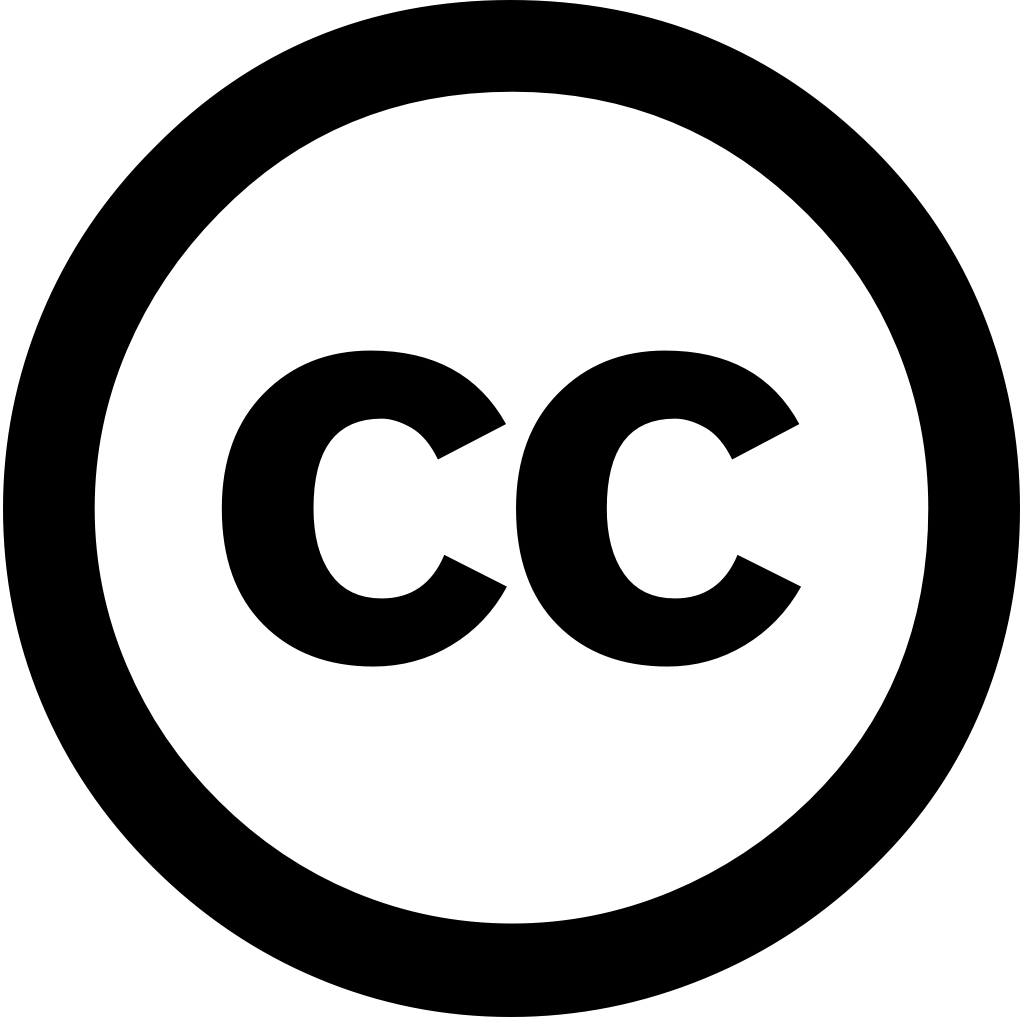
Frontiers in Environmental Science, Journal Year: 2025, Volume and Issue: 13
Published: April 30, 2025
Water catchment areas are the key strategic water sources with a variety of ecological benefits. However, trajectory Land Cover and Use Changes (LULC-C change poses significant threat to areas, negatively affecting quality. Thus, adoption remote sensing data Machine Learning Algorithms (MLAs) is novel approach that provides spatiotemporal on environmental changes resulting from LULC dynamics. Hence, this work harnessed Landsat imageries Random Forests (RF) classification as well hybrid model Multi-Layer Perceptron Markov chain (MLPNN-Markov) detect in forecast future changes. At every 5 years interval, RF generated more accurate maps for 2003–2023. The prediction 2019 also produced acceptable values kappa accuracy matrices, which were 65.50%, 58.4%, 90.90%, 0.52 overall accuracy, location, histogram, overall, respectively. findings highlighted decline forest strong negative correlation built-up mining areas. secondary invasion abandoned cropland occupied by grassland members was observed. displayed increasing trends between 2023. Wetlands water, however, exhibited steady trend minor variations. On other hand, each these persisted future, exception scaling-down behaviour 2032. outcomes will offer piece updated information LULC-C hints at possible direction This crucial local bodies tasked protect integrity aim improving
Language: Английский