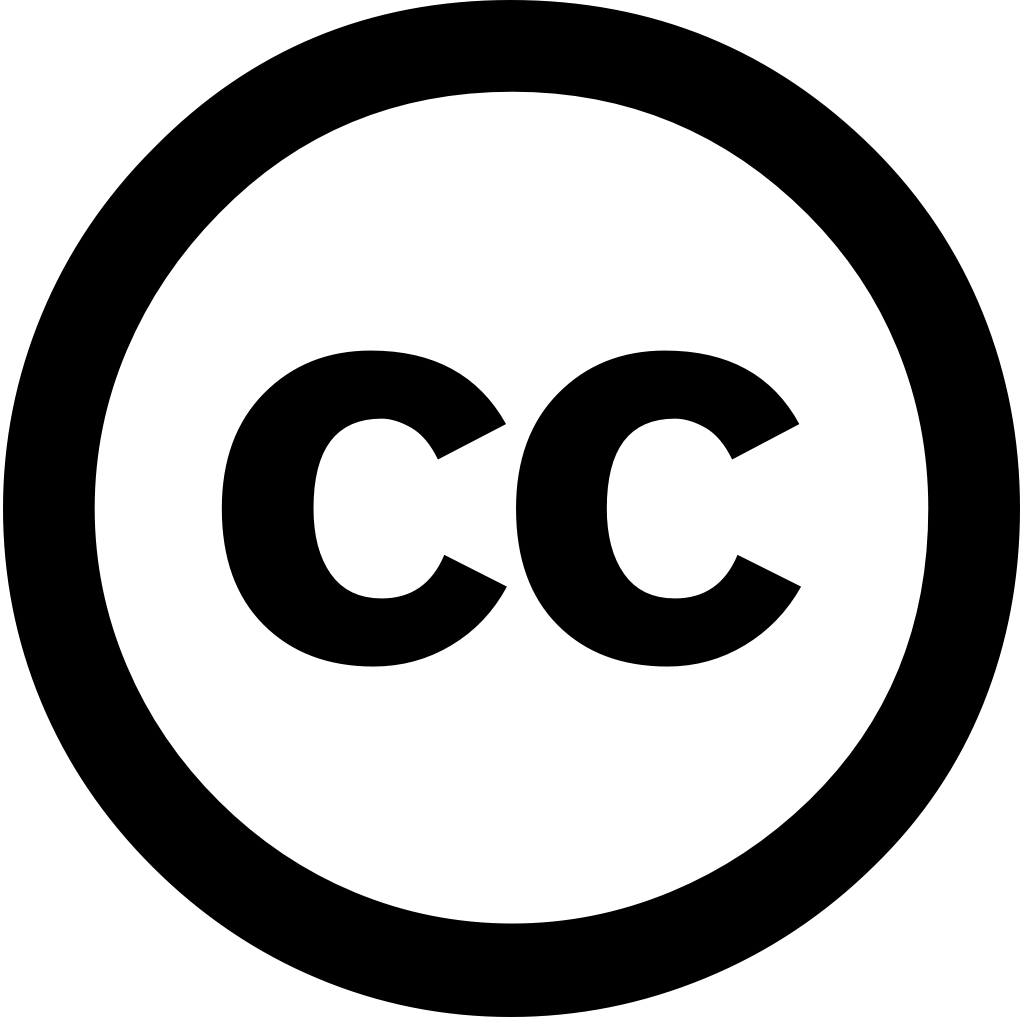
Research Square (Research Square), Journal Year: 2024, Volume and Issue: unknown
Published: Nov. 25, 2024
Language: Английский
Research Square (Research Square), Journal Year: 2024, Volume and Issue: unknown
Published: Nov. 25, 2024
Language: Английский
Energies, Journal Year: 2024, Volume and Issue: 17(8), P. 1815 - 1815
Published: April 10, 2024
In the realm of power systems, short-term electric load forecasting is pivotal for ensuring supply–demand balance, optimizing generation planning, reducing operational costs, and maintaining grid stability. Short-term curves are characteristically coarse, revealing high-frequency data upon decomposition that exhibit pronounced non-linearity significant noise, complicating efforts to enhance precision. To address these challenges, this study introduces an innovative model. This model employs complete ensemble empirical mode with adaptive noise (CEEMDAN) bifurcate original into low- components. For smoother low-frequency data, a temporal convolutional network (TCN) utilized, whereas components, which encapsulate detailed history information yet suffer from lower fitting accuracy, processed using enhanced soft thresholding TCN (SF-TCN) optimized slime mould algorithm (SMA). Experimental tests methodology on forecasts forthcoming 24 h across all seasons have demonstrated its superior accuracy compared non-decomposed models, such as support vector regression (SVR), recurrent neural (RNN), gated unit (GRU), long memory (LSTM), network-LSTM (CNN-LSTM), TCN, Informer, decomposed including CEEMDAN-TCN CEEMDAN-TCN-SMA.
Language: Английский
Citations
6Knowledge-Based Systems, Journal Year: 2024, Volume and Issue: 299, P. 111967 - 111967
Published: May 24, 2024
Language: Английский
Citations
4International Journal of Green Energy, Journal Year: 2025, Volume and Issue: unknown, P. 1 - 14
Published: March 25, 2025
Language: Английский
Citations
0Applied Sciences, Journal Year: 2025, Volume and Issue: 15(7), P. 4044 - 4044
Published: April 7, 2025
In multicriteria decision-making (MCDM), methods such as TOPSIS are essential for evaluating and comparing alternatives across multiple criteria. However, traditional normalization techniques often struggle with datasets containing outliers, large variances, or heterogeneous measurement units, which can lead to skewed biased rankings. To address these challenges, this paper proposes an adaptive, cluster-based approach, demonstrated through a real-world logistics case study involving the selection of host city international event. The method groups into clusters based on similarities in criterion values applies logarithmic within each cluster. This localized strategy reduces influence outliers ensures that scaling adjustments reflect specific characteristics group. study—where cities were evaluated cost, infrastructure, safety, accessibility—the yielded more stable balanced rankings, even presence significant data variability. By reducing allowing predefined cluster profiles expert judgment, improves fairness adaptability. These features strengthen TOPSIS’s ability deliver accurate, balanced, context-aware decisions complex, scenarios.
Language: Английский
Citations
0Sustainability, Journal Year: 2024, Volume and Issue: 16(16), P. 6903 - 6903
Published: Aug. 12, 2024
Accurate power load forecasting is critical to achieving the sustainability of energy management systems. However, conventional prediction methods suffer from low precision and stability because crude modules for predicting short-term medium-term loads. To solve such a problem, Combined Modeling Power Load-Forecasting (CMPLF) method proposed in this work. The CMPLF comprises two deal with forecasting, respectively. Each module consists four essential parts including initial decomposition denoising, nonlinear optimization, evaluation. Especially, break through bottlenecks hierarchical model we effectively fuse Nonlinear Autoregressive Exogenous Inputs (NARX) Long-Short Term Memory (LSTM) networks into Integrated Moving Average (ARIMA) model. experiment results based on real-world datasets Queensland China mainland show that our has significant performance superiority compared state-of-the-art (SOTA) methods. achieves goodness-of-fit value 97.174% 97.162% prediction. Our approach will be great significance promoting sustainable development smart cities.
Language: Английский
Citations
0Research Square (Research Square), Journal Year: 2024, Volume and Issue: unknown
Published: Nov. 25, 2024
Language: Английский
Citations
0