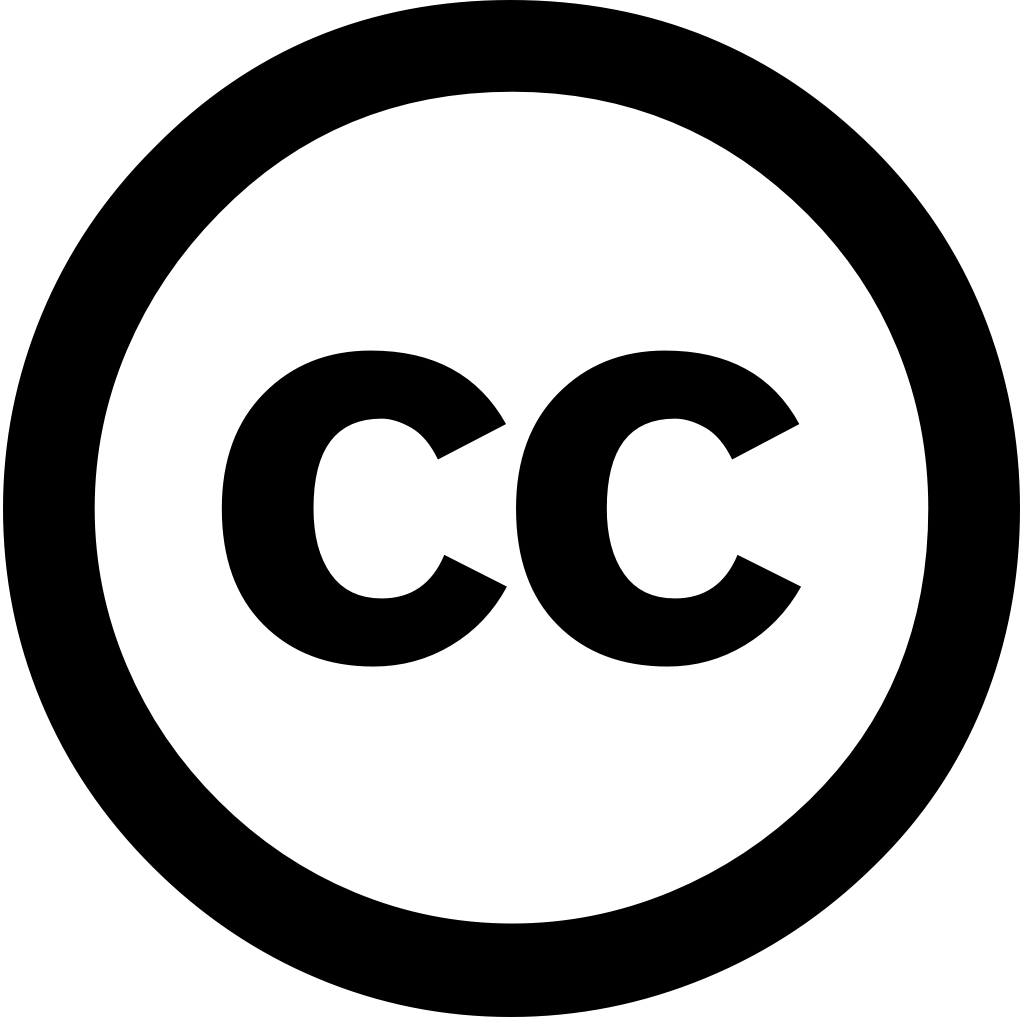
Deleted Journal, Journal Year: 2025, Volume and Issue: 7(5)
Published: April 20, 2025
Language: Английский
Deleted Journal, Journal Year: 2025, Volume and Issue: 7(5)
Published: April 20, 2025
Language: Английский
Scientific Reports, Journal Year: 2025, Volume and Issue: 15(1)
Published: Feb. 14, 2025
The classification of remote sensing images is inherently challenging due to the complexity, diversity, and sparsity data across different image samples. Existing advanced methods often require substantial modifications model architectures achieve optimal performance, resulting in complex frameworks that are difficult adapt. To overcome these limitations, we propose a lightweight ensemble method, enhanced by pure correction, called Exceptionally Straightforward Ensemble. This approach eliminates need for extensive structural models. A key innovation our method introduction novel strategy, quantitative augmentation, implemented through plug-and-play module. strategy effectively corrects feature distributions data, significantly improving performance Convolutional Neural Networks Vision Transformers beyond traditional augmentation techniques. Furthermore, straightforward algorithm generate an network composed two components, serving as proposed classifier. We evaluate on three well-known datasets, with results demonstrating models outperform 48 state-of-the-art published since 2020, excelling accuracy, inference speed, compactness. Specifically, overall accuracy up 96.8%, representing 1.1% improvement NWPU45 dataset. Moreover, smallest reduces parameters 90% time 74%. Notably, enhances Transformers, even limited training thus alleviating dependence large-scale datasets. In summary, data-driven offers efficient, accessible solution classification, providing elegant alternative researchers geoscience fields who may have or resources optimization.
