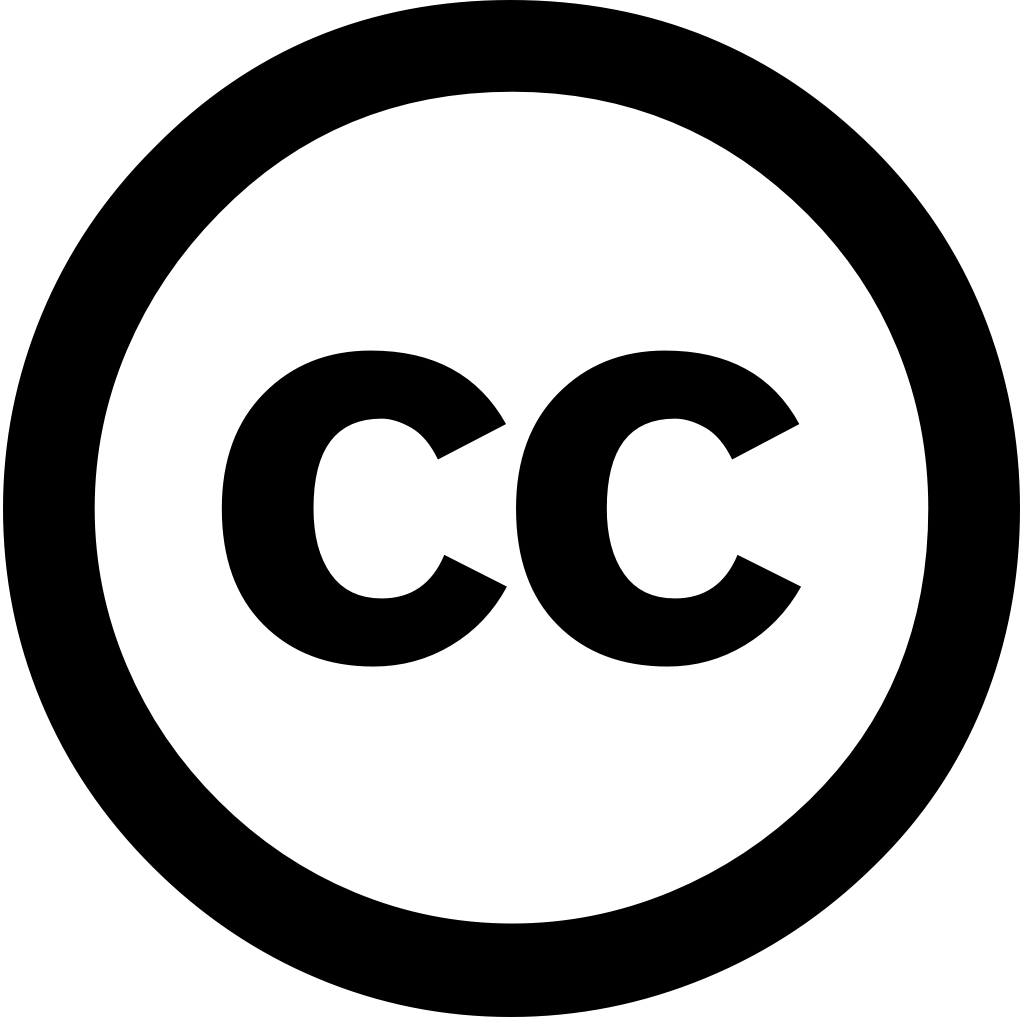
Scientific Reports, Journal Year: 2024, Volume and Issue: 14(1)
Published: Oct. 26, 2024
Language: Английский
Scientific Reports, Journal Year: 2024, Volume and Issue: 14(1)
Published: Oct. 26, 2024
Language: Английский
Space Weather, Journal Year: 2024, Volume and Issue: 22(3)
Published: March 1, 2024
Abstract Accurately predicting and modeling the ionospheric total electron content (TEC) can greatly improve accuracy of satellite navigation positioning help to correct delay. This study assesses effectiveness four different machine learning (ML) models in hourly vertical TEC (VTEC) data for a single‐station over Ethiopia. The employed include gradient boosting (GBM), extreme (XGBoost), light (LightGBM) algorithms, stacked combination these algorithms with linear regression algorithm. relied on input variables that represent solar activity, geomagnetic season, time day, interplanetary magnetic field, wind. were trained using VTEC from January 2011 December 2018, excluding testing data. comprised year 2015 initial 6 months 2017. RandomizedSearchCV algorithm was used determine optimal hyperparameters models. predicted values ML strongly correlated GPS VTEC, correlation coefficient ∼0.96, which is significantly higher than corresponding value International Reference Ionosphere (IRI 2020) model, 0.87. Comparing based diurnal seasonal characteristics showed predictions developed generally good agreement outperformed IRI 2020 model. Overall, this demonstrated promising potential accurate prediction
Language: Английский
Citations
52022 8th International Conference on Control, Decision and Information Technologies (CoDIT), Journal Year: 2023, Volume and Issue: unknown, P. 2061 - 2066
Published: July 3, 2023
This paper presents a hybrid method for accurately predicting Global Horizontal Irradiance (GHI) over the following 24 hours to forecast energy production from photo-voltaic system in positive building. The input data is preprocessed using Variational Mode Decomposition (VMD) extract wide-bandwidth features and decompose them into smooth modes focused on specific frequency ranges. Salp Swarm Algorithm (SSA) utilized identify optimal VMD parameters accurate extraction. analysis employed most critical of features. model's efficiency further enhanced by performing residual preprocessing step between observed solar radiance decomposed modes. Stacking technique (ST) predict 24-hour GHI residual, which are summed reconstruct final signal. proposed method's performance evaluated Normalized Root Mean Square Error (NRMSE) Absolute (NMAE) metrics three years available (2019–2022) Rabat, compared with model based raw data. results show that achieved promising an NRMSE 1.35% NMAE 0.82% cloudy day.
Language: Английский
Citations
