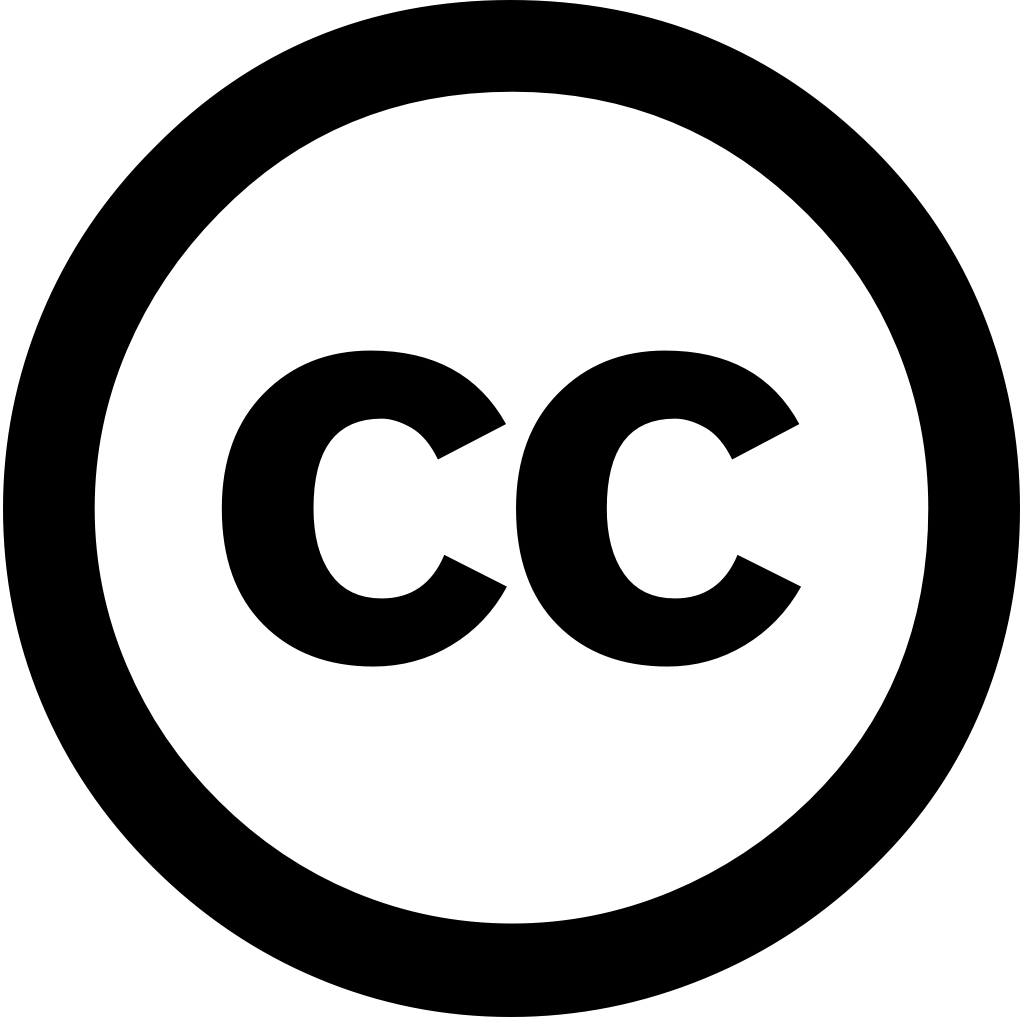
Alexandria Engineering Journal, Journal Year: 2024, Volume and Issue: 110, P. 153 - 167
Published: Oct. 8, 2024
Language: Английский
Alexandria Engineering Journal, Journal Year: 2024, Volume and Issue: 110, P. 153 - 167
Published: Oct. 8, 2024
Language: Английский
Applied Sciences, Journal Year: 2025, Volume and Issue: 15(5), P. 2747 - 2747
Published: March 4, 2025
Soil salinization is a significant threat to agricultural production, making accurate salinity prediction essential. This study addresses key challenges in the Yellow River Delta (YRD) soil inversion, including (1) determining which Landsat 8 OLI level performs better, (2) identifying most suitable month for and (3) improving model performance important variables modeling. Thus images (Level-1 Level-2) 12 months were collected, then having less than 10% cloud cover selected processed extract spectral values. A total of 86 sampled points measure salinity. Using Pearson correlation expert insights, January 15 August 26 identified as dates inversion. Then, seven original bands, 29 indicators, 39 derived created through six mathematical transformations, used construct following three models: partial least squares regression (PLSR), random forest (RF), backpropagation neural network (BPNN). The results showed following: Level-1 data, after FLAASH atmospheric correction, outperforms Level-2 data. optimal Among models, RF outperformed others, achieving test set R2 = 0.55, RMSE 3.4, suggesting that combination indicators mathematically transformed can effectively enhance accuracy predicting YRD. Furthermore, SWIR1, SWIR2, CLEX, second-order difference first-order SWIR2 along with NIR played role
Language: Английский
Citations
1Remote Sensing, Journal Year: 2024, Volume and Issue: 16(14), P. 2681 - 2681
Published: July 22, 2024
Salinization is a major soil degradation process threatening ecosystems and posing great challenge to sustainable agriculture food security worldwide. This study aimed evaluate the potential of state-of-the-art machine learning algorithms in salinity (EC1:5) mapping. Further, we predicted distribution patterns under different future scenarios Yellow River Delta. A geodatabase comprising 201 samples 19 conditioning factors (containing data based on remote sensing images such as Landsat, SPOT/VEGETATION PROBA-V, SRTMDEMUTM, Sentinel-1, Sentinel-2) was used compare predictive performance empirical bayesian kriging regression, random forest, CatBoost models. The model exhibited highest with both training testing datasets, an average MAE 1.86, RMSE 3.11, R2 0.59 datasets. Among explanatory