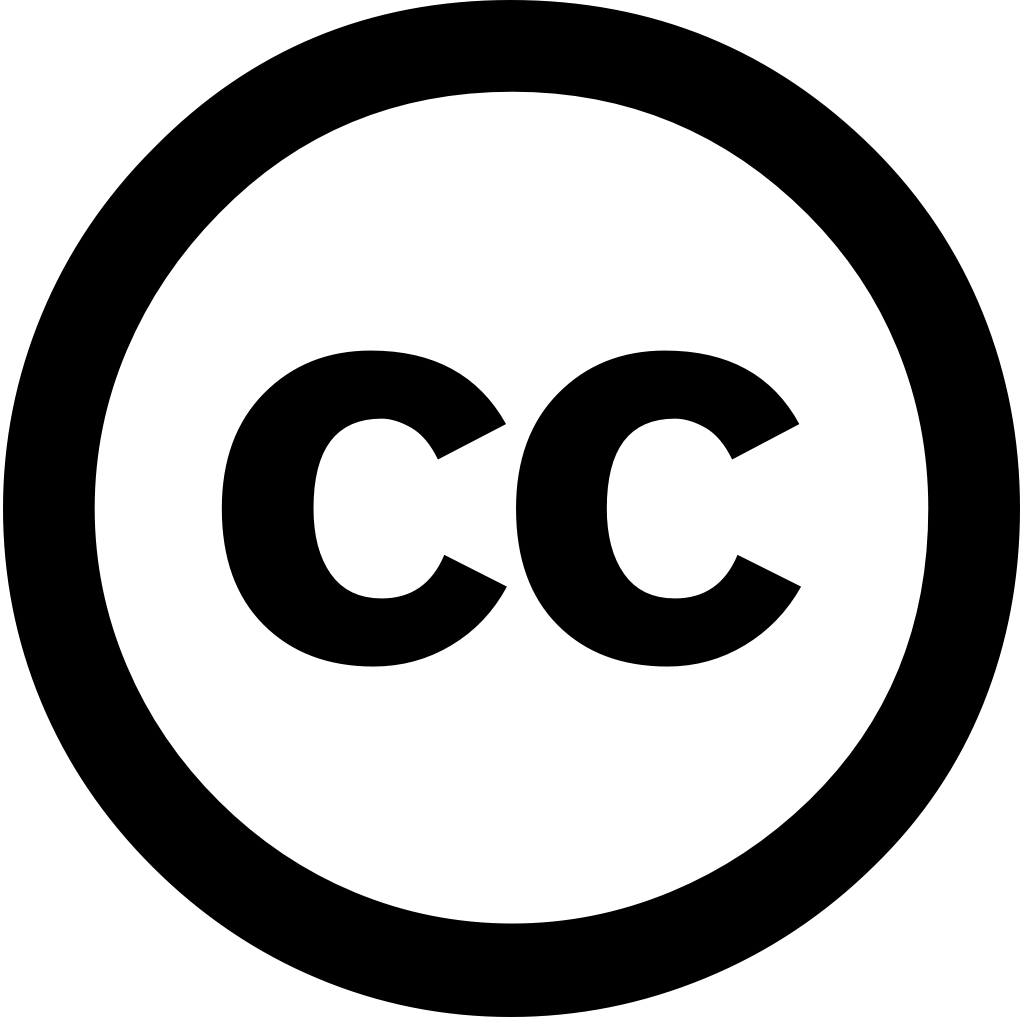
Research Square (Research Square), Journal Year: 2024, Volume and Issue: unknown
Published: Dec. 12, 2024
Language: Английский
Research Square (Research Square), Journal Year: 2024, Volume and Issue: unknown
Published: Dec. 12, 2024
Language: Английский
Sustainability, Journal Year: 2025, Volume and Issue: 17(2), P. 500 - 500
Published: Jan. 10, 2025
The energy sector plays a vital role in driving environmental and social advancements. Accurately predicting demand across various time frames offers numerous benefits, such as facilitating sustainable transition planning of resources. This research focuses on consumption using three individual models: Prophet, eXtreme Gradient Boosting (XGBoost), long short-term memory (LSTM). Additionally, it proposes an ensemble model that combines the predictions from all to enhance overall accuracy. approach aims leverage strengths each for better prediction performance. We examine accuracy Mean Absolute Error (MAE), Percentage (MAPE), Root Square (RMSE) through means resource allocation. investigates use real data smart meters gathered 5567 London residences part UK Power Networks-led Low Carbon project Datastore. performance was recorded follows: 62.96% Prophet model, 70.37% LSTM, 66.66% XGBoost. In contrast, proposed which XGBoost, achieved impressive 81.48%, surpassing models. findings this study indicate enhances efficiency supports towards future. Consequently, can accurately forecast maximum loads distribution networks households. addition, work contributes improvement load forecasting networks, guide higher authorities developing plans.
