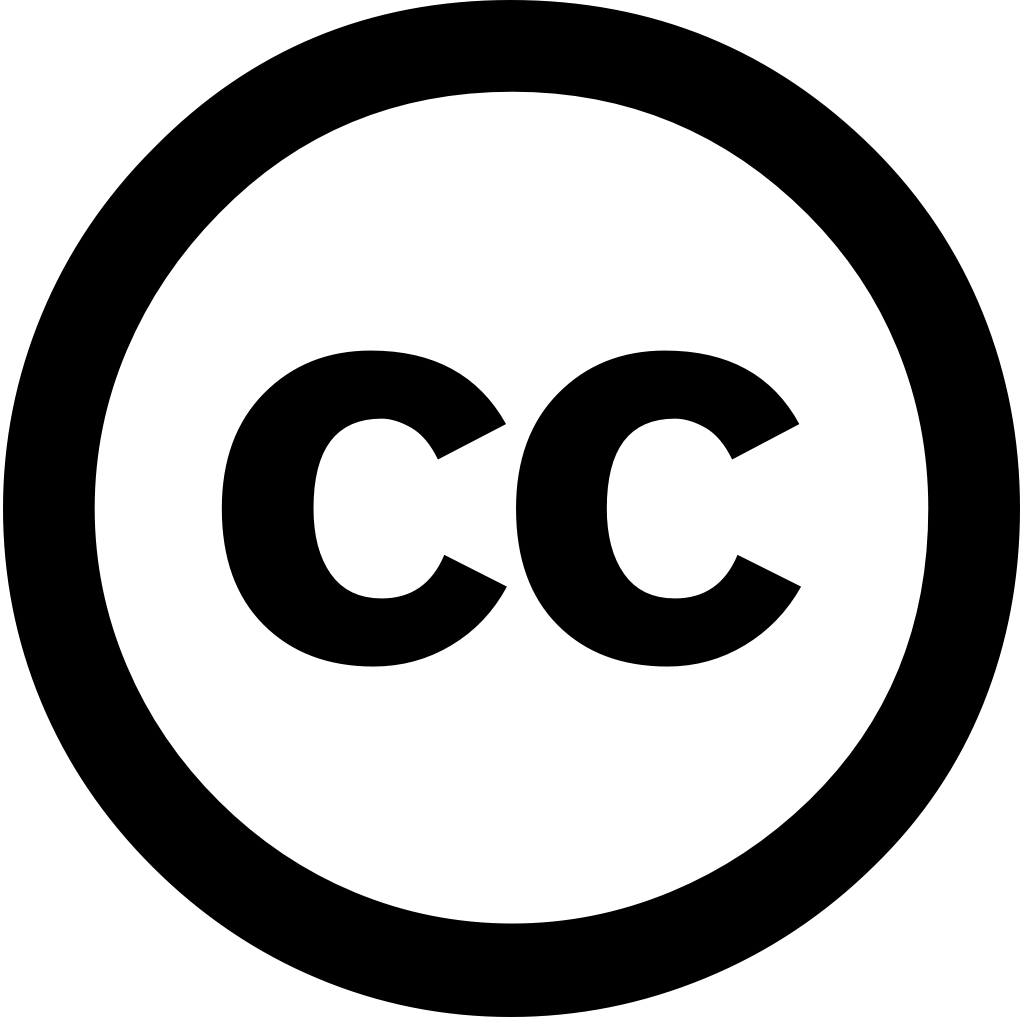
Sensors, Journal Year: 2024, Volume and Issue: 24(19), P. 6168 - 6168
Published: Sept. 24, 2024
As deep learning technology has progressed, automated medical image analysis is becoming ever more crucial in clinical diagnosis. However, due to the diversity and complexity of blood cell images, traditional models still exhibit deficiencies detection. To address detection, we developed TW-YOLO approach, leveraging multi-scale feature fusion techniques. Firstly, CNN (Convolutional Neural Network) convolution poor recognition capabilities for certain features, so RFAConv (Receptive Field Attention Convolution) module was incorporated into backbone model enhance its capacity extract geometric characteristics from cells. At same time, utilizing pyramid architecture YOLO (You Only Look Once), enhanced features at different scales by incorporating CBAM Block Module) detection head EMA (Efficient Multi-Scale Attention) neck, thereby improving ability Additionally, meet specific needs designed PGI-Ghost (Programmable Gradient Information-Ghost) strategy finely describe gradient flow throughout process extracting further model’s effectiveness. Experiments on datasets such as BloodCell-Detection-Dataset (BCD) reveal that outperforms other 2%, demonstrating excellent performance task In addition advancing research, this work offers strong technical support future diagnostics.
Language: Английский