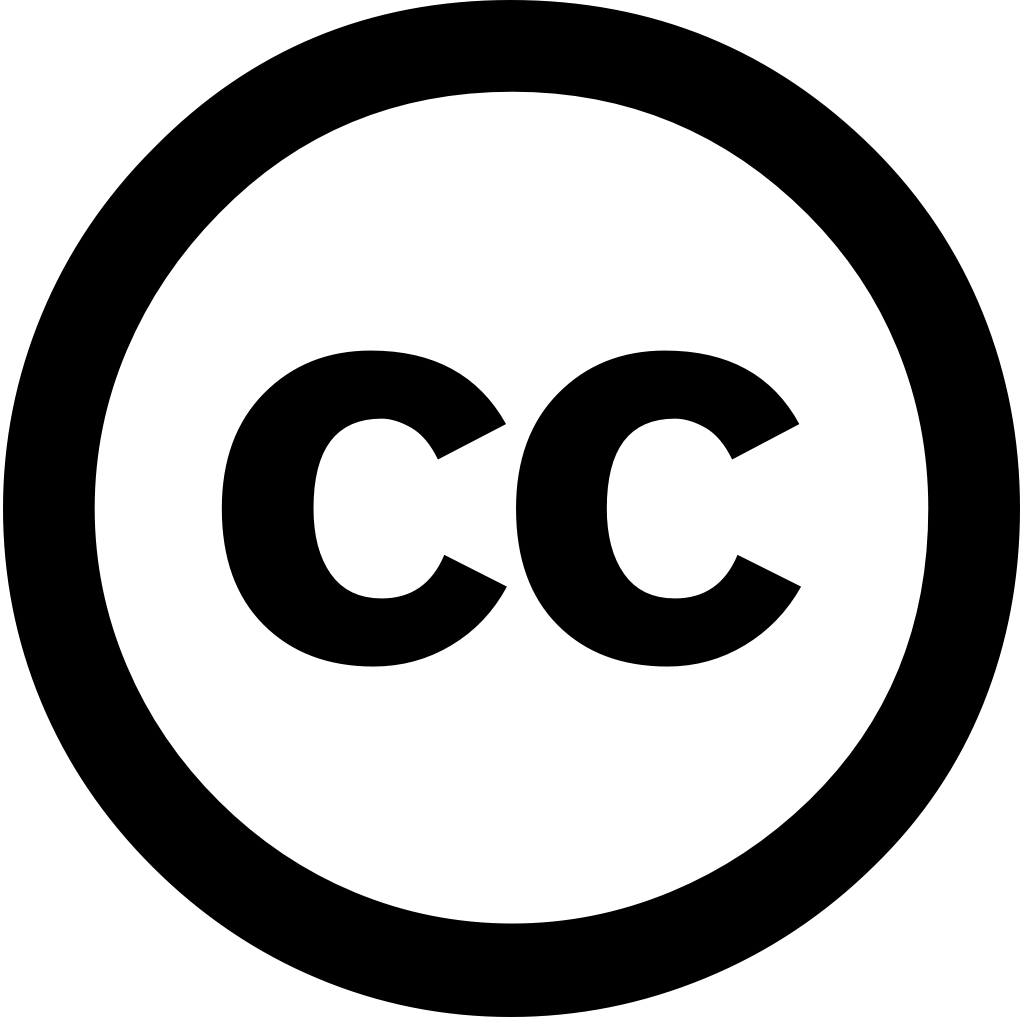
International Journal of Current Innovations in Advanced Research, Journal Year: 2024, Volume and Issue: unknown, P. 14 - 22
Published: April 11, 2024
Prediction models play a crucial role in early detection and intervention for cardiac diseases. However, their effectiveness is often hindered by limitations inherent current methodologies. This paper proposes novel approach to address these challenges integrating Independent Component Analysis (ICA) with the Support Vector Machine (SVM) technique. Utilizing comprehensive Cleveland dataset, our model achieves notable performance metrics, including an accuracy of 90.16%, Area Under Curve (AUC) 96.66%, precision 90.02%, recall 90.00%, F1-score minimal log loss 3.54. Our methodology not only surpasses previous methodologies through extensive comparative analysis but also addresses common constraints identified existing literature. These encompass insufficient feature representation, overfitting, lack proactive strategies. By amalgamating ICA SVM, enhances extraction, mitigates facilitates diagnosis individuals suspected having heart disease. study underscores importance mitigating literature potential contemporary machine-learning techniques advance prediction
Language: Английский