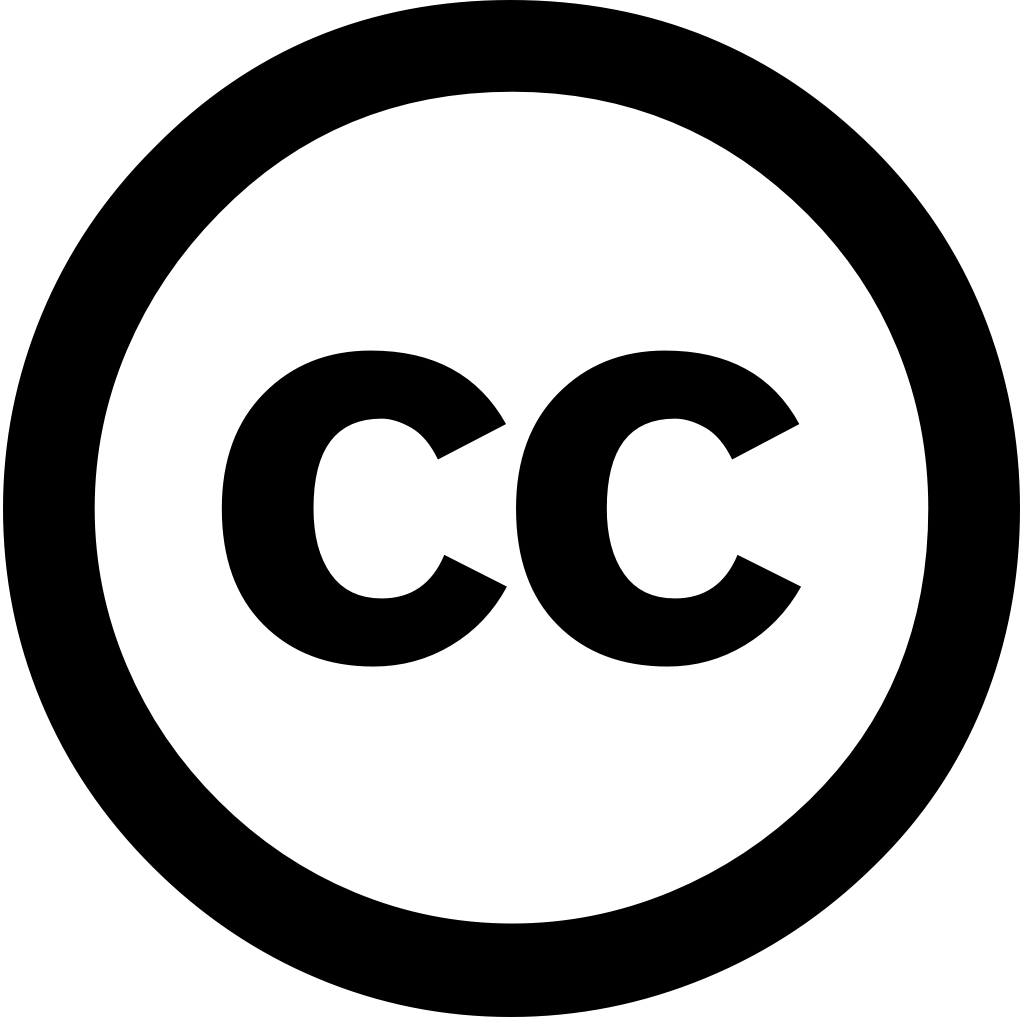
Ore Geology Reviews, Journal Year: 2024, Volume and Issue: 176, P. 106396 - 106396
Published: Dec. 13, 2024
Language: Английский
Ore Geology Reviews, Journal Year: 2024, Volume and Issue: 176, P. 106396 - 106396
Published: Dec. 13, 2024
Language: Английский
Land, Journal Year: 2025, Volume and Issue: 14(1), P. 172 - 172
Published: Jan. 15, 2025
The effectiveness of data-driven landslide susceptibility mapping relies on data integrity and advanced geospatial analysis; however, selecting the most suitable method identifying key regional factors remains a challenging task. To address this, this study assessed performance six machine learning models, including Convolutional Neural Networks (CNNs), Random Forest (RF), Categorical Boosting (CatBoost), their CNN-based hybrid models (CNN+RF CNN+CatBoost), Stacking Ensemble (SE) combining CNN, RF, CatBoost in along Karakoram Highway northern Pakistan. Twelve were examined, categorized into Topography/Geomorphology, Land Cover/Vegetation, Geology, Hydrology, Anthropogenic Influence. A detailed inventory 272 occurrences was compiled to train models. proposed stacking ensemble improve modeling, with achieving an AUC 0.91. Hybrid modeling enhances accuracy, CNN–RF boosting RF’s from 0.85 0.89 CNN–CatBoost increasing CatBoost’s 0.87 0.90. Chi-square (χ2) values (9.8–21.2) p-values (<0.005) confirm statistical significance across This identifies approximately 20.70% area as high very risk, SE model excelling detecting high-risk zones. Key influencing showed slight variations while multicollinearity among variables remained minimal. approach reduces uncertainties, prediction supports decision-makers implementing effective mitigation strategies.
