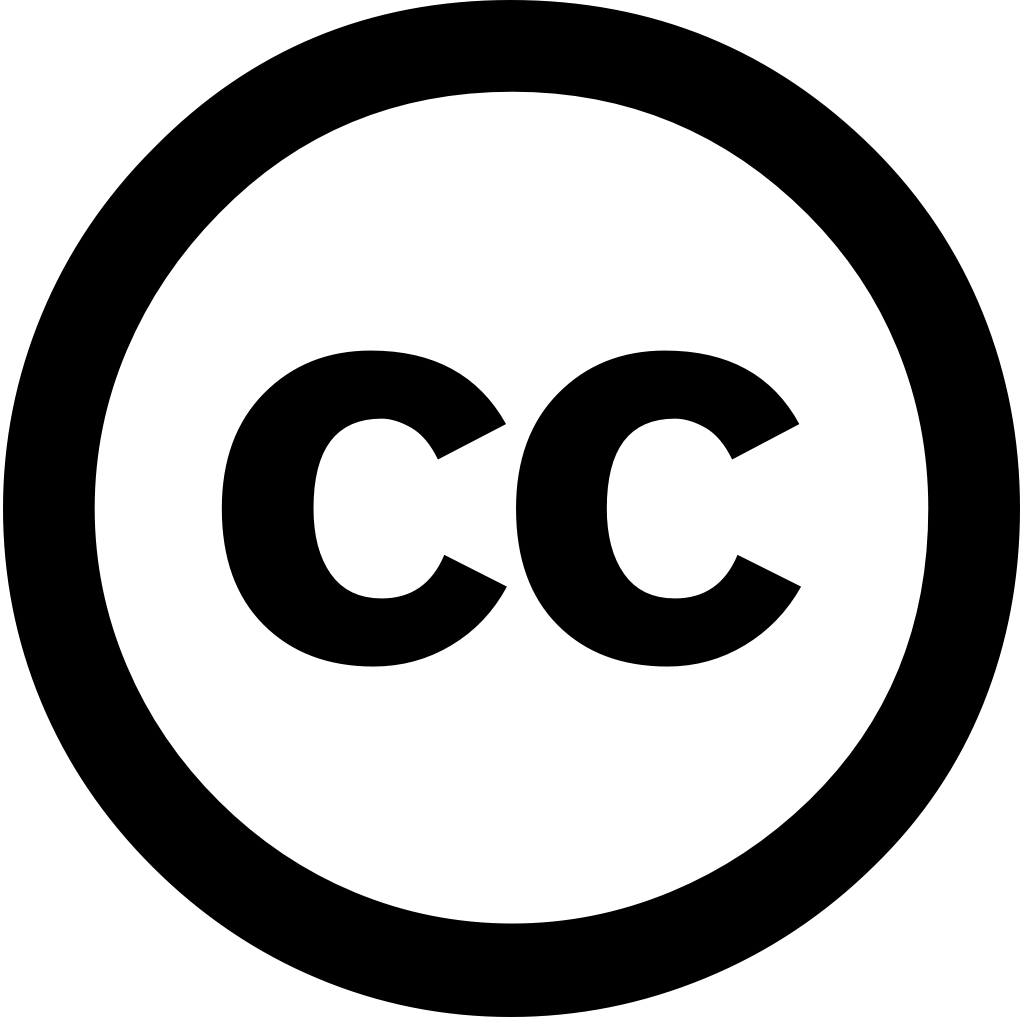
Plants, Journal Year: 2025, Volume and Issue: 14(3), P. 451 - 451
Published: Feb. 3, 2025
Huanglongbing is one of the most destructive diseases citrus worldwide. Infected trees die due to absence practical cures. Thus, removal HLB-infected principal HLB managements for regulation disease spread. Here, we propose a non-destructive detection method based on hyperspectral leaf reflectance. In total, 72 images were collected in an HLB-invaded orchard Thailand and each image was visually distinguished into either any symptom appearance (symptomatic) or no symptoms (asymptomatic) leaf. Principal component analysis applied data revealed 16 key wavelengths at red-edge near-infrared regions (715, 718, 721, 724, 727, 730, 733, 736, 930, 933, 936, 939, 942, 945, 957, 997 nm) that characteristically differentiated symptomatic group. Seven models learnt spectral these examined potential separate two groups: random forest, decision tree, support vector machine, k-nearest neighbor, gradient boosting, logistic regression, linear discriminant. F1-score employed select best-fit model distinguish categories: forest achieved best score 99.8%, followed by tree neighbor. The reliability visual grouping evaluated nearest neighbor matching permutation test. These three separated categories as precisely PCR results, indicating their alternative tool instead PCR.
Language: Английский