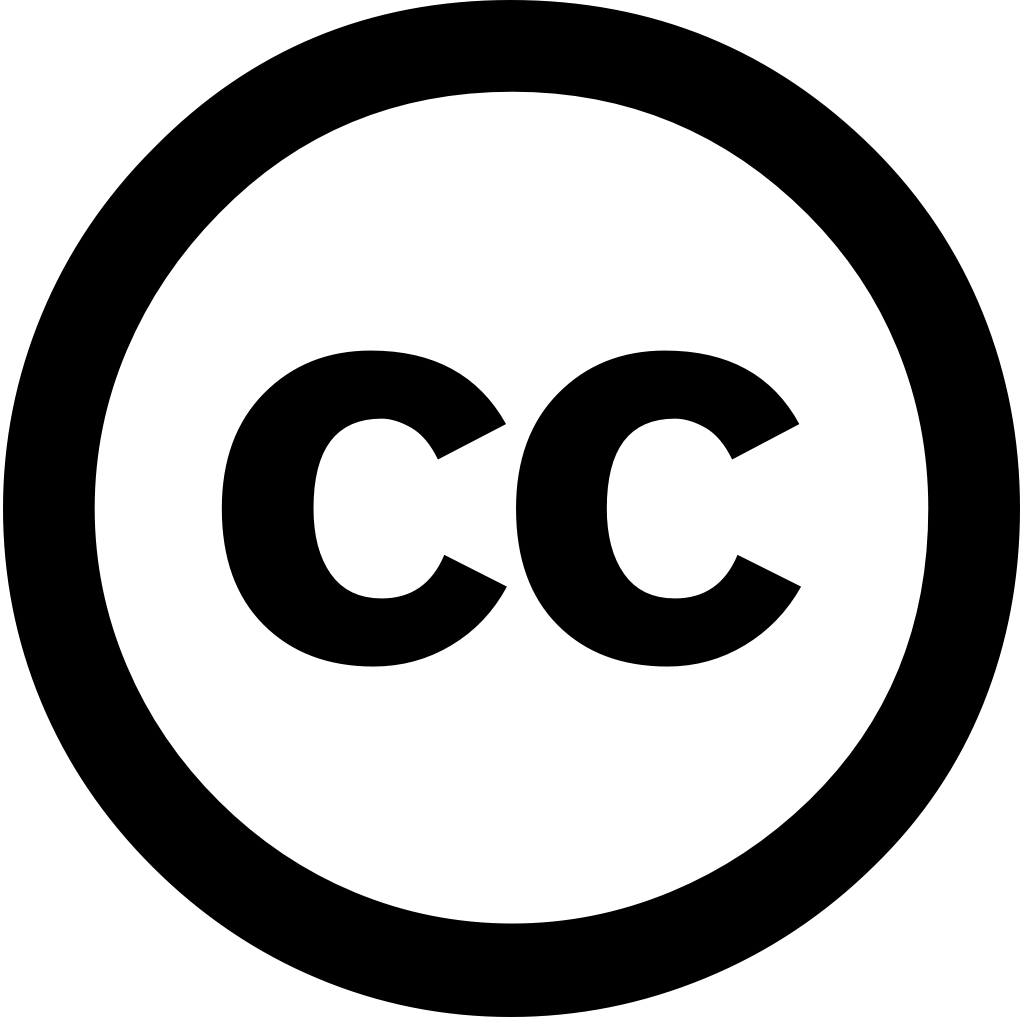
Research Square (Research Square), Journal Year: 2024, Volume and Issue: unknown
Published: Aug. 18, 2024
Language: Английский
Research Square (Research Square), Journal Year: 2024, Volume and Issue: unknown
Published: Aug. 18, 2024
Language: Английский
Environmental Technology & Innovation, Journal Year: 2024, Volume and Issue: 35, P. 103655 - 103655
Published: May 5, 2024
Forest fires pose a significant threat to ecosystems and socio-economic activities, necessitating the development of accurate predictive models for effective management mitigation. In this study, we present novel machine learning approach combined with Explainable Artificial Intelligence (XAI) techniques predict forest fire susceptibility in Nainital district. Our innovative methodology integrates several robust — AdaBoost, Gradient Boosting Machine (GBM), XGBoost Random Deep Neural Network (DNN) as meta-model stacking framework. This not only utilises individual strengths these models, but also improves overall prediction performance reliability. By using XAI techniques, particular SHAP (SHapley Additive exPlanations) LIME (Local Interpretable Model-agnostic Explanations), improve interpretability provide insights into decision-making processes. results show effectiveness ensemble model categorising different zones: very low, moderate, high high. particular, identified extensive areas susceptibility, precision, recall F1 values underpinning their effectiveness. These achieved ROC AUC above 0.90, performing exceptionally well an 0.94. The are remarkably inclusion confidence intervals most important metrics all emphasises robustness reliability supports practical use management. Through summary plots, analyze global variable importance, revealing annual rainfall Evapotranspiration (ET) key factors influencing susceptibility. Local analysis consistently highlights importance rainfall, ET, distance from roads across models. study fills research gap by providing comprehensive interpretable modelling that our ability effectively manage risk is consistent environmental protection sustainable goals.
