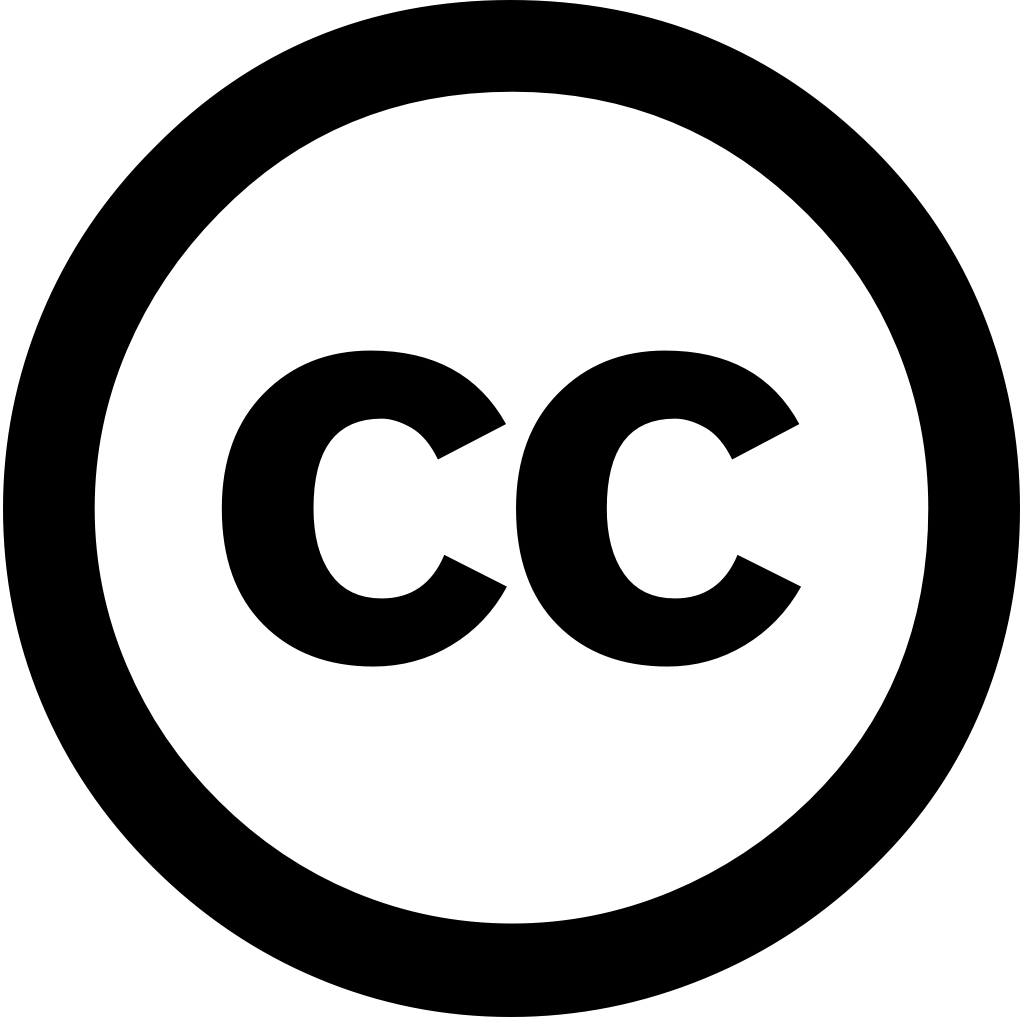
Mathematics, Journal Year: 2025, Volume and Issue: 13(7), P. 1083 - 1083
Published: March 26, 2025
Open-source disaster intelligence (OSDI) is crucial for improving situational awareness, preparedness, and real-time decision-making. Traditional OSDI frameworks often rely on social media data, which are susceptible to misinformation credibility issues. This study proposes a novel AI-driven framework utilizing automated data collection from 444 large-scale online news portals, including CNN, BBC, CBS News, The Guardian, enhance reliability. Over 514-day period (27 September 2023 26 February 2025), 1.25 million articles were collected, of 17,884 autonomously classified as disaster-related using Generative Pre-Trained Transformer (GPT) models. analysis identified 185 distinct countries 6068 unique locations, offering unprecedented geospatial temporal intelligence. Advanced clustering predictive analytics techniques, K-means, DBSCAN, seasonal decomposition (STL), Fourier transform, ARIMA, employed detect geographical hotspots, cyclical patterns, dependencies. ARIMA (2, 1, 2) model achieved mean squared error (MSE) 823,761, demonstrating high accuracy. Key findings highlight that the USA (6548 disasters), India (1393 Australia (1260 disasters) most disaster-prone countries, while hurricanes/typhoons/cyclones (5227 occurrences), floods (3360 wildfires (2724 occurrences) frequent types. establishes comprehensive methodology integrating clustering, analysis, multimodal processing in OSDI. By leveraging AI automation diverse sources, this provides scalable, adaptable, ethically robust solution proactive management, global resilience preparedness.
Language: Английский