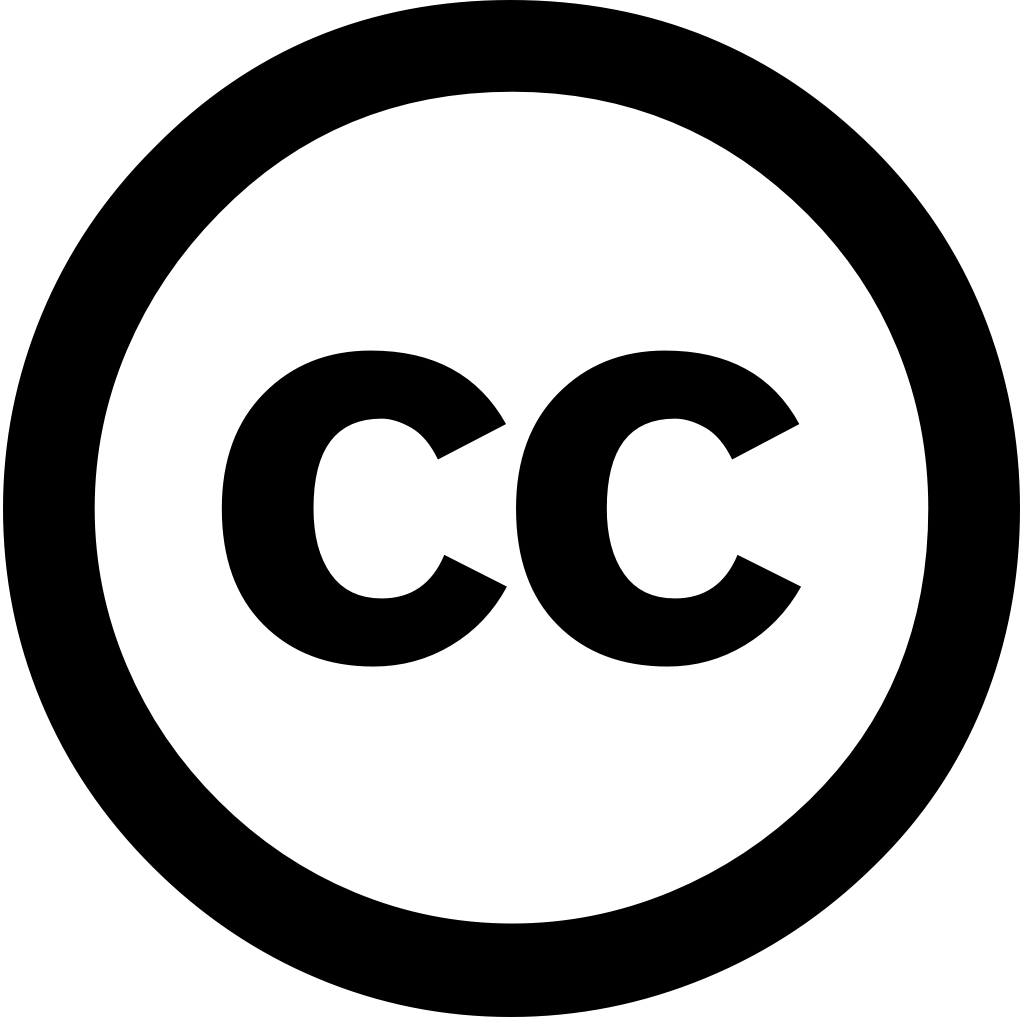
Applied Energy, Journal Year: 2022, Volume and Issue: 328, P. 120113 - 120113
Published: Nov. 1, 2022
Language: Английский
Applied Energy, Journal Year: 2022, Volume and Issue: 328, P. 120113 - 120113
Published: Nov. 1, 2022
Language: Английский
Sensors, Journal Year: 2023, Volume and Issue: 23(11), P. 5206 - 5206
Published: May 30, 2023
As the global population grows, and urbanization becomes more prevalent, cities often struggle to provide convenient, secure, sustainable lifestyles due lack of necessary smart technologies. Fortunately, Internet Things (IoT) has emerged as a solution this challenge by connecting physical objects using electronics, sensors, software, communication networks. This transformed city infrastructures, introducing various technologies that enhance sustainability, productivity, comfort for urban dwellers. By leveraging Artificial Intelligence (AI) analyze vast amount IoT data available, new opportunities are emerging design manage futuristic cities. In review article, we an overview cities, defining their characteristics exploring architecture IoT. A detailed analysis wireless employed in applications is presented, with extensive research conducted determine most appropriate specific use cases. The article also sheds light on different AI algorithms suitability applications. Furthermore, integration scenarios discussed, emphasizing potential contributions 5G networks coupled advancing modern environments. contributes existing literature highlighting tremendous presented integrating AI, paving way development significantly quality life dwellers while promoting sustainability productivity. IoT, integration, provides valuable insights into future demonstrating how these can positively impact environments well-being inhabitants.
