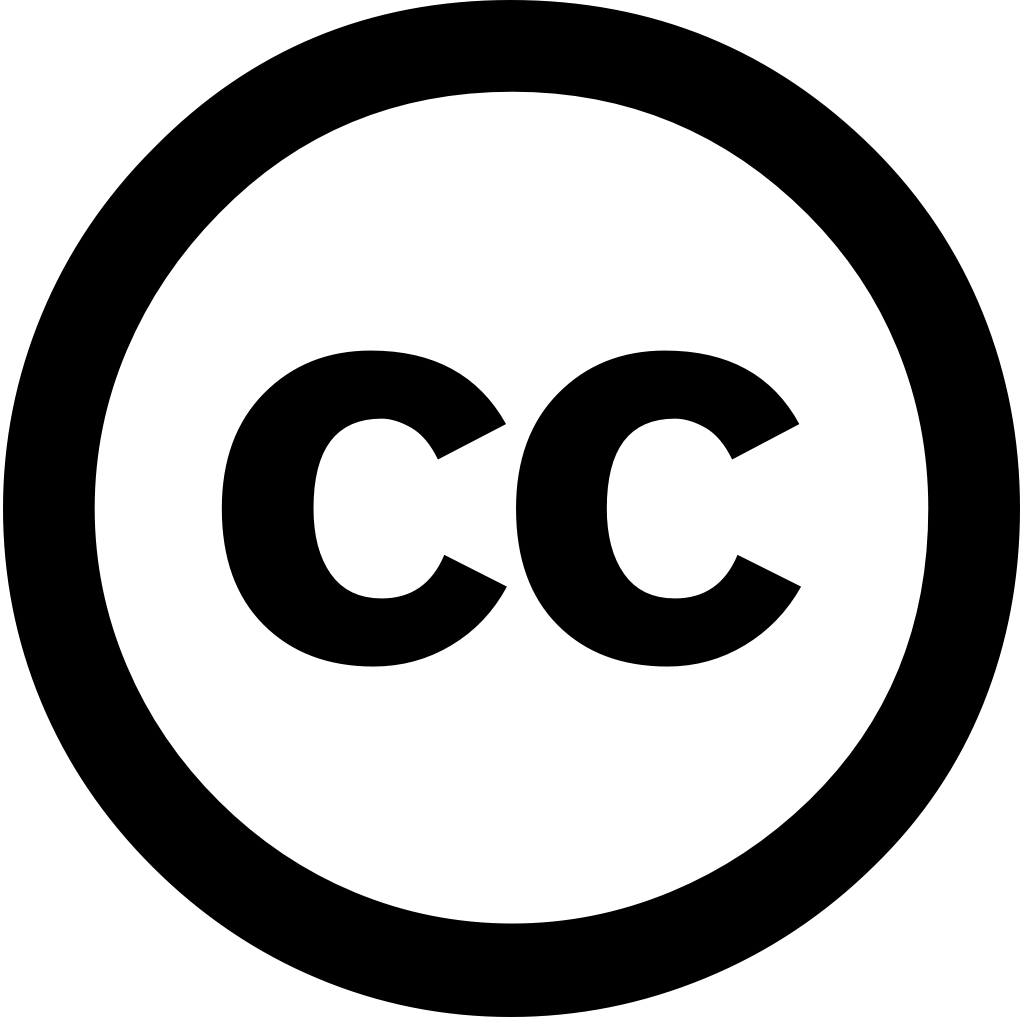
Applied Computational Intelligence and Soft Computing, Journal Year: 2025, Volume and Issue: 2025(1)
Published: Jan. 1, 2025
Hospital readmissions impose a significant financial strain on healthcare systems and can adversely affect patients. Unfortunately, traditional approaches to predicting frequently lack accuracy. This presents critical challenge, as identifying patients at high risk for readmission is essential implementing preventive measures. The study introduces novel method that employs machine learning automatically extract features from patient data, eliminating labor‐intensive manual feature engineering. primary goal develop predictive models unplanned UTI Jordan University within 3 months postdischarge. executed through retrospective analysis of electronic health records January 2020 June 2023. By leveraging techniques, the identifies high‐risk by evaluating demographic, clinical, outcome characteristics, ensuring model reliability thorough optimization, validation, performance assessment. Three were developed follows: gradient‐boosting classifier (GBC), logistic regression (LR), stochastic gradient descent (SGD). GBC, SGD, LR achieved impressive accuracy rates 99%, 95%, 89%, providing strong confidence in methodology. study’s findings reveal key factors associated with readmissions, enhancing our understanding this process offering valuable framework improving care, optimizing resource allocation, supporting evidence‐based decision‐making management.
Language: Английский