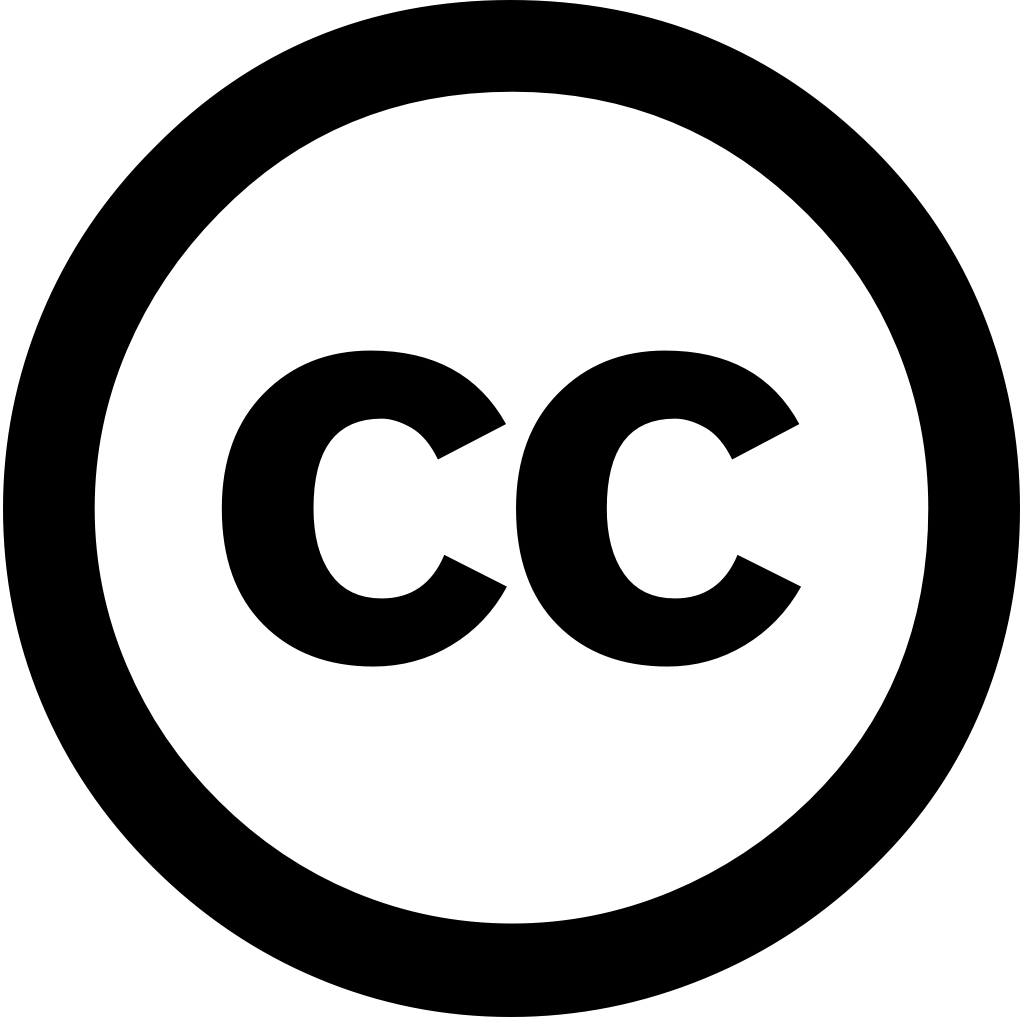
Ecological Informatics, Journal Year: 2024, Volume and Issue: unknown, P. 102945 - 102945
Published: Dec. 1, 2024
Language: Английский
Ecological Informatics, Journal Year: 2024, Volume and Issue: unknown, P. 102945 - 102945
Published: Dec. 1, 2024
Language: Английский
Earth Science Informatics, Journal Year: 2024, Volume and Issue: 17(5), P. 4419 - 4440
Published: July 6, 2024
Abstract Flash floods rank among the most catastrophic natural disasters worldwide, inflicting severe socio-economic, environmental, and human impacts. Consequently, accurately identifying areas at potential risk is of paramount importance. This study investigates efficacy Deep 1D-Convolutional Neural Networks (Deep 1D-CNN) in spatially predicting flash floods, with a specific focus on frequent tropical cyclone-induced Thanh Hoa province, North Central Vietnam. The 1D-CNN was structured four convolutional layers, two pooling one flattened layer, fully connected employing ADAM algorithm for optimization Mean Squared Error (MSE) loss calculation. A geodatabase containing 2540 flood locations 12 influencing factors compiled using multi-source geospatial data. database used to train check model. results indicate that model achieved high predictive accuracy (90.2%), along Kappa value 0.804 an AUC (Area Under Curve) 0.969, surpassing benchmark models such as SVM (Support Vector Machine) LR (Logistic Regression). concludes highly effective tool modeling floods.