Language: Английский
Citations
234IEEE Transactions on Smart Grid, Journal Year: 2022, Volume and Issue: 13(4), P. 2935 - 2958
Published: Feb. 25, 2022
With large-scale integration of renewable generation and distributed energy resources, modern power systems are confronted with new operational challenges, such as growing complexity, increasing uncertainty, aggravating volatility. Meanwhile, more data becoming available owing to the widespread deployment smart meters, sensors, upgraded communication networks. As a result, data-driven control techniques, especially reinforcement learning (RL), have attracted surging attention in recent years. This paper provides comprehensive review various RL techniques how they can be applied decision-making systems. In particular, we select three key applications, i.e., frequency regulation, voltage control, management, examples illustrate RL-based models solutions. We then present critical issues application RL, safety, robustness, scalability, data. Several potential future directions discussed well.
Language: Английский
Citations
210Journal of Modern Power Systems and Clean Energy, Journal Year: 2022, Volume and Issue: 10(2), P. 345 - 360
Published: Jan. 1, 2022
Deep neural networks have revolutionized many machine learning tasks in power systems, ranging from pattern recognition to signal processing. The data these are typically represented Euclidean domains. Nevertheless, there is an increasing number of applications where collected non-Euclidean domains and as graph-structured with high-dimensional features interdependency among nodes. complexity has brought significant challenges the existing deep defined Recently, publications generalizing for systems emerged. In this paper, a comprehensive overview graph (GNNs) proposed. Specifically, several classical paradigms GNN structures, e. g., convolutional networks, summarized. Key such fault scenario application, time-series prediction, flow calculation, generation reviewed detail. Further-more, main issues some research trends about GNNs discussed.
Language: Английский
Citations
197International Journal of Production Research, Journal Year: 2021, Volume and Issue: 60(13), P. 4316 - 4341
Published: Sept. 17, 2021
Shortening product development cycles and fully customisable products pose major challenges for production systems. These not only have to cope with an increased diversity but also enable high throughputs provide a adaptability robustness process variations unforeseen incidents. To overcome these challenges, deep Reinforcement Learning (RL) has been increasingly applied the optimisation of Unlike other machine learning methods, RL operates on recently collected sensor-data in direct interaction its environment enables real-time responses system changes. Although is already being deployed systems, systematic review results yet established. The main contribution this paper researchers practitioners overview applications motivate further implementations research supported Findings reveal that variety domains, contributing data-driven flexible processes. In most applications, conventional methods were outperformed implementation efforts or dependence human experience reduced. Nevertheless, future must focus more transferring findings real-world systems analyse safety aspects demonstrate reliability under prevailing conditions.
