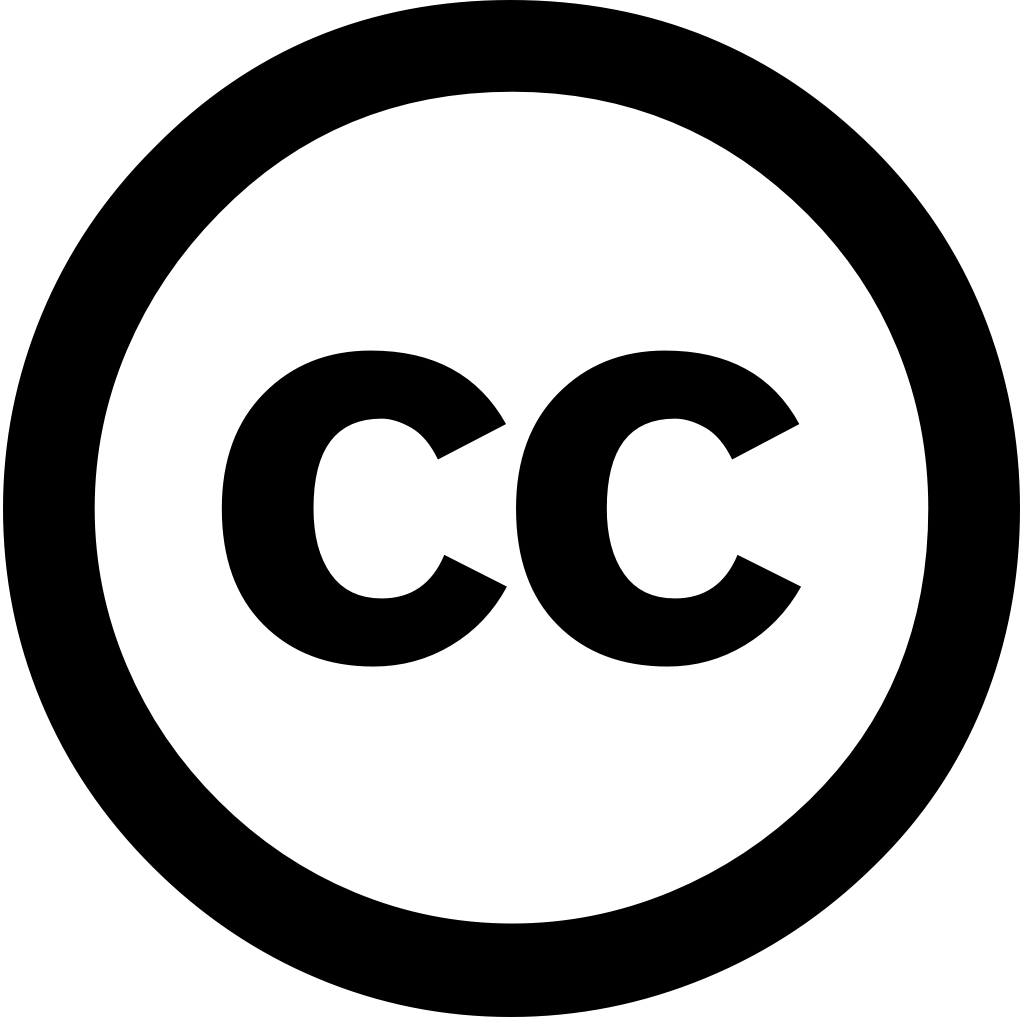
Journal of Hydrology Regional Studies, Journal Year: 2024, Volume and Issue: 56, P. 102031 - 102031
Published: Nov. 4, 2024
Language: Английский
Journal of Hydrology Regional Studies, Journal Year: 2024, Volume and Issue: 56, P. 102031 - 102031
Published: Nov. 4, 2024
Language: Английский
Earth system science data, Journal Year: 2024, Volume and Issue: 16(12), P. 5625 - 5642
Published: Dec. 9, 2024
Abstract. Comprehensive large-sample hydrological datasets, particularly the CAMELS datasets (Catchment Attributes and MEteorology for Large-sample Studies), have advanced research education in recent years. These integrate extensive hydro-meteorological observations with landscape features, such as geology land use, across numerous catchments within a national framework. They provide harmonised data various purposes, assessing impacts of climate change or testing models on large number catchments. Furthermore, these are essential rapid progress data-driven hydrology Despite Germany's measurement infrastructure, it has lacked consistent, nationwide dataset, largely due to its decentralised management different federal states. This fragmentation hindered cross-state studies made preparation labour-intensive. The introduction CAMELS-DE represents step forward bridging this gap. includes 1582 streamflow gauges time series covering up 70 years (median length 46 minimum 10 years), from January 1951 December 2020. It consistent catchment boundaries areas ranging 5 15 000 km2 along detailed attributes soil, cover, hydrogeologic properties, human influences. regionally trained long short-term memory (LSTM) network locally HBV (Hydrologiska Byråns Vattenbalansavdelning) model that were used quality control can be fill gaps discharge act baseline development new models. Given catchments, including relatively small ones (636 < 100 km2), (166 data), is one most comprehensive available offers opportunities research, studying long-term trends runoff formation analysing strong article describes version 1.0, which at https://doi.org/10.5281/zenodo.13837553 (Dolich et al., 2024).