12Results in Engineering, Journal Year: 2023, Volume and Issue: 21, P. 101704 - 101704
Published: Dec. 30, 2023
Hidden and perilous rip currents are one of the primary factors leading to drownings beach swimmers. By identifying coastal areas with highest likelihood generating currents, it becomes possible prevent fatalities mitigate economic losses associated these hazardous currents. Rip characterized as streams water moving towards open sea, forming within area where waves break, due variations in wave-induced radiation stresses pressure along coastline. This study utilized nine different Machine Learning (ML) models, including M5 Model Tree (MT), Multivariate Adaptive Regression Spline (MARS), Gene Expression Programming (GEP), Evolutionary Polynomial (EPR), Random Forest (RF), Support Vector (SVM), Extreme Gradient Boosting (XGBoost), (AdaBoost), Stacked ML estimate Relative Tide Range (RTR) values for 50 southern beaches China. Through this approach, we gathered a reliable dataset from prior research conducted on coast In study, two parameters, namely dimensionless fall velocity parameter (Ω) TR used predict vulnerability current event. The results AI models were assessed by various statistical analyses (Correlation Coefficient [R], Root Mean Square Error [RMSE], violin diagram, heatmap, taylor diagram) training testing stages. Accordingly, MARS model exhibited superior performance compared other accurately predicting RTR value. outcomes substantiated significant effectiveness capability estimating high accuracy. Southern China coasts have relative risk level current, necessitating attention strategic management dangerous managers.
Language: Английский
Citations
11Journal of Hydroinformatics, Journal Year: 2024, Volume and Issue: 26(5), P. 972 - 997
Published: April 16, 2024
ABSTRACT The present study aims to evaluate the potentiality of Bidirectional Long Short-Term Memory (Bi-LSTM), Convolutional Neural Networks (CNNs), eXtreme Gradient Boosting (XGBoost), Light Machine (LGBM), and Random Forest (RF) for predicting daily inflows Sri Ram Sagar Project (SRSP), Telangana, India. Inputs model are rainfall, evaporation, time lag inflows, climate indices. Seven combinations (S1–S7) inputs were made. Fifteen a half years data considered, out which 11 used training. Hyperparameter tuning is performed with Tree-Structured Parzen Estimator. performance algorithms assessed using Kling–Gupta efficiency (KGE). Results indicate that Bi-LSTM combination S7 better than others, as evident from KGE values 0.92 0.87 during training testing, respectively. Furthermore, Stacking Ensemble Mechanism (SEM) has also been employed ascertain its efficacy over other chosen algorithms, resulting in 0.94 0.89 testing. It able simulate peak inflow events satisfactorily. Thus, SEM alternative reservoir predictions.
