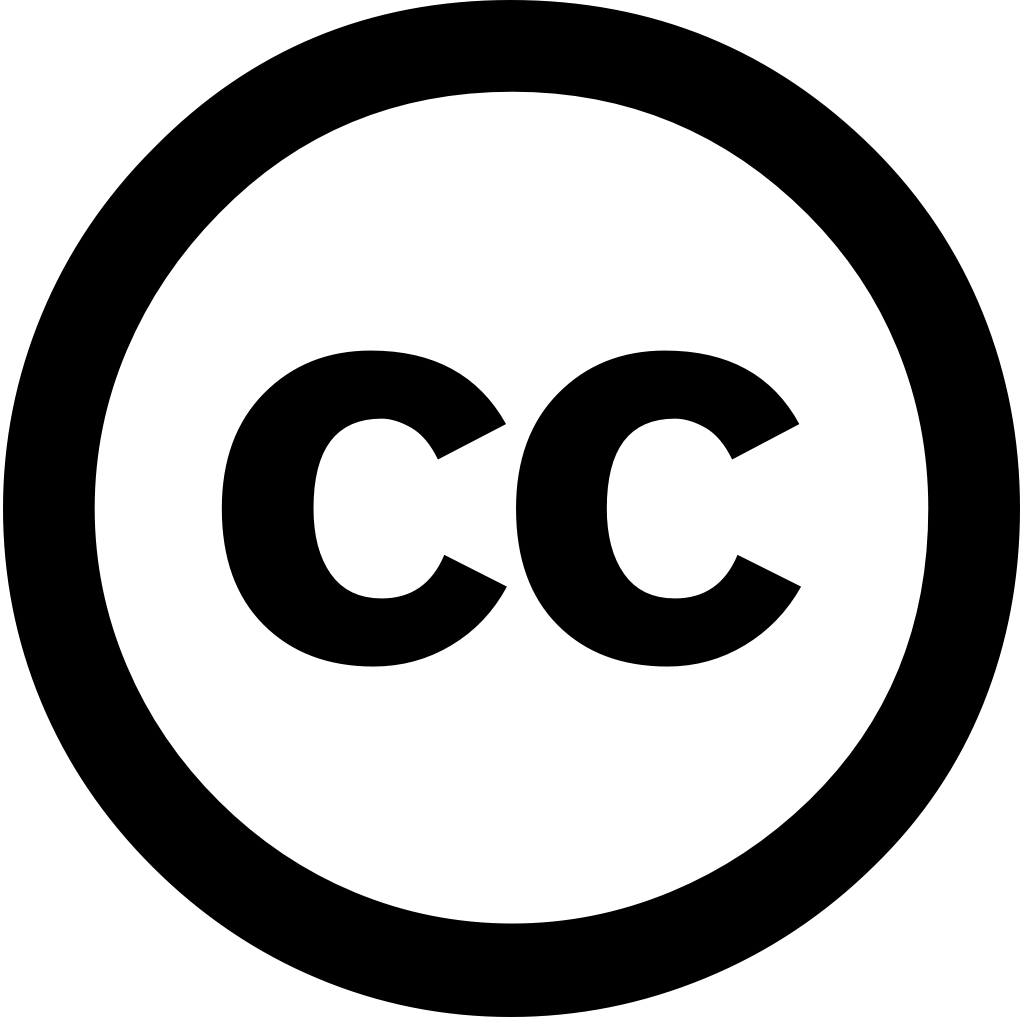
Land, Journal Year: 2024, Volume and Issue: 13(11), P. 1810 - 1810
Published: Nov. 1, 2024
Heavy metal pollution in agricultural land poses significant threats to both the ecological environment and human health. Therefore, rapid accurate prediction of heavy content soil is crucial for environmental protection remediation. Acknowledging limitations traditional single linear or nonlinear machine learning models terms accuracy, this study developed an ensemble model that integrates multiple with a random forest (RF) improve accuracy reliability. In study, we selected typical copper (Cu) polluted area Pearl River Delta Guangdong Province as research site collected Cu data indoor reflectance spectral from 269 surface samples. First, were preprocessed using Savitzky–Golay (SG) smoothing, multiplicative scattering correction (MSC), continuous wavelet transform (CWT) reduce noise interference. Next, principal components analysis (PCA) was employed dimensionality data, eliminating redundant features lowering computational complexity. Finally, based on dimensionality-reduced content, established stacked model, where base included SVR, PLSR, BPNN, XGBoost, RF serving meta-model estimate content. To evaluate performance stacking compared its individual models. The results indicate that, models, superior (R2 = 0.77; RMSE 7.65 mg/kg; RPD 2.29). This suggests integrated algorithm demonstrates greater robustness generalization capability. presents method estimation hyperspectral technology, ensuring robust supports policymakers making informed decisions about use, agriculture, protection.
Language: Английский