Language: Английский
Citations
5Journal of Hydrology, Journal Year: 2025, Volume and Issue: unknown, P. 132674 - 132674
Published: Jan. 1, 2025
Language: Английский
Citations
0Environmental Modelling & Software, Journal Year: 2025, Volume and Issue: unknown, P. 106350 - 106350
Published: Jan. 1, 2025
Language: Английский
Citations
0Hydrology and earth system sciences, Journal Year: 2025, Volume and Issue: 29(4), P. 841 - 861
Published: Feb. 18, 2025
Abstract. In this study, we use deep learning models with advanced variants of recurrent neural networks, specifically long short-term memory (LSTM), gated unit (GRU), and bidirectional LSTM (BiLSTM), to simulate large-scale groundwater level (GWL) fluctuations in northern France. We develop multi-station collective training for GWL simulations, using dynamic variables (i.e. climatic) static basin characteristics. This approach can incorporate features cover more reservoir heterogeneities the study area. Further, investigated performance relevant feature extraction techniques such as clustering wavelet transform decomposition simplify network regionalised information. Several modelling tests were conducted. Models trained on different types GWL, clustered based spectral properties, performed significantly better than whole dataset. Clustering-based reduces complexity data targets information efficiently. Applying without prior lead preferentially learn dominant behaviour, ignoring unique local variations. respect, pre-processing was found partially compensate clustering, bringing out common temporal characteristics shared by all available time series even when these are “hidden” (e.g. if their amplitude is too small). When employed along a technique improves model performances, particularly GWLs dominated low-frequency interannual decadal advances our understanding simulation learning, highlighting importance approaches, potential pre-processing, value incorporating attributes.
Language: Английский
Citations
0Earth system science data, Journal Year: 2025, Volume and Issue: 17(4), P. 1551 - 1572
Published: April 14, 2025
Abstract. Large samples of hydrometeorological time series and catchment attributes are critical for improving the understanding complex hydrological processes, model development, performance benchmarking. CAMELS (Catchment Attributes Meteorology Large-sample Studies) datasets have been developed in several countries regions around world, providing valuable data sources test beds analysis new frontiers data-driven modeling. Regarding lack from lowland, groundwater-dominated, small-sized catchments, we develop an extensive repository a CAMELS-style dataset Denmark (CAMELS-DK). This addition is first containing both gauged ungauged catchments as well detailed groundwater information. The provides dynamic static variables 3330 covering all various hydrogeological datasets, meteorological observations, well-established national-scale model. For 304 those streamflow observations provided, whereas simulated provided catchments. contains spanning 30 years (1989–2019) with daily step, will be updated once simulations become available. dense full spatial coverage instead only together simulation distributed, process-based model, enhances applicability such data, example, development hybrid physically informed modeling frameworks or other cases where consistent required. We also provide quantities related to human impact on system Denmark, abstraction irrigation. CAMELS-DK freely available at https://doi.org/10.22008/FK2/AZXSYP (Koch et al., 2024).
Language: Английский
Citations
0Scientific Data, Journal Year: 2025, Volume and Issue: 12(1)
Published: April 18, 2025
Abstract Human-induced warming is modifying the water cycle. Adaptation to posed threats requires an understanding of hydrological responses climate variability. Whilst these can be computationally modelled, observed streamflow data essential for constraining models, and quantifying emerging trends in To date, identification such at global scale has been hindered by limitations – particular, prevalence direct human influences on which obscure climate-driven By removing influences, more confidently attributed Here we describe Reference Observatory Basins INternational change detection (ROBIN) first iteration a network from national reference networks (RHNs) comprised catchments are near-natural or have limited influences. This collaboration established freely available RHN dataset over 3,000 code libraries, used underpin new science endeavours advance studies support international policy adaptation.
Language: Английский
Citations
0Journal of Hydrology Regional Studies, Journal Year: 2024, Volume and Issue: 56, P. 102031 - 102031
Published: Nov. 4, 2024
Language: Английский
Citations
0