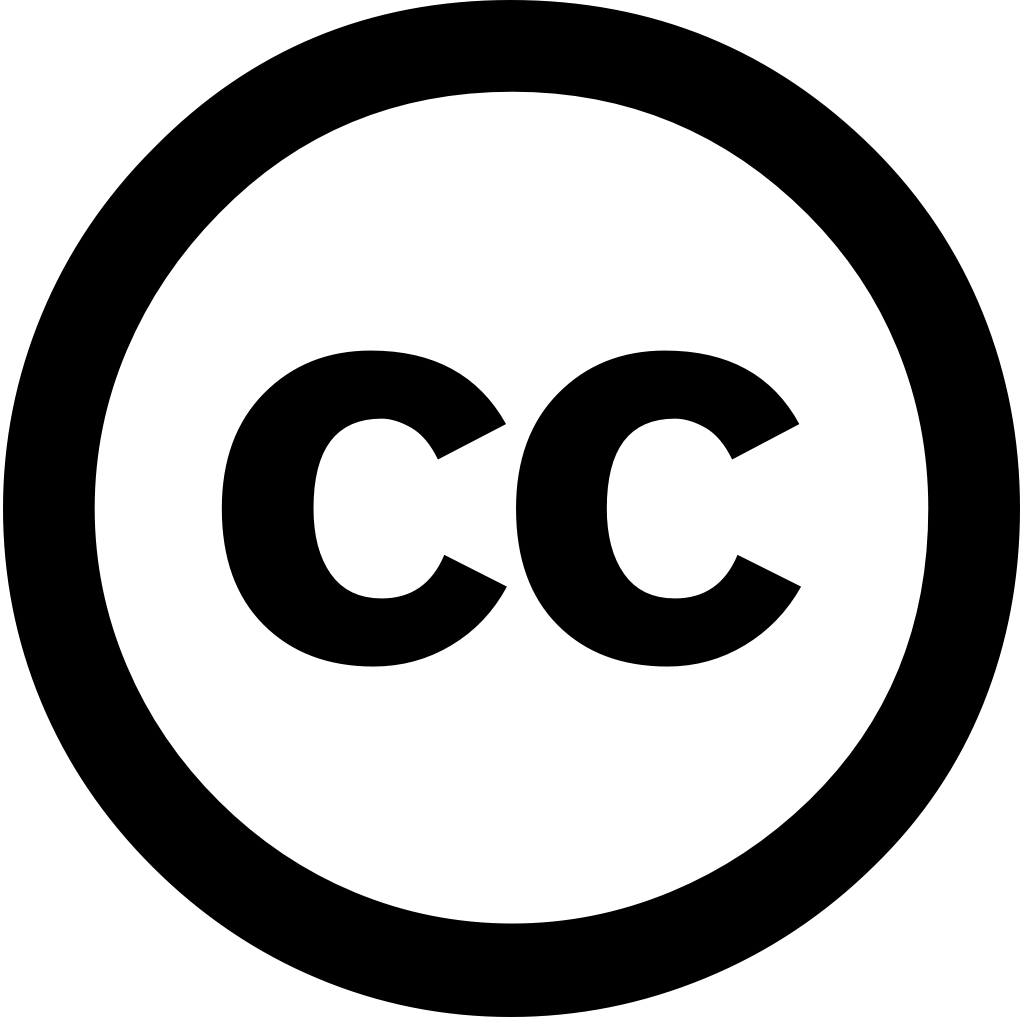
Solar Energy and Sustainable Development, Journal Year: 2025, Volume and Issue: 14(1), P. 361 - 378
Published: April 28, 2025
With the increasing adoption of solar photovoltaic (PV) systems, ensuring their reliability and efficiency is crucial for sustainable energy production. However, traditional fault detection methods rely on expensive manual inspections or sensor-based monitoring, often slow inefficient. This study aims to bridge this gap by leveraging machine learning techniques enhance maintenance optimization in PV systems. We evaluate five advanced models—Random Forest, XGBoost, Artificial Neural Networks (ANN), Convolutional (CNN), Support Vector Machines (SVM)—using accurate operational data from a 250-kW power station. The dataset includes key parameters such as current, voltage, output, temperature, irradiance. Data preprocessing included outlier removal, feature selection via Pearson correlation, normalization improve model performance. models were trained tested using an 80-20 split evaluated based classification accuracy, precision, recall, F1-score. Our results show that XGBoost achieved highest accuracy (88%), making it best candidate real-time predictive maintenance. Random Forest also performed well (87% accuracy), particularly handling noisy data. ANN CNN effectively detected long-term degradation patterns, supporting preventive strategies. Based these findings, we propose dual strategy: detection, while monitor gradual system deterioration. research provides practical framework integrating into management, offering scalable solution reliability, reduce costs, optimize efficiency.
Language: Английский