 0.88, decreasing depth increased. Clay normalized ratio (CLNR), Salinity Index 1, SAR important modeling. It found subsoils middle northwest both had higher level compared topsoil. This study highlighted effectiveness means identifying evaluating management facing significant salinity-related challenges. approach can be instrumental alternative suitable activities regional level.
Language: Английский
Citations
9Agronomy, Journal Year: 2024, Volume and Issue: 14(4), P. 693 - 693
Published: March 27, 2024
One of the challenges in site-specific phosphorus (P) management is substantial spatial variability plant available P across fields. To overcome this barrier, emerging sensing, data fusion, and predictive modeling approaches are needed to accurately reveal heterogeneity P. Seven spatially variable fields located Ontario, Canada clustered into two zones; four eastern Ontario three others western Ontario. This study compares Bayesian Additive Regression Trees (BART), Support Vector Machine regressor (SVM), Ordinary Kriging (OK), along with novel fusion concepts, analyze integrated high-density layers related soil Feature selection interaction detection using BART Recursive Elimination (RFE) for SVM were applied 42 predictors, including soil-vegetation indices derived from PlanetScope multispectral imagery, apparent electrical conductivity (ECa), high-resolution topographic attributes DUALEM-21S a Real-Time Kinematic (RTK) global navigation satellite systems (GNSS) receiver, respectively. Modeling showed higher accuracy than OK both zones when trained tested on ground truth clusters farms. A approach resulted six auxiliary predictors zone, while only selected predict zone. RFE models 15 12 zones. Topographic elevation was most influential predictor Compared methods, exhibited lower average RMSE values individual 1.86 ppm 3.58 zones, respectively, R2 0.85 0.83, In contrast, had averaging 5.04 7.51 0.27 0.43. averaged 4.77 7.81 ppm, method, 0.19 0.44. The suitable combined machine learning algorithms, have potential be useful tool estimate patterns agricultural Canada.
Language: Английский
Citations
2Remote Sensing, Journal Year: 2024, Volume and Issue: 16(24), P. 4812 - 4812
Published: Dec. 23, 2024
Soil salinization is a significant global ecological issue that leads to soil degradation and recognized as one of the primary factors hindering sustainable development irrigated farmlands deserts. The integration remote sensing (RS) machine learning algorithms increasingly employed deliver cost-effective, time-efficient, spatially resolved, accurately mapped, uncertainty-quantified salinity information. We reviewed articles published between January 2016 December 2023 on sensing-based prediction synthesized latest research advancements in terms innovation points, data, methodologies, variable importance, trends, current challenges, potential future directions. Our observations indicate innovations this field focus detection depth, iterations data conversion methods, application newly developed sensors. Statistical analysis reveals Landsat most frequently utilized sensor these studies. Furthermore, deep remains underexplored. ranking accuracy across various study areas follows: lake wetland (R2 = 0.81) > oasis 0.76) coastal zone 0.74) farmland 0.71). also examined relationship metadata accuracy: (1) Validation accuracy, sample size, number variables, mean exhibited some correlation with modeling while sampling type, time, maximum did not influence accuracy. (2) Across broad range scales, large sizes may lead error accumulation, which associated geographic diversity area. (3) inclusion additional environmental variables does necessarily enhance (4) Modeling improves when area exceeds 30 dS/m. Topography, vegetation, temperature are relatively covariates. Over past years, affected by has been increasing. To further we provide several suggestions for challenges directions research. While sole solution, it provides unique advantages salinity-related studies at both regional scales.
Language: Английский
Citations
2