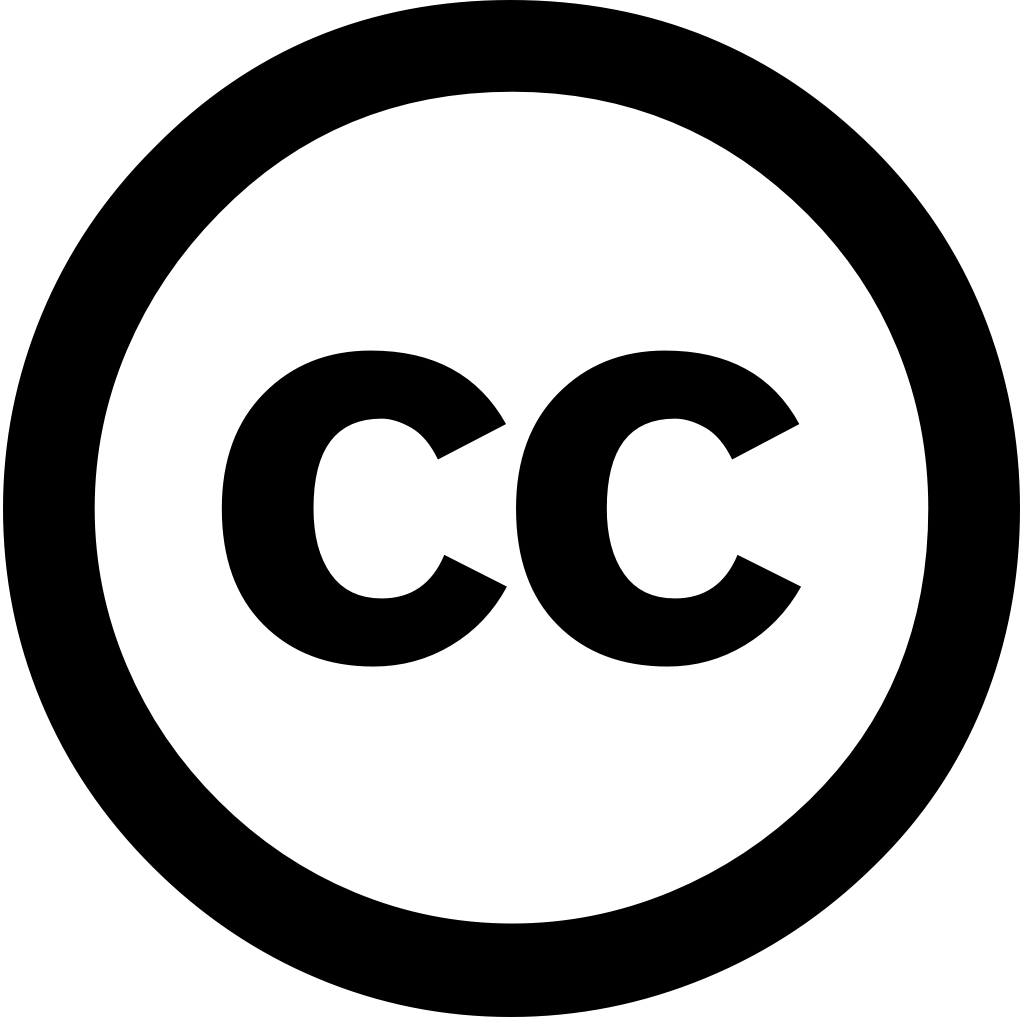
EURASIP Journal on Wireless Communications and Networking, Journal Year: 2024, Volume and Issue: 2024(1)
Published: Sept. 7, 2024
Language: Английский
EURASIP Journal on Wireless Communications and Networking, Journal Year: 2024, Volume and Issue: 2024(1)
Published: Sept. 7, 2024
Language: Английский
Computer Science Review, Journal Year: 2024, Volume and Issue: 54, P. 100680 - 100680
Published: Sept. 9, 2024
Language: Английский
Citations
7Internet of Things, Journal Year: 2024, Volume and Issue: 28, P. 101342 - 101342
Published: Aug. 23, 2024
Language: Английский
Citations
4Computer Science Review, Journal Year: 2025, Volume and Issue: 56, P. 100734 - 100734
Published: Feb. 7, 2025
Language: Английский
Citations
0Drones, Journal Year: 2025, Volume and Issue: 9(2), P. 153 - 153
Published: Feb. 19, 2025
Autonomous unmanned aerial vehicle (UAV) swarm networks (UAVSNs) can efficiently perform surveillance, connectivity, computing, and energy transfer services for ground users (GUs). These missions require trajectory planning, UAV-GUs association, task offloading, next-hop selection, resource allocation, including transmit power, bandwidth, timeslots, caching, computing resources, to enhance network performance. Owing the highly dynamic topology, limited stringent quality of service requirements, lack global knowledge, optimizing performance in UAVSNs is very intricate. To address this, an adaptive joint optimization framework required handle both discrete continuous decision variables, ensuring optimal under various constraints. A multi-agent deep reinforcement learning-based actor–critic offers effective solution by leveraging its ability extract hidden features through agent interactions, generate hybrid actions uncertainty, adaptively learn with scalable generalization conditions. This paper explores recent evolutions frameworks deal problems proposing a novel taxonomy based on modifications internal neural structure. Additionally, key open research challenges are identified, potential solutions suggested as directions future UAVSNs.
Language: Английский
Citations
0Vehicular Communications, Journal Year: 2025, Volume and Issue: unknown, P. 100899 - 100899
Published: Feb. 1, 2025
Language: Английский
Citations
0Computer Networks, Journal Year: 2025, Volume and Issue: unknown, P. 111280 - 111280
Published: April 1, 2025
Language: Английский
Citations
0Sustainable Computing Informatics and Systems, Journal Year: 2024, Volume and Issue: unknown, P. 101045 - 101045
Published: Oct. 1, 2024
Language: Английский
Citations
1EURASIP Journal on Wireless Communications and Networking, Journal Year: 2024, Volume and Issue: 2024(1)
Published: Sept. 7, 2024
Language: Английский
Citations
0