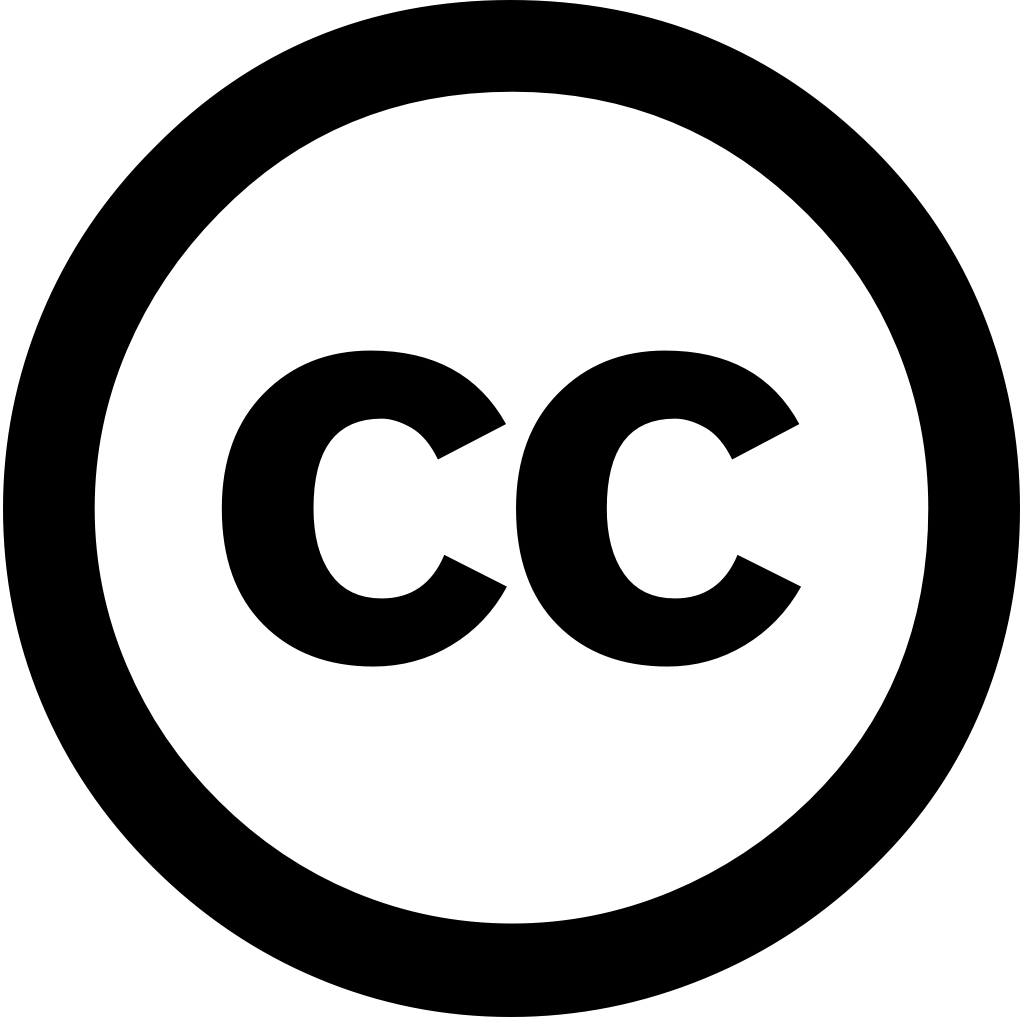
Advanced Science, Journal Year: 2025, Volume and Issue: unknown
Published: Feb. 3, 2025
Abstract Unexpected toxicity has become a significant obstacle to drug candidate development, accounting for 30% of discovery failures. Traditional assessment through animal testing is costly and time‐consuming. Big data artificial intelligence (AI), especially machine learning (ML), are robustly contributing innovation progress in toxicology research. However, the optimal AI model different types usually varies, making it essential conduct comparative analyses methods across domains. The diverse sources also pose challenges researchers focusing on specific studies. In this review, 10 categories drug‐induced examined, summarizing characteristics applicable ML models, including both predictive interpretable algorithms, striking balance between breadth depth. Key databases tools used prediction highlighted, toxicology, chemical, multi‐omics, benchmark databases, organized by their focus function clarify roles prediction. Finally, strategies turn into opportunities analyzed discussed. This review may provide with valuable reference understanding utilizing available resources bridge mechanistic insights, further advance application drugs‐induced
Language: Английский