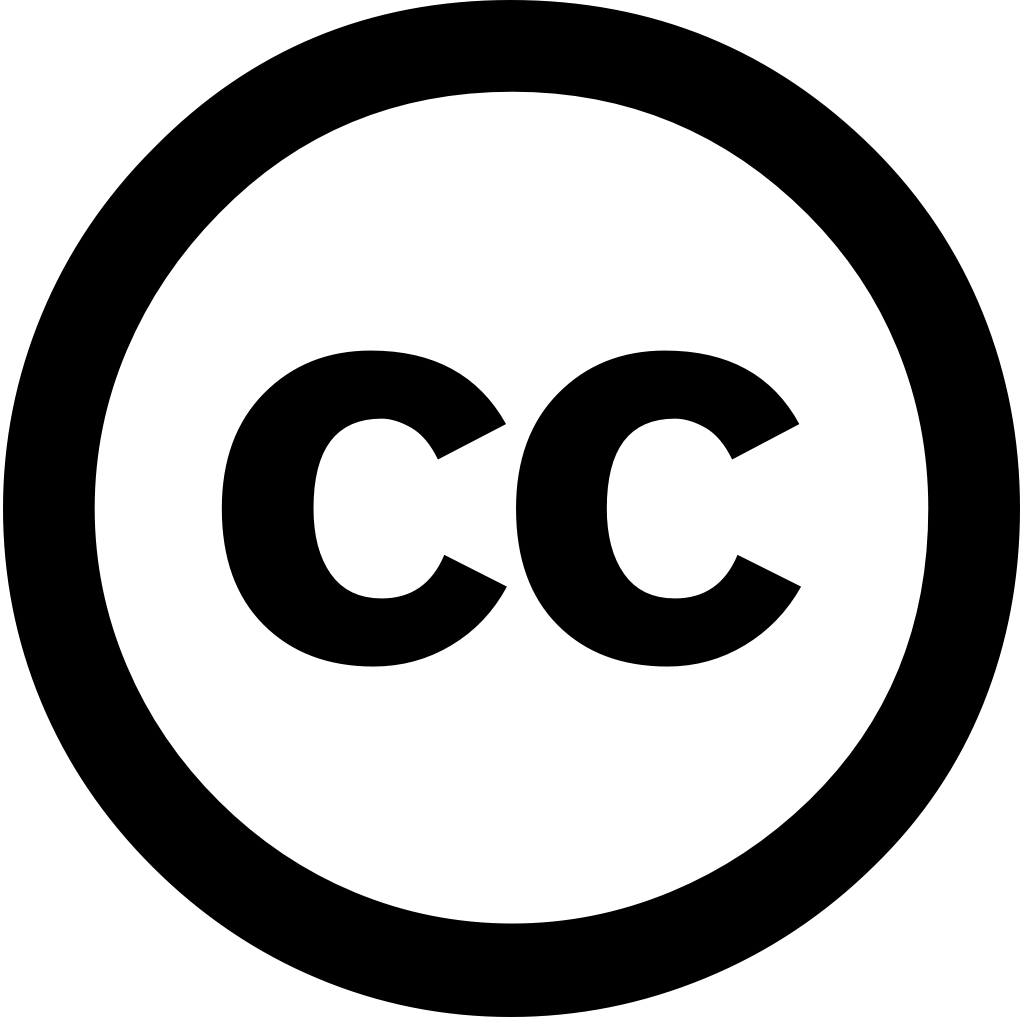
Sensors, Journal Year: 2025, Volume and Issue: 25(6), P. 1914 - 1914
Published: March 19, 2025
In the construction industry, along with traditional approaches for visual and instrumental assessment of building materials, methods based on intelligent algorithms are increasingly appearing; in particular, machine learning neural network technologies. The utilization modern technologies enables us to enhance processes a new quality level, decreasing pace without precision losses compared methods. This research introduces novel method characterizing crushed stone grain morphology using application specially designed three-dimensional computer vision networks point data clouds. Flakiness affects strength, adhesion, location grains. So, calculating this indicator by determining planar dimensions each particle is necessary its suitability various types work. Architectures PointNet PointCloudTransformer chosen as basis classification algorithms. input were 3D images grains, shapes which divided into needle-shaped, plate-shaped, cubic classes. accuracy metric achieved during training both models was 0.86. Using algorithms, analysis via manual selection, sieve analysis, or special equipment, will reduce labor can also serve an additional source verifying materials at stages construction.
Language: Английский