Language: Английский
Citations
1Remote Sensing, Journal Year: 2025, Volume and Issue: 17(2), P. 339 - 339
Published: Jan. 20, 2025
Landslide susceptibility evaluation is an indispensable part of disaster prevention and mitigation work. Selecting effective methods models for landslide assessment significant importance. This study focuses on selected areas in Yunyang County, Chongqing City. By interpreting high-resolution satellite remote sensing images from before after heavy rainfall 31 August 2014, the distribution rainfall-induced accumulation landslides was obtained. To evaluate landslides, we have equated factors to prediction factors. Eight were extracted using multi-source data, including lithology, elevation, slope, image texture features, normalized difference vegetation index (NDVI). Various machine learning models, such as Random Forest (RF), Support Vector Machine (SVM), BP Neural Network employed assess area. Subsequently, accuracy compared verified Receiver Operating Characteristic (ROC) curve, results analyzed. Finally, developed model applied Gongping Town Fengjie County verify its applicability other regions. The findings indicate that complex geological conditions unique tectonic erosion landform patterns northeastern region not only make this area a center but also lead frequent recurrent landslides. effectively reflects development characteristics High very high zones are concentrated northern central regions area, while low moderate predominantly occupy mountainous riverside areas. mapping shows yields reasonably graded results. Elevation, lithology highly system, indicating slope disasters mainly related topography, geomorphology, lithology. Town, validate
Language: Английский
Citations
0Remote Sensing, Journal Year: 2025, Volume and Issue: 17(6), P. 973 - 973
Published: March 10, 2025
The enhancement of remote sensing interpretation accuracy for rock strata in complex terrain areas has long been limited by challenges field validation and the insufficient integration geological knowledge traditional spectral–spatial feature selection methods. This study proposes a framework that integrates textual data, which enhances lithological identification systematically combining multi-source with machine learning algorithms. Using dataset 2591 survey reports scientific literature, ontology model was established, featuring four core entities (rock type, stratigraphic unit, spectral feature, geomorphological indicator). A hybrid information extraction process rule-based parsing fine-tuned Universal Information Extraction (UIE) employed to extract from unstructured texts. graph constructed using TransE algorithm consists 766 entity nodes 1008 relationships, enabling quantitative evaluation correlations based on semantic similarity. When combined Landsat multispectral data digital elevation (DEM)-derived parameters, knowledge-enhanced Random Forest (81.79%) Support Vector Machine (75.76%) models demonstrated excellent performance identifying rock-stratigraphic assemblages area. While reducing subjective biases manual interpretation, method still limitations. These include use cross-modal (e.g., geochemical tables, outcrop images) reliance static representations. Future research will introduce dynamic updating mechanisms multi-modal fusion architectures improve adaptability across diverse structural environments.
Language: Английский
Citations
0Ore Geology Reviews, Journal Year: 2024, Volume and Issue: 176, P. 106396 - 106396
Published: Dec. 13, 2024
Language: Английский
Citations
0