factors, Na most important for predicting EC1:5, followed by normalized difference vegetation index organic carbon. Soil EC1:5 predictions suggested that Delta region faces severe salinization, particularly coastal zones. three increases carbon content (1, 2, 3 g/kg), 2 g/kg scenario resulted best improvement effect saline–alkali soils > ds/m. Our results provide valuable insights policymakers improve land quality plan regional agricultural development.
Language: Английский
Citations
5Journal of Marine Science and Application, Journal Year: 2024, Volume and Issue: unknown
Published: Oct. 2, 2024
Language: Английский
Citations
4Scientific Reports, Journal Year: 2024, Volume and Issue: 14(1)
Published: Oct. 25, 2024
Oral Squamous Cell Carcinoma (OSCC) causes a severe challenge in oncology due to the lack of diagnostic devices, leading delays detecting disorder. The OSCC diagnosis through histopathology demands pathologist expert because cellular presentation is variable and highly complex. Existing approaches for have specific efficiency accuracy restrictions, highlighting necessity more reliable techniques. increase deep neural networks (DNN) model their applications medical imaging been instrumental disease detection. Automatic detection systems using learning (DL) show tremendous promise investigating imagery with speed, efficiency, accuracy. In terms OSCC, this system allows method be streamlined, facilitating earlier enhancing survival rates. analysis histopathological image (HI) can assist accurately identifying tumorous tissue, reducing turnaround times increasing efficacy pathologists. This study presents Squeeze-Excitation Hybrid Deep Learning Recognition (SEHDL-OSCCR) on HIs. presented SEHDL-OSCCR technique mainly focuses oral cancer (OC) hybrid DL models. bilateral filtering (BF) initially used remove noise. Next, employs SE-CapsNet recognize feature extractors. An improved crayfish optimization algorithm (ICOA) utilized improve performance model. At last, classification performed by employing convolutional network bidirectional long short-term memory (CNN-BiLSTM) simulation results obtained are investigated benchmark dataset. experimental validation illustrated greater outcome 98.75% compared recent approaches.
Language: Английский
Citations
2Published: Jan. 1, 2024
Language: Английский
Citations
0Biomedical Signal Processing and Control, Journal Year: 2024, Volume and Issue: 99, P. 106810 - 106810
Published: Sept. 12, 2024
Language: Английский
Citations
0Alexandria Engineering Journal, Journal Year: 2024, Volume and Issue: 110, P. 153 - 167
Published: Oct. 8, 2024
Language: Английский
Citations
0