Language: Английский
Citations
3International Journal of Intelligent Computing and Cybernetics, Journal Year: 2024, Volume and Issue: 18(1), P. 133 - 152
Published: Nov. 13, 2024
Purpose Vision transformers (ViT) detectors excel in processing natural images. However, when remote sensing images (RSIs), ViT methods generally exhibit inferior accuracy compared to approaches based on convolutional neural networks (CNNs). Recently, researchers have proposed various structural optimization strategies enhance the performance of detectors, but progress has been insignificant. We contend that frequent scarcity RSI samples is primary cause this problem, and model modifications alone cannot solve it. Design/methodology/approach To address this, we introduce a faster RCNN-based approach, termed QAGA-Net, which significantly enhances recognition. Initially, propose novel quantitative augmentation learning (QAL) strategy sparse data distribution RSIs. This integrated as QAL module, plug-and-play component active exclusively during model’s training phase. Subsequently, enhanced feature pyramid network (FPN) by introducing two efficient modules: global attention (GA) module long-range dependencies multi-scale information fusion, an pooling (EP) optimize capability understand both high low frequency information. Importantly, QAGA-Net compact size achieves balance between computational efficiency accuracy. Findings verified using different models detector’s backbone. Extensive experiments NWPU-10 DIOR20 datasets demonstrate superior 23 other or CNN literature. Specifically, shows increase mAP 2.1% 2.6% challenging dataset top-ranked respectively. Originality/value paper highlights impact detection performance. fundamentally data-driven approach: module. Additionally, introduced modules FPN. More importantly, our potential collaborate with method does not require any
Language: Английский
Citations
10Annals of Emerging Technologies in Computing, Journal Year: 2024, Volume and Issue: 8(4), P. 56 - 76
Published: Oct. 1, 2024
Vision Transformers (ViTs) have demonstrated exceptional accuracy in classifying remote sensing images (RSIs). However, existing knowledge distillation (KD) methods for transferring representations from a large ViT to more compact Convolutional Neural Network (CNN) proven ineffective. This limitation significantly hampers the remarkable generalization capability of ViTs during deployment due their substantial size. Contrary common beliefs, we argue that domain discrepancies along with RSI inherent natures constrain effectiveness and efficiency cross-modal transfer. Consequently, propose novel Variance Consistency Learning (VCL) strategy enhance KD process, implemented through plug-and-plug module within ViTteachingCNN pipeline. We evaluated our student model, termed VCL-Net, on three datasets. The results reveal VCL-Net exhibits superior size compared 33 other state-of-the-art published past years. Specifically, surpasses KD-based maximum improvement 22% across different Furthermore, visualization analysis model activations reveals has learned long-range dependencies features teacher. Moreover, ablation experiments suggest method reduced time costs process by at least 75%. Therefore, study offers effective efficient approach transfer when addressing discrepancies.
Language: Английский
Citations
6Information Fusion, Journal Year: 2024, Volume and Issue: unknown, P. 102742 - 102742
Published: Oct. 1, 2024
Language: Английский
Citations
4Deleted Journal, Journal Year: 2025, Volume and Issue: 7(5)
Published: April 20, 2025
Language: Английский
Citations
0