Language: Английский
Citations
2Electronics, Journal Year: 2024, Volume and Issue: 13(18), P. 3754 - 3754
Published: Sept. 21, 2024
Microgrids have emerged as a key element in the transition towards sustainable and resilient energy systems by integrating renewable sources enabling decentralized management. This systematic review, conducted using PRISMA methodology, analyzed 74 peer-reviewed articles from total of 4205 studies published between 2014 2024. review examines critical areas such reinforcement learning, multi-agent systems, predictive modeling, storage, optimization algorithms—essential for improving microgrid efficiency reliability. Emerging technologies like artificial intelligence (AI), Internet Things, flexible power electronics are highlighted enhancing management operational performance. However, challenges persist AI into complex, real-time control managing distributed resources. also identifies research opportunities to enhance scalability, resilience, efficiency, reaffirming their vital role solutions.
Language: Английский
Citations
11Deleted Journal, Journal Year: 2025, Volume and Issue: unknown, P. 100058 - 100058
Published: Jan. 1, 2025
Language: Английский
Citations
1Sustainability, Journal Year: 2024, Volume and Issue: 16(16), P. 7180 - 7180
Published: Aug. 21, 2024
This paper presents a comprehensive study on the optimization of electric vehicle (EV) battery management using Q-learning, powerful reinforcement learning technique. As demand for vehicles continues to grow, there is an increasing need efficient battery-management strategies extend life, enhance performance, and minimize operating costs. The primary objective this research develop assess Q-learning-based approach address intricate challenges associated with EV management. starts by elucidating key inherent in discusses potential advantages incorporating Q-learning into process. Leveraging Q-learning’s capacity make dynamic decisions based past experiences, we introduce framework that considers state-of-charge, state-of-health, charging infrastructure, driving patterns as critical state variables. methodology detailed, encompassing selection state, action, reward, policy, training process informed real-world data. Our experimental results underscore efficacy optimizing Through utilization achieve substantial enhancements energy efficiency, overall sustainability. A comparative analysis traditional presented highlight superior performance our approach. approach, demonstrating compelling results. method achieves significant 15% improvement efficiency compared conventional methods, translating savings operational costs reduced environmental impact. Moreover, observe remarkable 20% increase lifespan, showcasing effectiveness enhancing long-term sustainability user satisfaction. significantly enriches body knowledge introducing innovative, data-driven It provides applies novel methodologies practical implementation. implications beyond academic sphere applications, fostering broader adoption contributing reduction impact while
Language: Английский
Citations
4Journal of Atmospheric and Solar-Terrestrial Physics, Journal Year: 2025, Volume and Issue: 268, P. 106419 - 106419
Published: Jan. 10, 2025
Language: Английский
Citations
0Renewable and Sustainable Energy Reviews, Journal Year: 2025, Volume and Issue: 211, P. 115326 - 115326
Published: Jan. 16, 2025
Language: Английский
Citations
0Electric Power Systems Research, Journal Year: 2025, Volume and Issue: 243, P. 111511 - 111511
Published: Feb. 12, 2025
Language: Английский
Citations
0Engineering Applications of Artificial Intelligence, Journal Year: 2025, Volume and Issue: 148, P. 110367 - 110367
Published: March 1, 2025
Language: Английский
Citations
0E3S Web of Conferences, Journal Year: 2025, Volume and Issue: 626, P. 04003 - 04003
Published: Jan. 1, 2025
This paper presents an ensemble learning approach to predict the active power losses during allocation and sizing of distributed generation (DG) units in distribution networks. The forecast model incorporates Gradient Boosting Machine Regression (GBMR) estimate DG location, bus voltages, size, without conventional flow calculations. results demonstrate that suggested estimations are effective, practical, adaptable for system management. accuracy proposed has been validated using key performance metrics tested on standard IEEE 33 system. In case fixed load, GBMR outperforms other machine techniques with R-squared 0.9997, a very low mean absolute percentage error (MAPE) (0.2216%) root square (RMSE) 1.0673 predicting losses. is promising enabling grid operators effectively manage unit integration energy resources from precise reliable estimates loss.
Language: Английский
Citations
0Deleted Journal, Journal Year: 2025, Volume and Issue: 7(5)
Published: May 3, 2025
Abstract Microgrids, comprised of interconnected loads and distributed energy resources, function as single controllable entities with respect to the main grid. However, inherent variability wind solar generation within microgrids presents operational stability challenges concerning voltage regulation frequency stability. Accurate forecasting renewable is crucial for mitigating these challenges. This work proposes a one-dimensional Convolutional Neural Network (1-D CNN) based approach forecast photovoltaic (PV) energy, using data from University California, San Diego microgrid Airport weather records. The proposed method evaluated against various methods key metrics: Mean Squared Error (MSE), Absolute (MAE), Root (RMSE), R-squared value. Results show that 1-D CNN model achieves an improvement up 229.8 times in MSE 24.47 fold MAE compared baseline models use traditional statistical forecasting. demonstrates potential machine learning enhancing management, particularly short-term generation.
Language: Английский
Citations
0