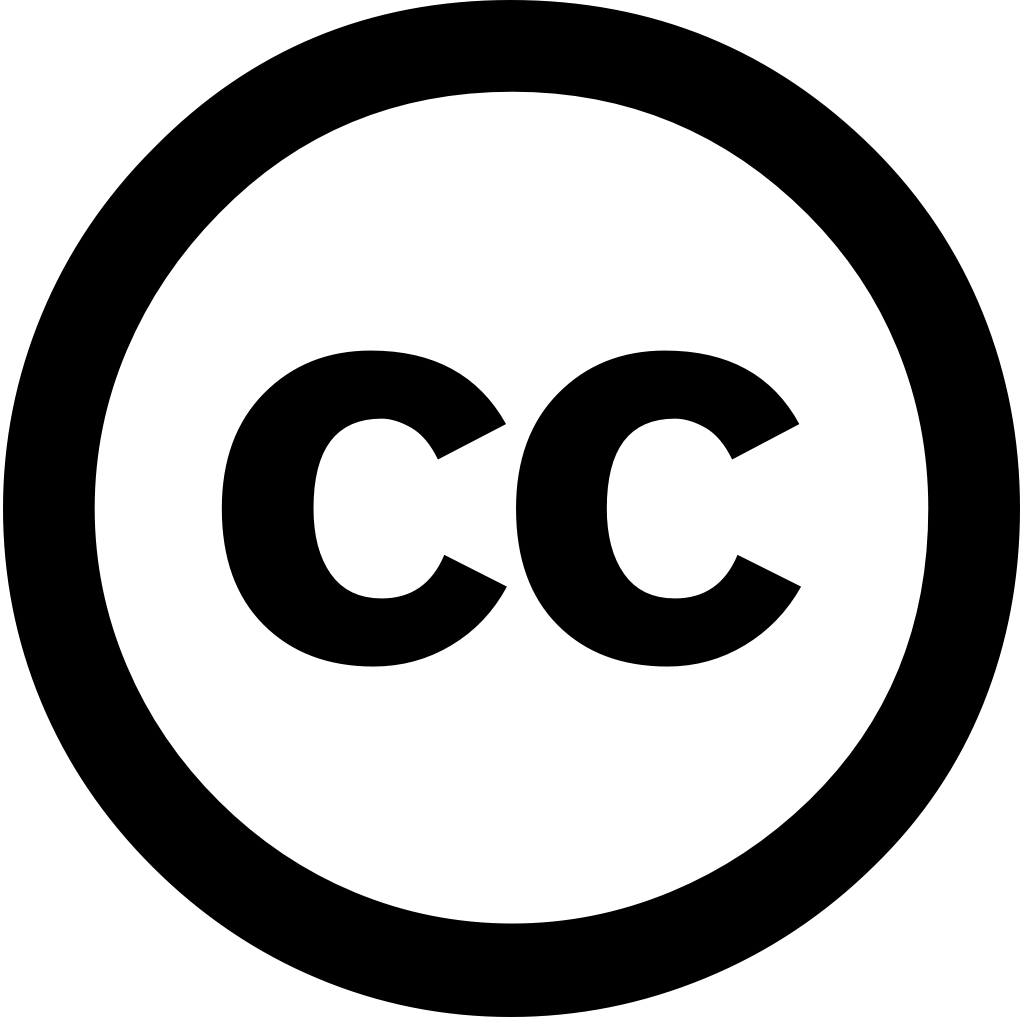
Plants, Journal Year: 2025, Volume and Issue: 14(8), P. 1226 - 1226
Published: April 16, 2025
In crop growth, irrigation has to be adjusted according developmental stages. Smart agriculture requires the accurate prediction of growth status and timely intervention improve quality agricultural products, but this task faces significant challenges due variable environmental factors. To address issue, study proposes a data-driven method enhance yield. Our approach harvests extensive datasets train optimize an integrated deep-learning architecture combining Informer, Long Short-Term Memory (LSTM) networks, Exponential Weighted Moving Average (EWMA) models. Controlled greenhouse experiments validated reliability practicality proposed strategy. The results showed that model accurately issued warnings 3–5 days in advance. Compared traditional fixed irrigation, significantly reduced frequency while maintaining same or even better conditions. terms plant quantity, experimental group increased by 410.0%, control grew 50.0%. Additionally, group’s average height was 21.8% higher than group. These demonstrate efficacy enhancing yield, providing novel strategy for future planning management.
Language: Английский