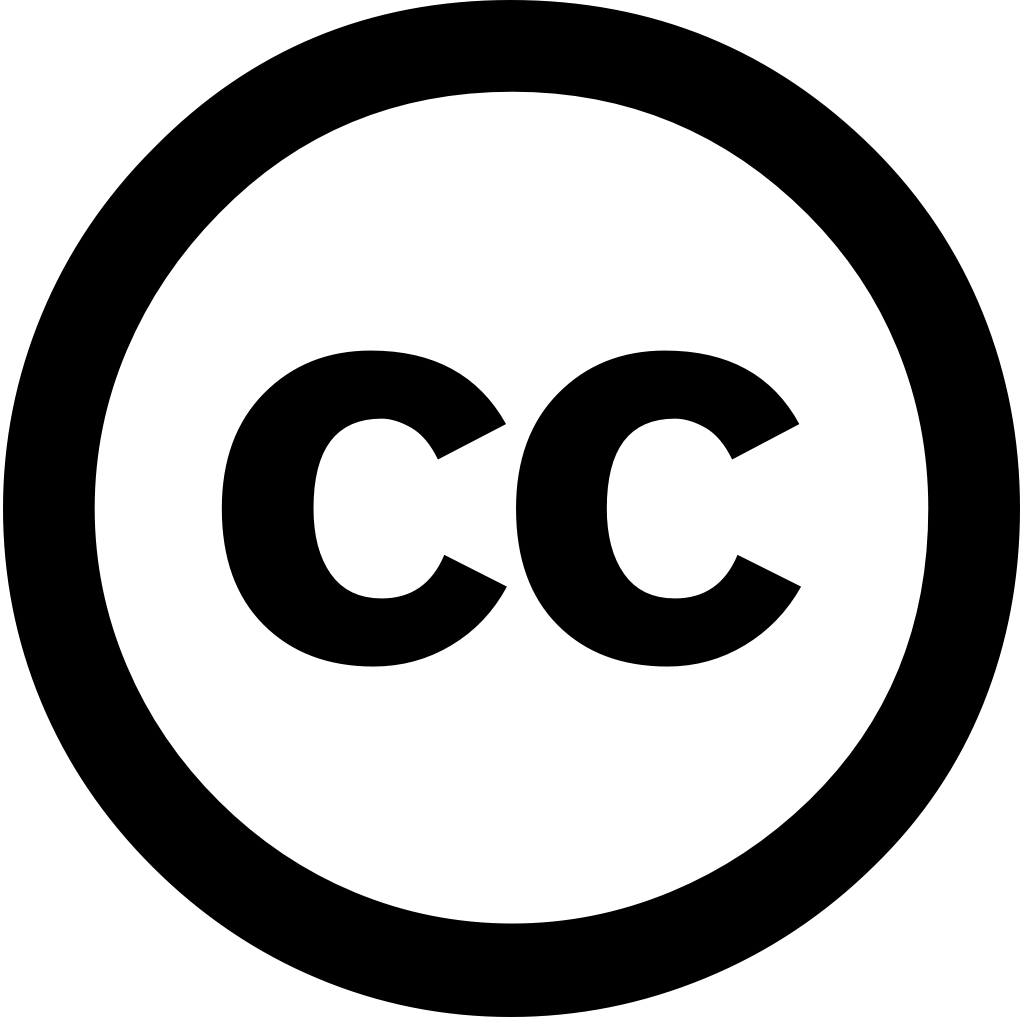
HydroResearch, Journal Year: 2024, Volume and Issue: 8, P. 139 - 151
Published: Oct. 30, 2024
Language: Английский
HydroResearch, Journal Year: 2024, Volume and Issue: 8, P. 139 - 151
Published: Oct. 30, 2024
Language: Английский
Results in Engineering, Journal Year: 2025, Volume and Issue: unknown, P. 104079 - 104079
Published: Jan. 1, 2025
Language: Английский
Citations
3Water, Journal Year: 2024, Volume and Issue: 16(2), P. 364 - 364
Published: Jan. 22, 2024
River flood routing computes changes in the shape of a wave over time as it travels downstream along river. Conventional models, especially hydrodynamic require high quality and quantity input data, such measured hydrologic series, geometric hydraulic structures, hydrological parameters. Unlike physically based machine learning algorithms, which are data-driven do not much knowledge about underlying physical processes can identify complex nonlinearity between inputs outputs. Due to their higher performance, lower complexity, low computation cost, researchers introduced novel methods single application or hybrid achieve more accurate efficient routing. This paper reviews recent river
Language: Английский
Citations
5Published: Jan. 3, 2024
River flood routing computes changes in shape of a wave over time as it travels downstream along river. Conventional models, especially hydrodynamic models require high quality and quantity input data such measured hydrologic series, geometric data, hydraulic structures hydrological parameters. Unlike physically based machine learning algorithms, which are driven do not much knowledge about underlying physical processes can identify complex nonlinearity between inputs outputs. Due to the higher performance, less complexity, low computation cost, novel methods single application or hybrid were introduced by researchers achieve more accurate efficient routing. This paper reviews recent river
Language: Английский
Citations
2Pure and Applied Geophysics, Journal Year: 2024, Volume and Issue: unknown
Published: Oct. 26, 2024
Language: Английский
Citations
2Geomatics Natural Hazards and Risk, Journal Year: 2023, Volume and Issue: 14(1)
Published: Nov. 10, 2023
Under global warming, the acceleration of water cycle has increased risk drought in Yellow River Basin. Revealing driving mechanisms basin and understanding situation have become particularly important. This paper uses wavelet analysis transfer entropy to analyze mechanisms. In addition, an Improved Particle Swarm Optimization (IPSO) coupled with Long Short-Term Memory (LSTM) is used for prediction. The results are as follows: (1) Hydrological lags behind meteorological by 2–3 months, they show two main periods on different time scales, which 5–6 months 8–14 respectively. (2) Rainfall, runoff, temperature, humidity, vapor pressure factors, rainfall humidity having most significant impact. (3) IPSO-LSTM model improved process selecting parameters based empirical experiences LSTM model, improving prediction accuracy average 3.1%. provides a scientific basis resource management assessment basin, better cope future climate challenges.
Language: Английский
Citations
6Water, Journal Year: 2024, Volume and Issue: 16(15), P. 2130 - 2130
Published: July 27, 2024