Language: Английский
Citations
17Water, Journal Year: 2025, Volume and Issue: 17(2), P. 167 - 167
Published: Jan. 10, 2025
Landslide susceptibility mapping (LSM) is crucial for disaster prevention in large, complex regions characterized by high-dimensional data. This study proposes a Feature-Selecting Long Short-Term Memory (FS-LSTM) framework to enhance LSM accuracy integrating feature selection techniques with sequence-based modeling. The Mean Decrease Impurity (MDI) and Information Gain Ratio (IGR) were used rank landslide conditioning factors (LCFs), these rankings structured FS-LSTM inputs assess the impact of ordering on model performance. Feature-ordering experiments demonstrated that significantly improve compared randomized inputs. Our outperformed traditional machine learning algorithms, such as logistic regression Support Vector Machine, well standard deep models like CNN basic LSTM, achieving score 0.988. MDI IGR consistently identified soil type, elevation, average annual cumulated rainfall most influential LCFs, improving interpretability results. Applied Yangtze River Basin, effectively landslide-prone areas, aligning known geological patterns. These findings highlight potential combining sequence-sensitive robustness LSM. Future studies could expand this approach other incorporate real-time monitoring systems dynamic management.
Language: Английский
Citations
1Land, Journal Year: 2025, Volume and Issue: 14(1), P. 172 - 172
Published: Jan. 15, 2025
The effectiveness of data-driven landslide susceptibility mapping relies on data integrity and advanced geospatial analysis; however, selecting the most suitable method identifying key regional factors remains a challenging task. To address this, this study assessed performance six machine learning models, including Convolutional Neural Networks (CNNs), Random Forest (RF), Categorical Boosting (CatBoost), their CNN-based hybrid models (CNN+RF CNN+CatBoost), Stacking Ensemble (SE) combining CNN, RF, CatBoost in along Karakoram Highway northern Pakistan. Twelve were examined, categorized into Topography/Geomorphology, Land Cover/Vegetation, Geology, Hydrology, Anthropogenic Influence. A detailed inventory 272 occurrences was compiled to train models. proposed stacking ensemble improve modeling, with achieving an AUC 0.91. Hybrid modeling enhances accuracy, CNN–RF boosting RF’s from 0.85 0.89 CNN–CatBoost increasing CatBoost’s 0.87 0.90. Chi-square (χ2) values (9.8–21.2) p-values (<0.005) confirm statistical significance across This identifies approximately 20.70% area as high very risk, SE model excelling detecting high-risk zones. Key influencing showed slight variations while multicollinearity among variables remained minimal. approach reduces uncertainties, prediction supports decision-makers implementing effective mitigation strategies.
