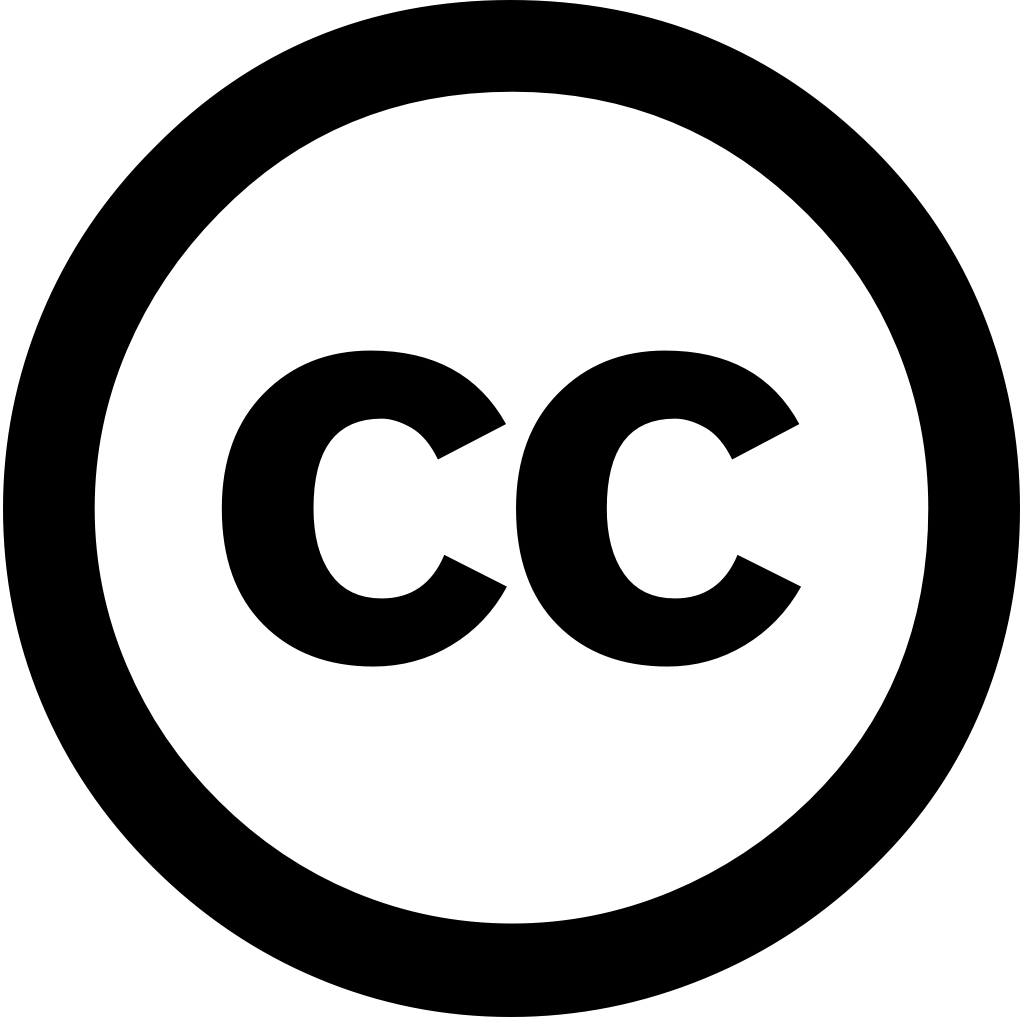
Journal of Environmental Management, Journal Year: 2024, Volume and Issue: 373, P. 123570 - 123570
Published: Dec. 6, 2024
Language: Английский
Journal of Environmental Management, Journal Year: 2024, Volume and Issue: 373, P. 123570 - 123570
Published: Dec. 6, 2024
Language: Английский
Sustainability, Journal Year: 2024, Volume and Issue: 16(16), P. 6897 - 6897
Published: Aug. 11, 2024
Runoff is greatly influenced by changes in climate conditions. Predicting runoff and analyzing its variations under future climates are crucial for ensuring water security, managing resources effectively, promoting sustainable development within the catchment area. As key step modeling, calibration of hydrological model parameters plays an important role models’ performance. Identifying efficient reliable optimization algorithm objective function continues to be a significant challenge applying models. This study selected new algorithms, including strategic random search (SRS) sparrow (SSA) used hydrology, gold rush optimizer (GRO) snow ablation (SAO) not classical i.e., shuffling complex evolution (SCE-UA) particle swarm (PSO), calibrate two-parameter monthly balance (TWBM), abcd, HYMOD four functions Kling–Gupta efficiency (KGE) variant based on knowable moments (KMoments) considering high low flows (HiLo) simulation prediction Tunxi basin, China. Furthermore, identified scenario with best performance were applied change projections. The results show that abcd has performance, followed TWBM models, rank stability > functions, contributing years history period. KMoments KGE can play positive calibration, while effect adding HiLo unstable. SRS exhibits faster, more stable, than others calibration. obtained from optimal showed decrease compared reference period all SSP scenarios. In addition, distribution changed, maximum changing June May. Decreases simulated mainly occurred February July (10.9–56.1%). These findings may helpful determination parameter strategies, thus improving accuracy modeling prediction.
Language: Английский
Citations
3Journal of Environmental Management, Journal Year: 2024, Volume and Issue: 373, P. 123570 - 123570
Published: Dec. 6, 2024
Language: Английский
Citations
1