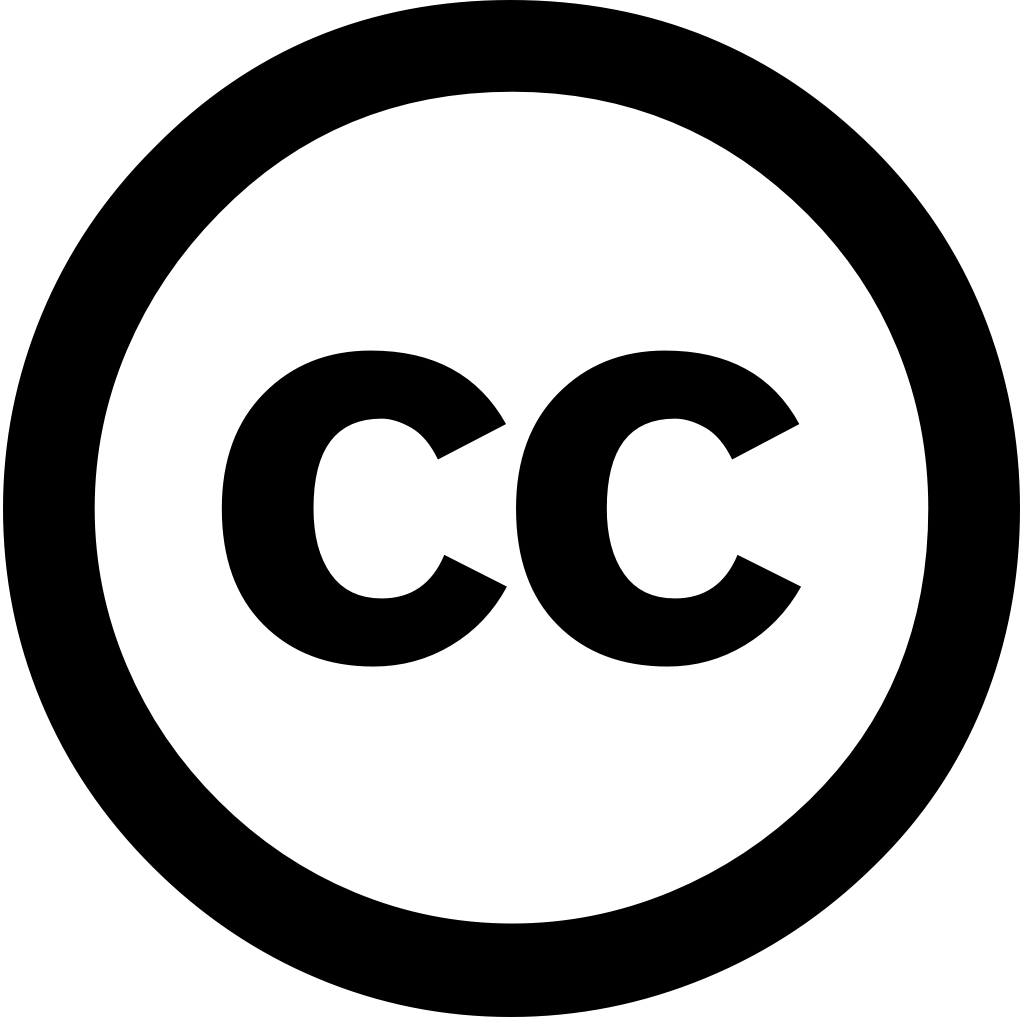
Journal of Imaging, Journal Year: 2024, Volume and Issue: 11(1), P. 5 - 5
Published: Dec. 31, 2024
The geometric feature characterization of fruit trees plays a role in effective management orchards. LiDAR (light detection and ranging) technology for object enables the rapid precise evaluation features. This study aimed to quantify height, canopy volume, tree spacing, row spacing an apple orchard using three-dimensional (3D) sensor. A sensor was used collect 3D point cloud data from orchard. Six samples trees, representing variety shapes sizes, were selected collection validation. Commercial software python programming language utilized process collected data. processing steps involved conversion, radius outlier removal, voxel grid downsampling, denoising through filtering erroneous points, segmentation region interest (ROI), clustering density-based spatial (DBSCAN) algorithm, transformation, removal ground points. Accuracy assessed by comparing estimated outputs with corresponding measured values. sensor-estimated heights 3.05 ± 0.34 m 3.13 0.33 m, respectively, mean absolute error (MAE) 0.08 root squared (RMSE) 0.09 linear coefficient determination (r2) 0.98, confidence interval (CI) −0.14 −0.02 high concordance correlation (CCC) 0.96, indicating strong agreement accuracy. volumes 13.76 2.46 m3 14.09 2.10 m3, MAE 0.57 RMSE 0.61 r2 value 0.97, CI −0.92 0.26, demonstrating precision. For distances 3.04 0.17 3.18 0.24 3.35 3.40 0.05 values 0.12 0.92 0.07 0.94 respectively. −0.18 0.01, −0.1, 0.002 Although minor differences observed, estimates efficient, though specific measurements require further refinement. results are based on limited dataset six values, providing initial insights into performance. However, larger would offer more reliable accuracy assessment. small sample size (six trees) limits generalizability findings necessitates caution interpreting results. Future studies should incorporate broader diverse validate refine characterization, enhancing practices
Language: Английский