Accurate runoff prediction is crucial for watershed water resource management, flood prevention, and hydropower station scheduling. Data-driven models have been increasingly applied to tasks achieved impressive results. However, existing data-driven methods may produce unreasonable predictions due the lack of prior knowledge guidance. This study proposes a multivariate model that couples embedding with approaches, integrating information contained in probability distributions as constraints into optimizing loss function density functions (PDFs). Using main stream Yellow River Basin nine hydrological stations an example, we selected feature factors using transfer entropy method, chose temporal convolutional network (TCN) model, optimized parameters IPSO algorithm, studying univariate input (TCN-UID), multivariable (TCN-MID), coupling model. The results indicate following: (1) Among numerous influencing factors, precipitation, sunshine duration, relative humidity are key driving occurrence; (2) can effectively fit extremes sequences, improving accuracy training set by 6.9% 4.7% compared TCN-UID TCN-MID, respectively, 5.7% 2.8% test set. established through not only retains advantages but also addresses poor performance at extremes, thereby enhancing predictions.
Language: Английский
Citations
1Water Science & Technology Water Supply, Journal Year: 2023, Volume and Issue: 23(11), P. 4403 - 4415
Published: Oct. 31, 2023
Abstract Flood routing is vital in helping to reduce the impact of floods on people and communities by allowing timely appropriate responses. In this study, empirical mode decomposition (EMD) signal technique combined with cascade forward backpropagation neural network (CFBNN) feed-forward (FFBNN) machine learning (ML) techniques model 2014 Ankara, Mera River. The data are split order avoid underfitting overfitting problems algorithm. While establishing algorithm, 70% were divided into training, 15% testing validation. Graphical indicators statistical parameters used for analysis performance. As a result, EMD has been found improve performance ML models. addition, EMD-FFBNN hybrid showed most accurate estimation results flood calculation. study's outputs can assist designing control structures such as levees dams help risk.
Language: Английский
Citations
3Water, Journal Year: 2023, Volume and Issue: 16(1), P. 43 - 43
Published: Dec. 21, 2023
Since improving floodwater utilization may increase flood risk, risk control methods for trade-offs between these factors have research value. This study presented a method oriented towards which considers multiple main factors. The proposed not only achieves the boundaries of limited water level (FLWL) under various acceptable risks but also dynamically controls to enhance utilization. A case conducted on Danjiangkou reservoir yielded following results: (1) provides FLWL dynamic risks. (2) reveals potential raise FLWL, with possibility it by 1.00 m above present absence risk. (3) available resources in both wet and dry seasons increase, average, 0.83 0.81 billion m3, remains within range after raising m, contributes enhancing
Language: Английский
Citations
2HydroResearch, Journal Year: 2024, Volume and Issue: 8, P. 139 - 151
Published: Oct. 30, 2024
Language: Английский
Citations
0