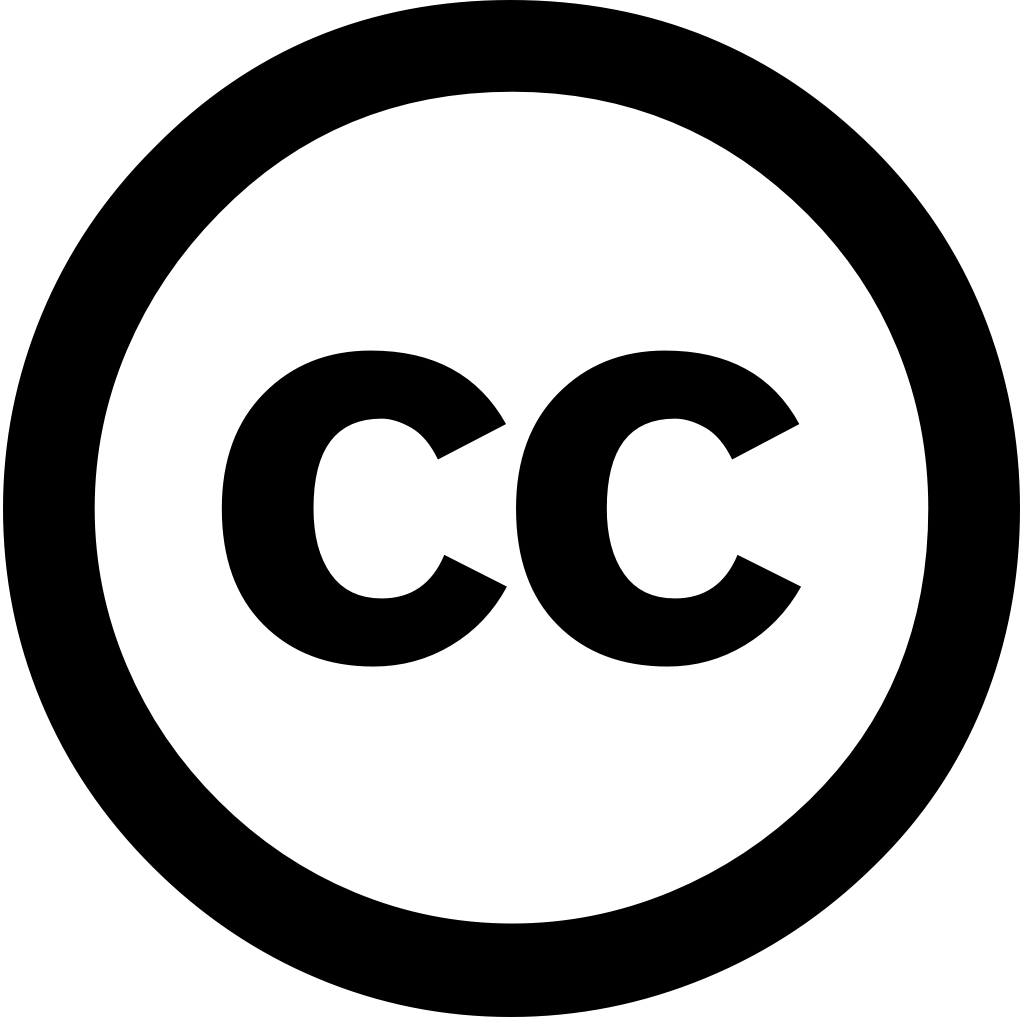
Agronomy, Journal Year: 2025, Volume and Issue: 15(3), P. 727 - 727
Published: March 18, 2025
The detection and identification of tea leaf diseases pests play a crucial role in determining the yield quality tea. However, high similarity between different difficulty balancing model accuracy complexity pose significant challenges during process. This study proposes an enhanced Tea Leaf Disease Detection Model (TLDDM), improved based on YOLOv8 to tackle challenges. Initially, C2f-Faster-EMA module is employed reduce number parameters while enhancing image feature extraction capabilities. Furthermore, Deformable Attention mechanism integrated improve model’s adaptability spatial transformations irregular data structures. Moreover, Slimneck structure incorporated scale. Finally, novel head structure, termed EfficientPHead, proposed maintain performance improving computational efficiency reducing which leads inference speed acceleration. Experimental results demonstrate that TLDDM achieves AP 98.0%, demonstrates enhancement compared SSD Faster R-CNN algorithm. not only great significance accuracy, but also can provide remarkable advantages real-time applications with FPS (frames per second) 98.2.
Language: Английский