Language: Английский
Citations
7Ecological Informatics, Journal Year: 2024, Volume and Issue: 84, P. 102904 - 102904
Published: Nov. 17, 2024
Language: Английский
Citations
6Natural Hazards, Journal Year: 2024, Volume and Issue: unknown
Published: Sept. 2, 2024
Language: Английский
Citations
4Geoscience Frontiers, Journal Year: 2024, Volume and Issue: 16(1), P. 101960 - 101960
Published: Nov. 1, 2024
Language: Английский
Citations
4Journal of Environmental Radioactivity, Journal Year: 2025, Volume and Issue: 282, P. 107619 - 107619
Published: Jan. 17, 2025
Language: Английский
Citations
0Urban Water Journal, Journal Year: 2025, Volume and Issue: unknown, P. 1 - 25
Published: Jan. 20, 2025
Urban pluvial floods are characterised by a number of features such as the high spatial and temporal resolution needed to capture their dynamics, complexity dual drainage systems lack sewer data availability, which make them very different from other types floods, like coastal or fluvial increase difficulty modelling them. As consequence, most flood management plans do not include rigorous evaluation urban risk. In this paper, we give comprehensive view current state modelling, restricted mere description mathematical approaches, but also including relevant that should be considered in validation studies have been performed date our vision on challenges addressed near future.
Language: Английский
Citations
0Remote Sensing, Journal Year: 2025, Volume and Issue: 17(3), P. 422 - 422
Published: Jan. 26, 2025
The main objective of the present study was to develop an integrated approach combining remote sensing techniques and U-Net-based deep learning models for lithology mapping. methodology incorporates Landsat 8 imagery, ALOS PALSAR data, field surveys, complemented by derived products such as False Color Composites (FCCs), Minimum Noise Fraction (MNF), Principal Component Analysis (PCA). Dissection Index, a morphological index, calculated characterize geomorphological variability region. Three variations U-Net architecture, Dense U-Net, Residual Attention were implemented evaluate performance in lithological classification. Validation conducted using metrics accuracy, precision, recall, F1-score, mean intersection over union (mIoU). results highlight effectiveness model, which provided highest mapping accuracy superior feature extraction delineating flysch formations associated units. This demonstrates potential integrating data with advanced machine enhance geological challenging terrains.
Language: Английский
Citations
0Stochastic Environmental Research and Risk Assessment, Journal Year: 2025, Volume and Issue: unknown
Published: Feb. 8, 2025
Language: Английский
Citations
0Engineering Applications of Artificial Intelligence, Journal Year: 2025, Volume and Issue: 150, P. 110616 - 110616
Published: March 23, 2025
Language: Английский
Citations
0Remote Sensing, Journal Year: 2023, Volume and Issue: 15(22), P. 5429 - 5429
Published: Nov. 20, 2023
Fluvial floods endure as one of the most catastrophic weather-induced disasters worldwide, leading to numerous fatalities each year and significantly impacting socio-economic development environment. Hence, research new methods algorithms focused on improving fluvial flood prediction devising robust management strategies are essential. This study explores assesses potential application 1D-Convolution Neural Networks (1D-CNN) for spatial in Quang Nam province, a high-frequency tropical cyclone area central Vietnam. To this end, geospatial database with 4156 locations 12 indicators was considered. The ADAM algorithm MSE loss function were used train 1D-CNN model, whereas popular performance metrics, such Accuracy (Acc), Kappa, AUC, measure performance. results indicated remarkable by achieving high accuracy metrics Acc = 90.7%, Kappa 0.814, AUC 0.963. Notably, proposed model outperformed benchmark models, including DeepNN, SVM, LR. achievement underscores promise innovation brought realm susceptibility mapping floods.
Language: Английский
Citations
10