Language: Английский
Citations
150IEEE Access, Journal Year: 2021, Volume and Issue: 9, P. 54558 - 54578
Published: Jan. 1, 2021
The current electric power system witnesses a significant transition into Smart Grids (SG) as promising landscape for high grid reliability and efficient energy management. This ongoing undergoes rapid changes, requiring plethora of advanced methodologies to process the big data generated by various units. In this context, SG stands tied very closely Deep Learning (DL) an emerging technology creating more decentralized intelligent paradigm while integrating intelligence in supervisory operational decision-making. Motivated outstanding success DL-based prediction methods, article attempts provide thorough review from broad perspective on state-of-the-art advances DL systems. Firstly, bibliometric analysis has been conducted categorize review's methodology. Further, we taxonomically delve mechanism behind some trending algorithms. We then showcase enabling technologies SG, such federated learning, edge intelligence, distributed computing. Finally, challenges research frontiers are provided serve guidelines future work futuristic domain. study's core objective is foster synergy between these two fields decision-makers researchers accelerate DL's practical deployment
Language: Английский
Citations
133Journal of Modern Power Systems and Clean Energy, Journal Year: 2022, Volume and Issue: 10(3), P. 562 - 576
Published: Jan. 1, 2022
Security-constrained unit commitment (SCUC) has been extensively studied as a key decision-making tool to determine optimal power generation schedules in the operation of electricity market. With development emerging grids, fruitful research results on SCUC have obtained. Therefore, it is essential review current work and propose future directions for meet needs developing systems. In this paper, basic mathematical model standard summarized, characteristics application scopes common solution algorithms are presented. Customized models focusing diverse properties then categorized corresponding solving methodologies discussed. Finally, trends field prospected based summary state-of-the-art latest studies. It hoped that paper can be useful reference support theoretical practical applications future.
Language: Английский
Citations
106Reliability Engineering & System Safety, Journal Year: 2023, Volume and Issue: 234, P. 109204 - 109204
Published: Feb. 27, 2023
This work reviews maintenance optimization from different and complementary points of view. Specifically, we systematically analyze the knowledge, information data that can be exploited for within Industry 4.0 paradigm. Then, possible objectives are critically discussed, together with features to optimized, such as periods degradation thresholds. The main challenges trends are, then, highlighted need is identified methods do not require a-priori selection a predefined strategy, able deal large amounts heterogeneous collected sources, properly treat all uncertainties affecting behavior systems environment, jointly consider multiple objectives, including emerging ones related sustainability resilience.
Language: Английский
Citations
94Journal of Modern Power Systems and Clean Energy, Journal Year: 2022, Volume and Issue: 10(3), P. 719 - 730
Published: Jan. 1, 2022
A time-variable time-of-use electricity price can be used to reduce the charging costs for electric vehicle (EV) owners. Considering uncertainty of fluctuation and randomness EV owner's commuting behavior, we propose a deep reinforcement learning based method minimization individual cost. The problem is first formulated as Markov decision process (MDP), which has unknown transition probability. modified long short-term memory (LSTM) neural network representation layer extract temporal features from signal. deterministic policy gradient (DDPG) algorithm, continuous action spaces, solve MDP. proposed automatically adjust strategy according cost owner. Several other methods are also implemented quantitatively compared with up 70.2% benchmark methods.
Language: Английский
Citations
89Sustainability, Journal Year: 2023, Volume and Issue: 15(9), P. 7087 - 7087
Published: April 23, 2023
This article presents a review of current advances and prospects in the field forecasting renewable energy generation using machine learning (ML) deep (DL) techniques. With increasing penetration sources (RES) into electricity grid, accurate their becomes crucial for efficient grid operation management. Traditional methods have limitations, thus ML DL algorithms gained popularity due to ability learn complex relationships from data provide predictions. paper reviews different approaches models that been used discusses strengths limitations. It also highlights challenges future research directions field, such as dealing with uncertainty variability generation, availability, model interpretability. Finally, this emphasizes importance developing robust enable integration RES facilitate transition towards sustainable future.
Language: Английский
Citations
87IEEE Transactions on Industrial Informatics, Journal Year: 2022, Volume and Issue: 19(3), P. 2552 - 2564
Published: April 5, 2022
Effective detection of fault in rolling bearings with a limited amount data is essential for the safe operation electric machines. This article proposes novel meta-learning-enabled method machines under varying working conditions data. The diagnosis various cast as few-shot classification problem, which solved using model-agnostic meta-learning-based model. Specifically, meta-learner first trained series interrelated fault-diagnosis tasks conditions. During this stage, gradient-by-gradient rule utilized parameter optimization to achieve an effective representation these tasks. Then, parameters are refined on new task. technique can fast adaptation by utilizing only samples. proposed obtain high fault-detection accuracy when available. Comparative tests among methods were carried out Case Western Reserve University Bearing Dataset and Paderborn Rolling Dataset. results show that model performs better than other state-of-the-art conditions; our has stronger generalization ability faster ability. both datasets was at least 99%, proves strategy be flexibly applied scenarios.
Language: Английский
Citations
75