Language: Английский
Citations
4Remote Sensing, Journal Year: 2024, Volume and Issue: 16(12), P. 2060 - 2060
Published: June 7, 2024
The fall armyworm (Spodoptera frugiperda) (J. E. Smith) is a widespread, polyphagous, and highly destructive agricultural pest. Global climate change may facilitate its spread to new suitable areas, thereby increasing threats host plants. Consequently, predicting the potential distribution for plants under current future scenarios crucial assessing outbreak risks formulating control strategies. This study, based on remote sensing assimilation data plant protection survey data, utilized machine learning methods (RF, CatBoost, XGBoost, LightGBM) construct prediction models 120 Hyperparameter stacking ensemble method (SEL) were introduced optimize models. results showed that SEL demonstrated optimal performance in armyworm, with an AUC of 0.971 ± 0.012 TSS 0.824 0.047. Additionally, LightGBM 47 30 plants, respectively. Overlay analysis suggests overlap areas interaction links between will generally increase future, most significant rise RCP8.5 scenario, indicating threat further intensify due change. findings this study provide support planning implementing global intercontinental long-term pest management measures aimed at mitigating impact food production.
Language: Английский
Citations
4International Journal of Cognitive Computing in Engineering, Journal Year: 2025, Volume and Issue: unknown
Published: Jan. 1, 2025
Language: Английский
Citations
0Journal of risk and financial management, Journal Year: 2025, Volume and Issue: 18(2), P. 74 - 74
Published: Feb. 2, 2025
The financial technology domain has undertaken significant strides toward more inclusive credit scoring systems by integrating alternative data sources, prompting an exploration of how we can further simplify the process efficiently assessing creditworthiness for younger generation who lack traditional histories and collateral assets. This study introduces a novel approach leveraging social media analytics advanced machine learning techniques to assess individuals without Conventional methods tend rely heavily on central bank information, especially assets such as property or savings accounts. We leverage demographics, personality, psycholinguistics, network from LinkedIn profiles develop predictive models comprehensive reliability assessment. Our propose produce continuous scores classification categorize potential borrowers—particularly young lacking assets—as either good bad risks based expert judgment thresholds. innovative questions conventional evaluation enhances access marginalized communities. research question addressed in this is mechanism using data. contributes advancing fintech landscape presenting framework that transform practices adapt modern economic activities digital footprints.
Language: Английский
Citations
0Environmental Research Communications, Journal Year: 2025, Volume and Issue: 7(3), P. 035006 - 035006
Published: Feb. 24, 2025
Abstract Predicting crop yields remains a crucial challenge in agriculture, as these forecasts influence decision-making at global, regional, and individual levels. Historically, such predictions have utilized diverse data sources, including agricultural, land, climatic, atmospheric, other pertinent information. Over the past several years, application of machine learning techniques has emerged valuable analytical approach for estimating agricultural productivity, thereby informing decisions regarding selection management strategies throughout entire growing cycle. Various kinds models been research to forecast yields. Our work proposes stacked ensemble model designed purpose predicting yield. The proposed employs approach, with Decision Tree Regressor functioning meta-model amalgamate from six distinct base learner models: Linear Regression (LR), Elastic Net, XGBoost Regressor, K-Neighbors (KNR), AdaBoost Random Forest (RFR). achieves superior yield prediction performance, evidenced by notable enhancement accuracy significant decrease RMSE, surpassing predictive capabilities traditional models. model’s performance was assessed using metrics, Mean Absolute Error 7.20 tons/hectare, Square 15570.32 tons 2 /hectare , Root 124.78 Coefficient Determination (R Score) 0.98. results demonstrate that outperforms conventional approaches, achieving high R-squared score 98%.
Language: Английский
Citations
0Published: March 24, 2025
Language: Английский
Citations
0Journal of Sensor and Actuator Networks, Journal Year: 2025, Volume and Issue: 14(2), P. 44 - 44
Published: April 18, 2025
Chest X-ray interpretation is essential for diagnosing cardiac and respiratory diseases. This study introduces a deep learning ensemble approach that integrates Convolutional Neural Networks (CNNs), including ResNet-152, VGG19, EfficientNet, Vision Transformer (ViT), to enhance diagnostic accuracy. Using the NIH dataset, methodology involved comprehensive preprocessing, data augmentation, model optimization techniques address challenges such as label imbalance feature variability. Among individual models, VGG19 exhibited strong performance with Hamming Loss of 0.1335 high accuracy in detecting Edema, while ViT excelled classifying certain conditions like Hernia. Despite strengths meta-model achieved best overall performance, 0.1408 consistently higher ROC-AUC values across multiple diseases, demonstrating its superior capability handle complex classification tasks. robust framework underscores potential reliable precise disease detection, offering significant improvements over traditional methods. The findings highlight value integrating diverse architectures complexities multi-label chest classification, providing pathway more accurate, scalable, accessible tools clinical practice.
Language: Английский
Citations
0