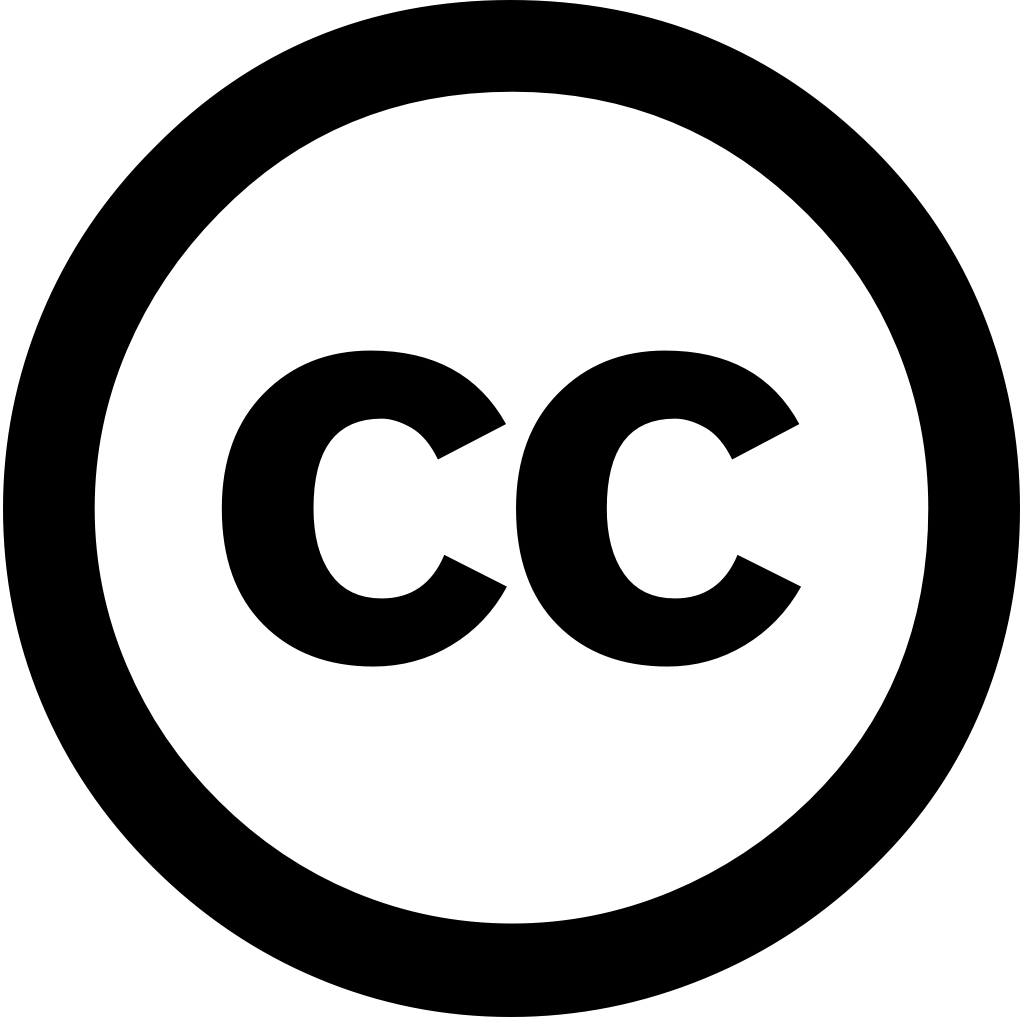
Geomatics Natural Hazards and Risk, Journal Year: 2024, Volume and Issue: 15(1)
Published: May 20, 2024
This study aims to evaluate the effectiveness of various individual machine learning and their ensemble techniques such as Stacking, Voting Meta-learning in landslide susceptibility assessment taking Poyang, Jiangxi, China an example. Multi-source geo-environmental data including field surveys, Sentinel-2A/B satellite images, Digital Elevation Models (DEM), geological hydrological were utilized construct validate models. Results show that Stacking Classifier outperformed other models, achieving highest F1 Score 0.846 AUC (Area Under ROC Curve) 0.923, demonstrating its strong predictivity, followed by with 0.829 0.922. Among Multi-Layer Perceptron (MLP) performed best 0.828 0.904. Furthermore, explainable Artificial Intelligence (XAI) technique was applied better understand mechanism classifiers predicting it suggests a significant correlation between land use, distance fault, occurrences. In conclusion, hybrid models clear advantages over ones for risk zoning. The results may provide technical support disaster mitigation efforts future urban planning areas prone landslides Poyang.
Language: Английский