Language: Английский
Citations
1Landslides, Journal Year: 2025, Volume and Issue: unknown
Published: Feb. 13, 2025
Language: Английский
Citations
1Land, Journal Year: 2024, Volume and Issue: 13(7), P. 1011 - 1011
Published: July 8, 2024
The reliability of data-driven approaches in generating landslide susceptibility maps depends on data quality, analytical method selection, and sampling techniques. Selecting optimal datasets determining the most effective methods pose significant challenges. This study assesses performance seven machine learning classifiers Himalayan region China–Pakistan Economic Corridor, utilizing statistical techniques validation metrics. Thirteen geo-environmental variables were analyzed, including topographic (8), land cover (1), hydrological geological (2), meteorological (1) factors. These evaluated for multicollinearity, feature importance, their influence incidences. Our findings indicate that Support Vector Machines Logistic Regression highly effective, particularly near fault zones roads, due to effectiveness handling complex, non-linear terrain interactions. Conversely, Random Forest demonstrated variability results. Each model distinctly identified ranging from very low high risk. Significant conditioning such as elevation, rainfall, lithology, slope, use identified, reflecting unique geomorphological conditions Himalayas. Further analysis using Variance Inflation Factor Pearson correlation coefficient showed minimal multicollinearity among variables. Moreover, evaluations Area Under Receiver Operating Characteristic Curve (AUC-ROC) values confirmed strong predictive capabilities models, with Classifier performing exceptionally well, achieving an AUC 0.96 F-Score 0.86. shows importance selection based dataset characteristics enhance decision-making strategy effectiveness.
Language: Английский
Citations
5Boreas, Journal Year: 2024, Volume and Issue: unknown
Published: Nov. 27, 2024
Subglacial processes exert a major control on ice streaming. Constraining subglacial conditions thus allows for more accurate predictions of mass loss. Due to the difficulty in observing large‐scale modern environment, we turn geological records streaming deglaciated environments. Morphometric values streamlined bedforms provide valuable information about relative speed, direction, and maturity past streams relationship between erosion deposition. However, manually identifying across landscapes, sometimes clusters several thousand, is an arduous task with difficult‐to‐control sources variability human‐biased errors. This paper presents new tool that utilizes machine learning approach automatically identify glacially derived features. Slope variations landscape, identified by topographic position index, undergo analysis from series supervised models trained over 600 000 data points Northern Hemisphere. A filtered set produced through combination scientifically driven preprocessing statistical downsampling improved robustness our approach. After cross‐validation, found Random Forest detected most true positives, up 94.5% withheld test set, ensemble average provided highest stability when applied within range applicable sets, performing at 79% identification positives out distribution area interest. We build these into open‐source Python package, bedfinder, apply it Green Bay Lobe region, USA, finding general ice‐flow direction bedform elongation minimal effort. type open, reproducible leading edge glacial geomorphology research will continue improve integration newly acquired previously collected data.
Language: Английский
Citations
4Energy, Journal Year: 2025, Volume and Issue: unknown, P. 134974 - 134974
Published: Feb. 1, 2025
Language: Английский
Citations
0Earth Science Informatics, Journal Year: 2025, Volume and Issue: 18(4)
Published: March 22, 2025
Language: Английский
Citations
0Electronics, Journal Year: 2025, Volume and Issue: 14(7), P. 1409 - 1409
Published: March 31, 2025
With more attention paid to the prevention of cardiovascular diseases, convenient and non-invasive methods blood pressure measurement are gradually receiving attention. Non-invasive based on pulse wave signals is simple fast but requires specialized medical knowledge deal with complex features. The aim this study was map signal features systolic/diastolic values using machine learning methods. In study, a flexible piezoelectric sensor its circuit were designed measure preprocess signals. Then, 32 extracted from time domain, frequency domain wavelet random forest regression model introduced estimate diastolic/systolic pressure. Finally, optimization effect evaluation carried out. mean absolute errors systolic diastolic pressures estimated by proposed system within 1.72 mmHg 1.40 mmHg, which meets requirement Association for Advancement Medical Instrumentation error below 5 mmHg. expected enable daily monitoring applications.
Language: Английский
Citations
0Bulletin of Engineering Geology and the Environment, Journal Year: 2025, Volume and Issue: 84(5)
Published: April 28, 2025
Language: Английский
Citations
0