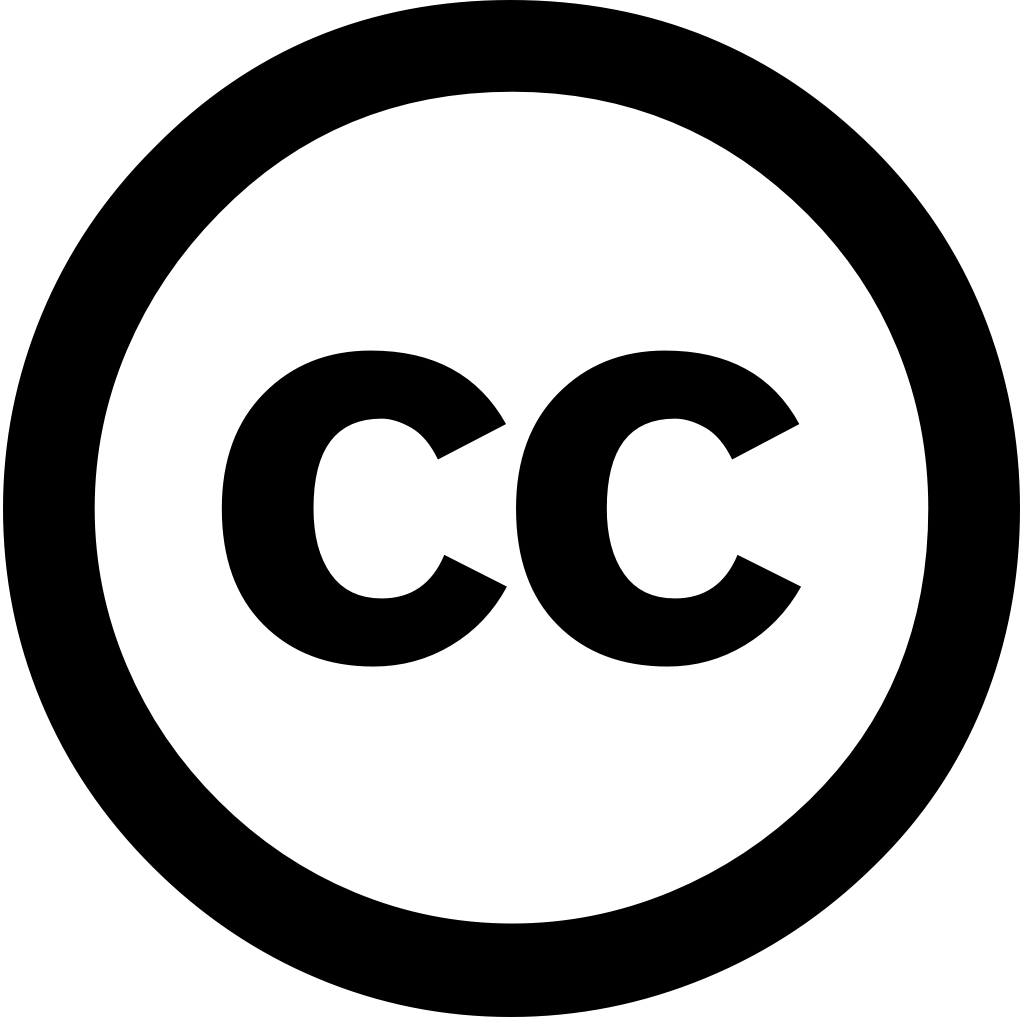
Atmosphere, Journal Year: 2025, Volume and Issue: 16(5), P. 523 - 523
Published: April 29, 2025
Air pollution remains one of the environmental issues affecting some countries, which leads to health globally. Though several machine learning and deep models are used analyze air pollutants, model interpretability is a challenge. Also, dynamic time-varying nature pollutants often creates noise in measurements, making pollutant prediction (e.g., Sulfur Dioxide (SO2) concentration) inaccurate, influences model’s performance. Recent advancements artificial intelligence (AI), particularly explainable AI, offer transparency trust models. In this regard, organizations using traditional confronted with how integrate AI into systems. paper, we propose novel approach that integrates (xAI) long short-term memory (LSTM) attempts address by Adaptive Kalman Filters (AKFs) also includes causal inference analysis. By utilizing LSTM, long-term dependencies daily concentration meteorological datasets (between 2008 2024) for City Kimberley, South Africa, captured analyzed multi-time steps. The proposed (AKF_LSTM_xAI) was compared Gate Recurrent Unit (GRU), LSTM-multilayer perceptron (LSTM-MLP) at different time performance evaluation results based on root mean square error (RMSE) one-day step suggest AKF_LSTM_xAI guaranteed 0.382, LSTM (2.122), LSTM_MLP (3.602), GRU (2.309). SHapley Additive exPlanations (SHAP) value reveals “Relative_humidity_t0” as most influential variable predicting SO2 concentration, whereas LIME values high “wind_speed_t0” reduces predicted concentration.
Language: Английский