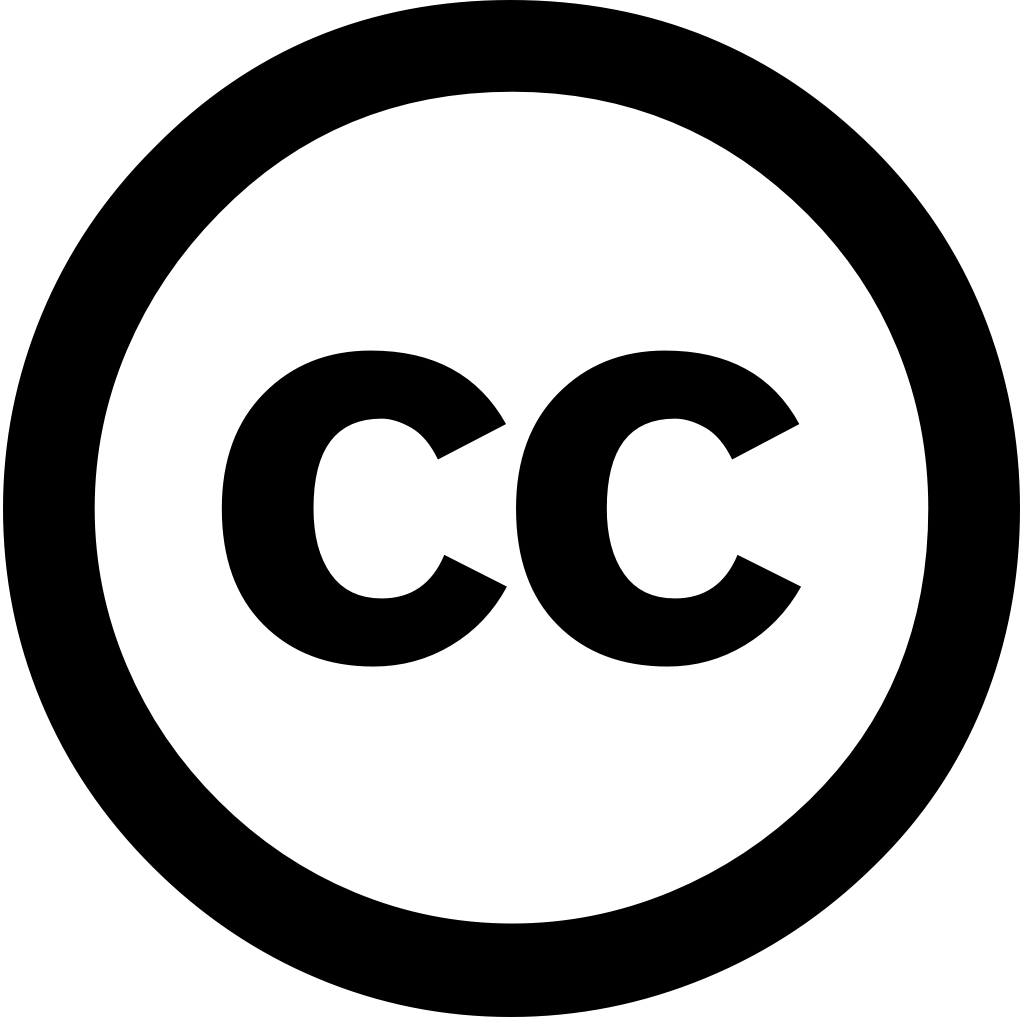
Trees Forests and People, Journal Year: 2024, Volume and Issue: 18, P. 100727 - 100727
Published: Nov. 16, 2024
Language: Английский
Trees Forests and People, Journal Year: 2024, Volume and Issue: 18, P. 100727 - 100727
Published: Nov. 16, 2024
Language: Английский
Ecography, Journal Year: 2025, Volume and Issue: unknown
Published: March 19, 2025
Advances in computational power and methods, the widespread availability of remote sensing data have driven development machine learning models for estimating global carbon storage. Current often rely on dozens predictor variables to estimate aboveground biomass density (AGBD), resulting accurate but complex that are challenging interpret from a biological ecological standpoint. Yet, it remains unclear whether such model complexity is essential achieving predictions. This manuscript investigates potential create simpler, yet accurate, AGBD model. Our approach leverages only climate‐based predictors, using systematic selection process determine optimal subset maximize accuracy. Surprisingly, we found minimal trained with four bioclimatic outperformed more models. When compared state‐of‐the‐art ground‐based data, our achieved comparable accuracy far fewer than 186 predictors used In conclusion, present lightweight, interpretable estimation, additional advantage being adaptable projecting under future climate scenarios.
Language: Английский
Citations
0PLOS Climate, Journal Year: 2025, Volume and Issue: 4(3), P. e0000584 - e0000584
Published: March 26, 2025
Citations
0Trees Forests and People, Journal Year: 2024, Volume and Issue: 18, P. 100727 - 100727
Published: Nov. 16, 2024
Language: Английский
Citations
0